Prof. Liu Yang from the College of Chinese Foundation of Sciences (UCAS), in a joint effort with her partners from Renmin College of China and Massachusetts Establishment of Innovation, has proposed an original organization, in particular the physical science encoded repetitive convolutional brain organization (PeRCNN), for displaying and revelation of nonlinear spatio-fleeting dynamical frameworks in light of meager and boisterous information.
The methodology can be applied to various issues, for example, response dissemination processes and other halfway differential condition (PDE) frameworks, including forward and reverse investigation, information-driven demonstration, and revelation of PDEs. The earlier physical science information is effectively “encoded,” which gives the organization interpretability.
Specifically, the specialists proposed a profound learning system that effectively encodes a given physical science structure in a repetitive convolutional brain organization to work with learning of the spatiotemporal elements in scanty information systems. The paper is distributed in the journal Nature Machine Knowledge.
They found that such a computational worldview shows high exactness, heartiness, interpretability, and generalizability and exhibited the capacities of the proposed network engineering by applying it to different errands in the logical demonstration of spatiotemporal elements, for example, response dispersion processes.
As a rule, foreseeing the development of mind-boggling spatiotemporal dynamical frameworks is a moving undertaking much of the time because of deficient earlier information and the absence of an express PDE definition to portray the nonlinear course of the framework factors. Customary AI strategies need to depend on a lot of preparation and experience the ill effects of issues like unfortunate interpretability, powerless speculation, and wild demonstrating blunders.
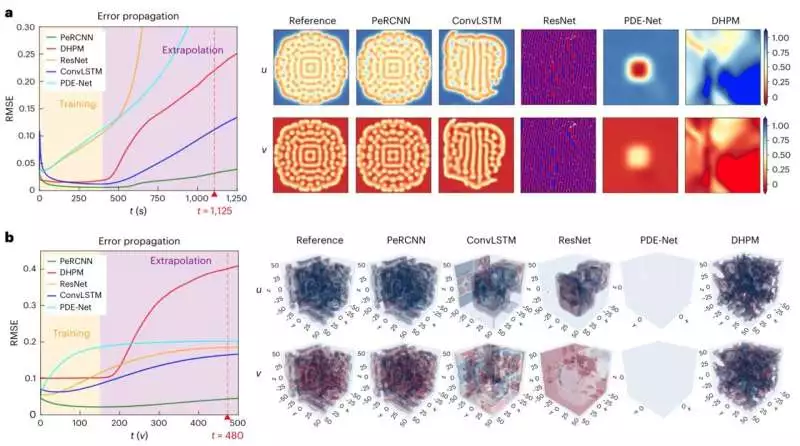
Blunder spreads the bend of expectation and the extrapolated depictions from every information-driven model contrasted with the reference arrangement. Credit: UCAS
On account of the advancement of information-driven approaches, it is feasible to gain spatio-fleeting elements from estimation information while adding earlier material science information. In any case, existing material science-based AI ideal models force actual regulations or overseeing conditions through delicate punishment imperatives, and the arrangement quality to a great extent relies upon an experimentation-legitimate setting of hyperparameters. In this way, it is of extraordinary need to foster a new information implanted gaining model to gain the hidden spatiotemporal elements from information.
As indicated by the scientists, a significant benefit of the PeRCNN is that the earlier material science information can be encoded into the organization, which ensures that the subsequent organization stringently submits to the given physical science. This has huge benefits for working on the union and the exactness of the model. By encoding the given actual construction into the intermittent convolutional brain organization, the exhibition of displaying spatiotemporal dynamical frameworks in light of meager and boisterous information has been moved along.
Through broad mathematical trials, they demonstrated the way that the proposed approach can be applied to display and find an assortment of response dispersion processes and other PDE frameworks. By contrasting the proposed approach with a few existing techniques (otherwise called standard models), they found that the methodology reliably beats (albeit not as well as) the considered baselines under various noise levels and information wealth.
What’s more, the scientists coordinated the inadequate relapse method with the PeRCNN model to find the exact type of PDEs. The whole strategy comprises three stages: information reproduction, scanty relapse, and tweaking of coefficients.
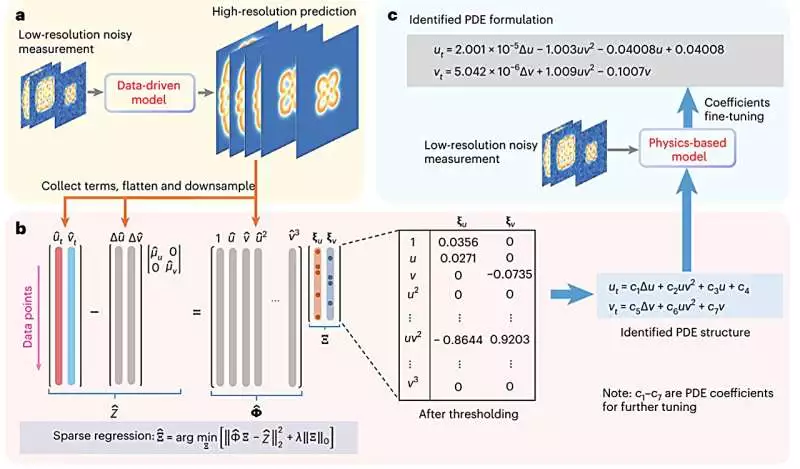
Flowchart of the disclosure of overseeing PDEs Credit: UCAS
This study is supposed to propel the improvement of information-driven displaying of complicated spatiotemporal dynamical frameworks, furnishing researchers and architects with additional amazing assets for understanding and anticipating normal and designing peculiarities.
The methodology, which joins profound learning and their earlier physical science information, is supposed to be pertinent in different subjects and assume a significant part, including liquid mechanics, organic chemistry, natural science, designing, and materials science, and that’s only the tip of the iceberg.
The analysts anticipate seeing a further turn of events and the utilization of this new methodology, which might disentangle more secrets about complex spatiotemporal dynamical frameworks and bring new leaps forward for future logical and mechanical turns of events.
More information: Chengping Rao et al, Encoding physics to learn reaction–diffusion processes, Nature Machine Intelligence (2023). DOI: 10.1038/s42256-023-00685-7