Using machine-learning algorithms, researchers created a method for swiftly calculating the transition state structure of a chemical reaction. During a chemical reaction, molecules accumulate energy until they reach a point known as the transition state, from which the reaction must continue. This state is so transient that observing it experimentally is practically impossible.
The structures of these transition states can be determined using quantum chemistry techniques, but the procedure is exceedingly time-consuming. A group of MIT researchers has now created an alternate approach based on machine learning that can calculate these structures far faster – in a matter of seconds.
Their new model might be used to assist scientists in developing new reactions and catalysts to produce useful products such as fuels or pharmaceuticals, or to represent naturally occurring chemical processes such as those that may have aided in the evolution of life on Earth.
“Knowing that transition state structure is really important as a starting point for thinking about designing catalysts or understanding how natural systems enact certain transformations,” says Heather Kulik, senior author of the study and an associate professor of chemistry and chemical engineering at MIT.
Chenru Duan PhD ’22 is the lead author of a paper describing the work, which appears today in Nature Computational Science. Cornell University graduate student Yuanqi Du and MIT graduate student Haojun Jia are also authors of the paper.
Knowing that transition state structure is really important as a starting point for thinking about designing catalysts or understanding how natural systems enact certain transformations.
Heather Kulik
Fleeting transitions
To occur, any particular chemical reaction must go through a transition state, which occurs when it achieves the energy threshold required for the reaction to proceed. The likelihood of any chemical reaction occurring is governed in part by the likelihood of the transition state forming.
“The transition state influences the likelihood of a chemical transformation occurring. If we have a lot of something we don’t want, such as carbon dioxide, and we want to convert it to a useful fuel, such as methanol, the transition state and how favorable it is influences how likely we are to move from the reactant to the product,” Kulik explains.
Chemists can calculate transition states using a quantum chemistry method known as density functional theory. However, this method requires a huge amount of computing power and can take many hours or even days to calculate just one transition state.
Recently, some researchers have tried to use machine-learning models to discover transition state structures. However, models developed so far require considering two reactants as a single entity in which the reactants maintain the same orientation with respect to each other. Any other possible orientations must be modeled as separate reactions, which adds to the computation time.
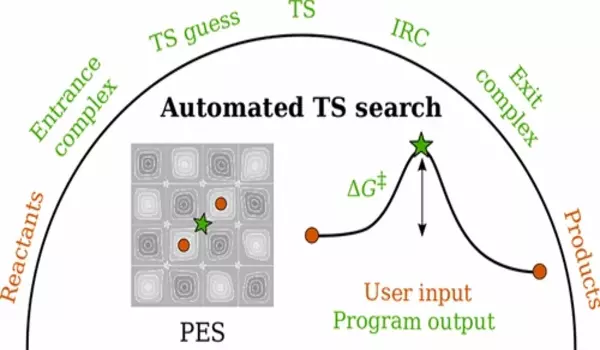
“If the reactant molecules are rotated, then in principle, before and after this rotation they can still undergo the same chemical reaction. But in the traditional machine-learning approach, the model will see these as two different reactions. That makes the machine-learning training much harder, as well as less accurate,” Duan says.
The MIT team created a new computational technique that allowed them to represent two reactants in any arbitrary orientation with regard to each other, using a diffusion model, which can learn which types of processes are more likely to produce a specific conclusion. The researchers employed structures of reactants, products, and transition states generated using quantum computation methods for 9,000 distinct chemical processes as training data for their model.
“Once the model learns the underlying distribution of how these three structures coexist, we can give it new reactants and products, and it will try to generate a transition state structure that pairs with those reactants and products,” Duan says.
The researchers put their model to the test on around 1,000 new reactions, asking it to provide 40 different solutions for each transition state. They then used a “confidence model” to estimate which states will occur most frequently. When compared to transition state structures constructed using quantum approaches, these answers were accurate to 0.08 angstroms (one hundred millionth of a centimeter). For each reaction, the complete computer procedure takes only a few seconds.
“You can imagine that really scales to thinking about generating thousands of transition states in the time that it would normally take you to generate just a handful with the conventional method,” Kulik said.
Modeling reactions
Although the researchers trained their model primarily on reactions involving compounds with a relatively small number of atoms — up to 23 atoms for the entire system — they found that it could also make accurate predictions for reactions involving larger molecules.
“Even if you look at bigger systems or systems catalyzed by enzymes, you’re getting pretty good coverage of the different types of ways that atoms are most likely to rearrange,” Kulik says.
The researchers intend to develop their model to include other components such as catalysts, which will allow them to explore how much a specific catalyst will speed up a reaction. This could be valuable for inventing new procedures for producing medications, fuels, or other useful substances, particularly when the synthesis requires a large number of chemical steps.
“Traditionally all of these calculations are performed with quantum chemistry, and now we’re able to replace the quantum chemistry part with this fast generative model,” Duan said.
According to the researchers, another potential application for this type of model is examining the interactions that might occur between gases present on other planets, or modeling the simple reactions that may have occurred during the early evolution of life on Earth.