Omnipose, a profound learning program, is assisting with tackling the test of recognizing changed and miniscule microbes in microscopy pictures. It has gone past this underlying objective to recognize a few different sorts of small items in micrographs.
The UW Medication microbial science lab of Joseph Mougous and the College of Washington physical science and bioengineering lab of Paul A. Wiggins tried the device. It was created by College of Washington material science graduate understudy Kevin J. Cutler and his group.
Mougous said that Cutler, as a material science understudy, “showed a strange interest in drenching himself in a scientific climate so he could learn directly about issues needing arrangement in this field. He approached my lab and immediately found one that he settled into with amazing style.”
Their outcomes are accounted for in the Oct. 17 release of Nature Strategies.
The researchers tracked down that Omnipose, prepared on a huge data set of bacterial pictures, performed well in describing and measuring the heap of microorganisms in blended microbial societies and killed a portion of the blunders that could happen in its ancestor, Cellpose.
Besides, the product wasn’t handily tricked by outrageous changes in a phone’s shape because of anti-toxin treatment or enmity by synthetics created during interbacterial hostility. Truth be told, the program demonstrated the way that it might identify cell inebriation in a preliminary utilizing E. coli.
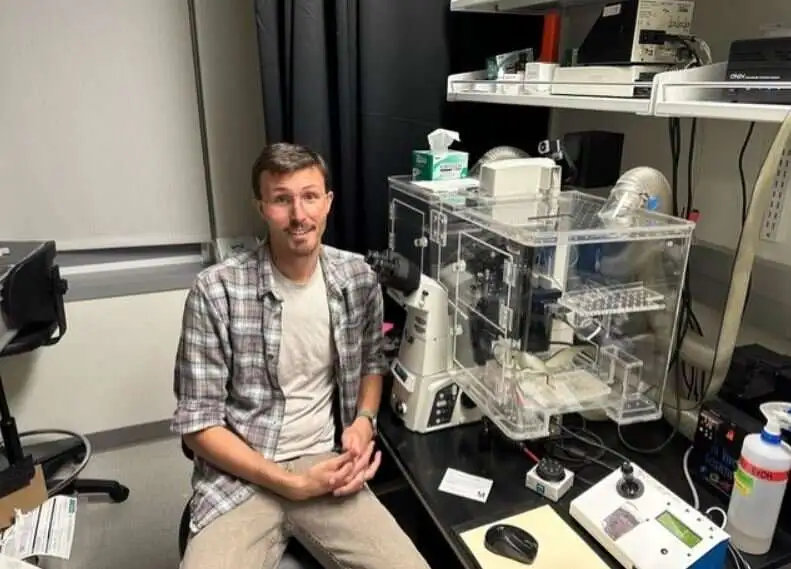
Kevin Cutler, a College of Washington graduate student in physical science, drove the turn of events and testing of Omnipose, a profound learning program for recognizing microbes in micrographs. Furthermore, Omnipose performed well in overcoming recognition issues caused by differences in optical qualities among various microbes.
Most microbes are circles or bars, yet some have other essential structures, like winding twisting. Other than these, Omnipose could recognize more intricate microbes with extended shapes or with branches, fibers, and limbs, all actual qualities that can make it hard for profound learning devices to suss out which microorganisms are available in a picture.
The program truly does, in any case, confront a few limits in dealing with object cross-over in a 2D version of a 3D example of a packed microbial local area. Object cross-over produces, for instance, the impact of a clock on a wall, giving the deception of jumping out of an individual’s head in a photo.
In examining cells in a root early stage informational index from the quickly developing weed A, thaliana, Omnipose showed a few benefits over past methodologies in this 3D example.
Different surveys by the Mougous lab group of Omnipose’s capacities showed microbes under a specific edge in size can be difficult for the device to suss out.
In spite of these downsides, the scientists accepted that Omnipose could be an answer. They noted that it could “assist with responding to different inquiries in bacterial cell science.”
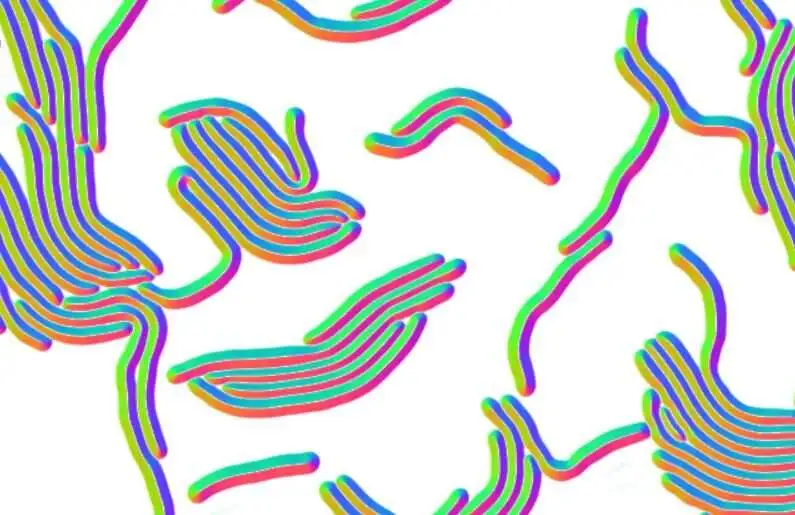
As a feature of the Omnipose profound learning device, a brain network-based result, or stream field, was obtained from a microscopy image of extended bacterial cells.To check whether it could likewise be turned into a multifunctional device in other natural or even non-life sciences fields subject to microscopy, the researchers evaluated the program on micrographs of the super small roundworm C. elegans is a crucial creature in hereditary, neuroscience, formative, and microbial research.Like a few microbes, this animal has an extended shape. In the same way as other different worms, it can likewise bend itself. Omnipose could select C. elegans, regardless of its various stretches, withdrawals, and developments.This capacity could be helpful, for example, in brain investigations of C. Following a time-pass, motion occurs.
In planning devices like Omnipose, scientists are taking a gander at a size of single-pixel accuracy to characterize the limits of a cell. That is on the grounds that most bacterial cell self-perceptions are made up of just a few pixels. The scientists made sense of the fact that characterizing limits inside a picture is called division. They created Ominpose through profound brain organization and high-accuracy division calculation. Their tests revealed that Omnipose has an unusual division precision.
The researchers planned Omnipose for use by common exploration labs and made its source code available, preparing information and models openly accessible, alongside documentation on the most efficient method to utilize the program.
The analysts wrote in their report that “we guess that the elite exhibition of Omnipose across shifted cell morphologies and modalities” may have opened data from microscopy pictures that were already distant.
“Mirroring the significance of the issue, this is a packed field,” Mougous said. “Mirroring the significance of the issue, this is a packed field,” Mougous said. However, Kevin’s answer stands apart from the pack. We accept it will be a unique advantage for organic picture examination.
More information: Paul Wiggins, Omnipose: a high-precision morphology-independent solution for bacterial cell segmentation, Nature Methods (2022). DOI: 10.1038/s41592-022-01639-4. www.nature.com/articles/s41592-022-01639-4
Journal information: Nature Methods