A semantic decoder, a brand-new artificial intelligence system, is capable of transforming a person’s brain activity—whether they are silently imagining telling a story or listening to a story—into a continuous stream of text. The framework created by analysts at The College of Texas at Austin could assist people who are intellectually cognizant yet incapable of truly talking, for example, those crippled by strokes, to impart clearly once more.
Jerry Tang, a doctoral student in computer science, and Alex Huth, an assistant professor of neuroscience and computer science at UT Austin, led the study, which was published in the journal Nature Neuroscience. The work depends to some degree on a transformer model, like the ones that power Open Computer-Based Intelligence’s ChatGPT and Google’s Troubadour.
This system does not require subjects to have surgical implants, making it noninvasive compared to other language decoding systems in development. Members likewise don’t have to use just words from a recommended list. After the decoder has been trained extensively and the individual has listened to hours of podcasts in the scanner, brain activity is measured using an fMRI scanner. Later, if the participant is willing to have their thoughts decoded, the machine can generate corresponding text solely from brain activity by listening to a new story or imagining telling a story.
“Compared to previous work, which has often consisted of single words or brief sentences, this is a significant advancement for a noninvasive technology. We are training the model to decode continuous language with complex thoughts over long periods of time.”
Alex Huth, an assistant professor of neuroscience and computer science at UT Austin.
“This is a real leap forward compared to what’s been done before, which is typically single words or short sentences,” Huth stated. “For a noninvasive method, this is a real leap forward.” With complicated concepts, we are getting the model to decode continuous language for extended periods of time.
The outcome is not a complete transcript. Instead, it was designed by researchers to, albeit imperfectly, summarize what is being said or thought. When the decoder has been trained to monitor a participant’s brain activity, about half of the time, the machine produces text that closely (and sometimes precisely) matches the original words’ intended meanings.
For instance, a participant in an experiment who was hearing a speaker say, “I don’t have my driver’s license yet,” had their thoughts translated as, “She has not even started to learn to drive yet.” “I didn’t know whether to scream, cry, or run away.” I was listening to All things considered, I said, ‘Let me be!'” “She started to scream and cry, and then she just said, ‘I told you to leave me alone.”” was decoded.
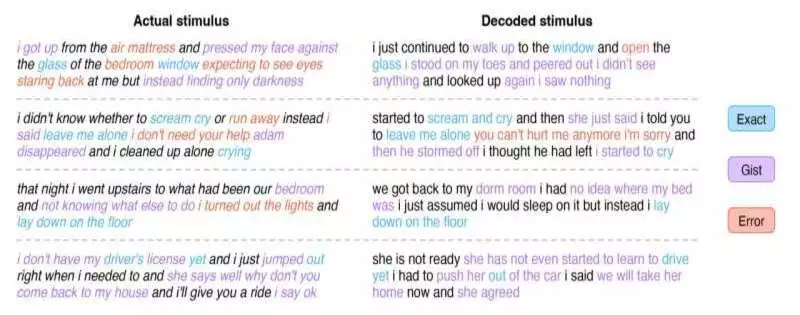
This picture shows decoder forecasts from cerebrum accounts gathered while a client paid attention to four stories. In order to demonstrate typical decoder behaviors, example segments were manually chosen and annotated. Some words and phrases are exactly reproduced by the decoder, and many others are captured in their essence. Credit: University of Texas at Austin
The researchers began by addressing concerns regarding the technology’s potential misuse in an earlier version of the paper that was published online as a preprint. The paper depicts how unraveling functioned exclusively with helpful members who had taken part readily in preparing the decoder. The results were unusable for people on whom the decoder had not been trained, and the results were the same if participants on whom the decoder had been trained later put up resistance, such as by thinking different thoughts.
“We treat exceptionally seriously the worries that it very well may be utilized for terrible purposes and have attempted to keep away from that,” Tang said. “We need to ensure individuals possibly utilize these kinds of advances when they need to and that it helps them.”
The researchers asked subjects to watch four brief, silent videos while they were in the scanner, in addition to listening to stories or thinking about them. The semantic decoder was able to accurately describe certain events from the videos by using their brain activity.
Due to its dependence on fMRI machine time, the system cannot be used outside of the laboratory at this time. However, the researchers believe that this work could be applied to functional near-infrared spectroscopy (fNIRS), a portable brain imaging technique.
“fNIRS measures where there’s something else or less blood stream in the cerebrum at various moments, which, it ends up, is the very same sort of sign that fMRI is estimating,” Huth said. “Consequently, our exact strategy ought to translate into fNIRS,” despite the fact that, as he pointed out, fNIRS would have a lower resolution.
The review’s other co-creators are Amanda LeBel, a previous exploration partner in the Huth lab, and Shailee Jain, a software engineering graduate understudy at UT Austin. Jerry Tang and Alexander Huth have submitted a PCT patent application for this work.
Frequently Asked Questions Could an authoritarian regime interrogating political prisoners or an employer spying on employees use this technology without their knowledge? No. The framework must be widely prepared on a willing subject in an office with enormous, costly gear. Loyer spying on employees use this technology without their knowledge? No. “The framework must be widely prepared on a willing subject in an office with enormous, costly gear.” “Huth stated that a person needs to spend up to 15 hours lying still in an MRI scanner and paying good attention to the stories they are listening to before this really works well on them.”
Is it possible to skip training entirely? No. The system’s results were incomprehensible when tested on untrained individuals by the researchers.
Are there ways somebody can guard against having their contemplations decoded? Yes. The specialists tried to determine whether an individual who had recently taken part in preparation could effectively oppose the resulting endeavors at mind translation. Participants were able to completely and easily prevent the system from recovering the speech they were exposed to by employing techniques such as thinking of animals or quietly imagining themselves telling their own story.
What if these defenses or obstacles were eventually overcome by technology and related research? Tang stated, “I think it’s important to be proactive by enacting policies that protect people and their privacy right now, while the technology is in such an early state.” Managing what these gadgets can be utilized for is additionally vital.”
More information: Semantic reconstruction of continuous language from non-invasive brain recordings, Nature Neuroscience (2023). DOI: 10.1038/s41593-023-01304-9