The new appearance of generative models, computational devices that can produce new texts or pictures in light of the information they are prepared on, opened fascinating additional opportunities for imaginative businesses. They make it simple for artists and digital content creators to create realistic media content that incorporates elements from a variety of images or videos, for instance.
A new model that can realistically insert specific humans into various scenes, such as showing them exercising in the gym, watching a sunset on the beach, and so on, has been developed by researchers at Stanford University, UC Berkeley, and Adobe Research.
A paper that was pre-published on the arXiv server and will be presented at the Conference on Computer Vision and Pattern Recognition (CVPR) 2023 in Vancouver this June introduced their proposed architecture, which is based on a class of generative models known as diffusion models.
“Our large-scale generative model, trained on a dataset of millions of videos, provides better generalization to new scenes and people. Furthermore, our model has a variety of exciting auxiliary capabilities, such as person hallucination and virtual try-on.”
Sumith Kulal, one of the researchers who carried out the study,
According to Sumith Kulal, one of the study’s authors, “visual systems inherently possess the ability to infer potential actions or interactions that an environment or a scene allows,” or “affordances.”
“In the fields of vision, psychology, and cognitive sciences, this has been the subject of extensive research. Due to inherent limitations in their methodologies and datasets, computational models for affordance perception developed over the past two decades were frequently constrained. Nonetheless, the great authenticity exhibited by huge-scope generative models showed a promising road for progress. We wanted to develop a model that could specifically identify these advantages using these insights.”
The essential target of the concentration by Kulal and his partners was to apply generative models to the assignment of affordance discernment in the desire to accomplish more reliable and practical outcomes. They specifically addressed the issue of realistically inserting a person into a scene in their most recent paper.
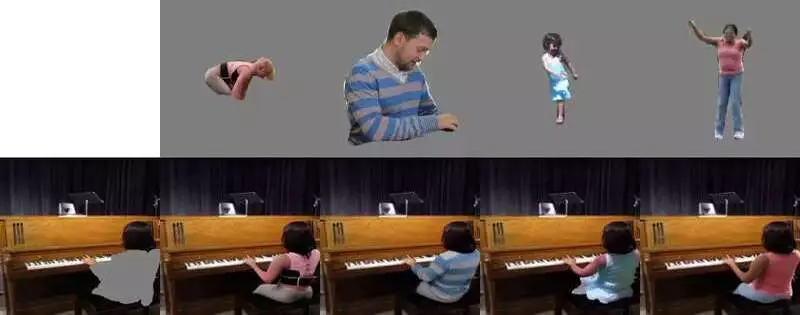
Displaying the model’s auxiliary tasks at inference time, such as creating a scene that is appropriate for a particular person, swapping clothes in a virtual try-on environment, and hallucinating a person who is compatible with the scene. Credit: Kulal et al.
Kulal and colleagues explained, “Our inputs include an image of a person and a scene image with a designated region, and the output is a realistic scene image now including the person.” Our large-scale generative model, which was trained on a dataset of millions of videos, is better able to generalize to new people and scenes. Besides, our model shows a scope of charming helper capacities, for example, individual visualization and virtual take a stab at.”
Using a self-supervised training method, Kulal and his colleagues trained a diffusion model, a type of generative model that can transform “noise” into a desired image. Dissemination models basically work by “annihilating” the information they are prepared on, adding “commotion” to it, and afterward recuperating a portion of the first information by switching this interaction.
The researchers fed their model videos of a human moving through a scene as part of its training, and it randomly selected two frames from each video. The people in the principal outline are veiled, implying that a locale of pixels around the human is grayed out.
The model then attempts to recreate people on this covered edge by utilizing something similar, exposed people in the second casing, as a molding signal. Over the long run, the model can, in this manner, figure out how to sensibly repeat how people would look assuming that they were put in unambiguous scenes.
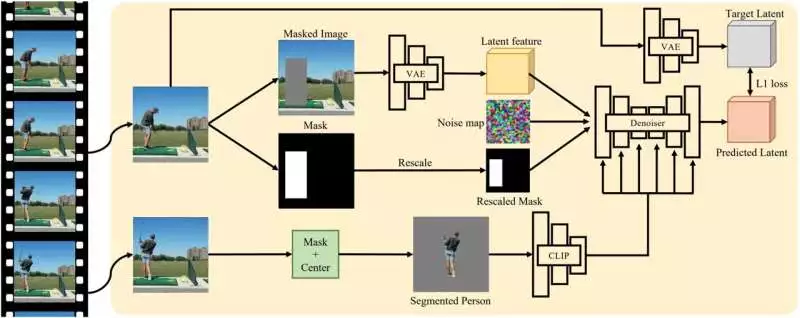
plan for training with self-supervision. Two arbitrary edges are removed, with the individual in the main casing being covered out. The individual from the subsequent casing is then used as a molding component to ink the picture. Credit: Kulal et al.
“Our technique forces the model to derive a potential posture from the scene setting, re-represent the individual, and blend the inclusion,” Kulal said. “Our dataset, which consists of millions of human videos, is an essential component of this strategy. Our model, whose architecture is comparable to that of the stable diffusion model, generalizes exceptionally well to various inputs due to its scale.”
In a series of preliminary tests, the researchers fed their generative model new images of people and scenes to see how well it placed these people in the scenes. They discovered that it worked exceptionally well, producing edited images that appeared very realistic. Their model’s affordance predictions are superior to those of previous non-generative models and work in a wider range of contexts.
Kulal stated, “We were delighted to observe the model’s effectiveness for a broad range of scene and person images, accurately identifying the appropriate affordances in most cases.” We guess that our discoveries will altogether add to future examinations in affordance discernment and related regions. There are also significant repercussions for robotics research, where identifying potential interaction opportunities is essential. Besides, our model has down-to-earth applications in making practical media (like pictures and recordings).”
Later on, the model created by Kulal and his partners could be coordinated inside various innovative programming apparatuses to expand their picture-altering functionalities, finally supported by specialists and media makers. It could also be added to smartphone photo editing applications, making it easier and more realistic for people to add people to pictures.
Kulal continued, “This work offers several potential avenues for future exploration.” Recent works like ControlNet provide pertinent insights, so we are thinking about making the generated poses more controllable. This system could also be expanded to produce moving-person videos rather than static images in scenes. We also want to know how efficient our models are and whether we can get the same quality from a smaller, faster model. At long last, the techniques introduced in this paper aren’t limited to people; this method could be applied to all objects.
More information: Sumith Kulal et al, Putting People in Their Place: Affordance-Aware Human Insertion into Scenes, arXiv (2023). DOI: 10.48550/arxiv.2304.14406