By only looking at data from noninvasive brain imaging without taking into account information from the hands themselves, researchers at the University of California, San Diego, were able to distinguish between different hand gestures that people were using. The outcomes are an early move toward fostering a harmless cerebrum PC interface that may one day permit patients with loss of motion, cut-off appendages, or other actual difficulties to utilize their psyche to control a gadget that helps with regular undertakings.
The study, which was recently published online ahead of print in the journal Cerebral Cortex, is the best one yet for using magnetoencephalography (MEG), a completely noninvasive method, to differentiate single-hand gestures.
“Our objective was to sidestep intrusive parts,” said the paper’s senior creator, Mingxiong Huang, Ph.D., co-head of the MEG Place at the Qualcomm Organization at UC San Diego. In addition, Huang is associated with the Veterans Affairs (VA) San Diego Healthcare System, the Department of Radiology at the UC San Diego School of Medicine, and the Department of Electrical and Computer Engineering at the UC San Diego Jacobs School of Engineering. MEG gives a protected and exact choice for fostering a mind-PC interface that could at last assist patients.”
“All I have to do is put the MEG helmet on their head.” There are no electrodes that could break while implanted within the head; there is no pricey, delicate brain surgery; and there is no risk of brain infection.”
Author Roland Lee, MD, director of the MEG Center at the UC San Diego Qualcomm Institute.
The advantages of MEG, which detects the magnetic fields produced by neuronal electric currents moving between brain neurons using an embedded 306-sensor helmet, were emphasized by the researchers. Electrocorticography (ECoG), which requires surgically implanting electrodes on the surface of the brain, and scalp electroencephalography (EEG), which locates brain activity with less precision, are alternative brain-computer interface techniques.
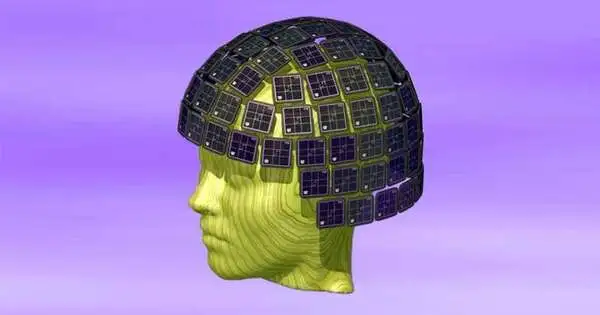
The new study from the Qualcomm Institute at UC San Diego made use of machine learning and magnetoencephalography (MEG), a noninvasive imaging method. The 306-sensor MEG helmet, which measures the magnetic field to detect nerve activity in the brain, is shown here. Credit: MEG Center at UC San Diego Qualcomm Institute.
MEG Center at the UC San Diego Qualcomm Institute According to study co-author Roland Lee, MD, director of the MEG Center at the UC San Diego Qualcomm Institute, emeritus professor of radiology at the UC San Diego School of Medicine, and physician with the VA San Diego Healthcare System, “With MEG, I can see the brain thinking without taking off the skull and putting electrodes on the brain itself.” All that remains is for me to put the MEG helmet on their head. There are no cathodes that could break while embedded inside the head, avoiding costly and delicate brain surgery and possible infections of the brain.”
Lee compares the wellbeing of MEG to taking a patient’s temperature. ” Similar to how a thermometer measures your body’s heat, MEG measures the magnetic energy your brain produces. That makes it totally painless and safe.”
Rock-paper-scissors The current study looked at whether 12 volunteers’ hand gestures could be distinguished using MEG. As in previous studies of this kind, the volunteers were given the MEG helmet and randomly instructed to make one of the gestures in the game Rock, Paper, Scissors. MRI images were overlayed with MEG functional information, which provided structural information about the brain.
A high-performing deep learning model known as MEG-RPSnet was created by Yifeng (“Troy”) Bu, a Ph.D. student in electrical and computer engineering at the UC San Diego Jacobs School of Engineering and the first author of the paper, to interpret the generated data.
“The unique component of this organization is that it joins spatial and worldly elements at the same time,” said Bu. “This is primarily why it performs better than previous models.
The study’s findings revealed that the researchers’ methods could be used to distinguish between hand gestures with more than 85% accuracy. These outcomes were practically identical to those of past examinations, with a much more modest example size utilizing the obtrusive ECoG mind PC interface.
Additionally, the team discovered that MEG measurements from only half of the brain regions that were sampled were able to produce results with only a small (2–3%) loss of accuracy. This suggests that future MEG helmets may require fewer sensors.
“This work builds a foundation for future MEG-based brain-computer interface development,” Bu stated in regard to the future.
Notwithstanding Huang, Lee and Bu, the article, “Magnetoencephalogram-based mind PC interface for hand-motion interpreting utilizing profound learning,” was composed by Deborah L. Harrington, Qian Shen and Annemarie Angeles-Quinto of VA San Diego Medical services Framework and UC San Diego Institute of Medication; The VA San Diego Healthcare System’s Hayden Hansen; Zhengwei Ji, Jaqueline Hernandez-Lucas, Jared Baumgartner, Tao Melody and Sharon Nichols of UC San Diego Institute of Medication; Dewleen Cook of VA Focus of Greatness for Stress and Emotional well-being and UC San Diego Institute of Medication; Imanuel Lerman of the University of California, San Diego, and the VA Center of Excellence for Stress and Mental Health; and UC San Diego’s Tuo Lin and Xin Ming Tu, as well as Ramesh Rao, director of the Qualcomm Institute.
More information: Yifeng Bu et al, Magnetoencephalogram-based brain–computer interface for hand-gesture decoding using deep learning, Cerebral Cortex (2023). DOI: 10.1093/cercor/bhad173