PC vision calculations have progressively progressed over the course of the last many years, empowering the improvement of refined advances to screen explicit conditions, identify objects of interest in video film, and uncover dubious exercises in CCTV accounts. A portion of these calculations are explicitly intended to identify and disconnect moving items or individuals of interest in a video, an errand known as moving objective division.
While a few regular calculations for moving objective division achieved promising outcomes, the greater part of them performed ineffectively continuously (i.e., while examining recordings that were not pre-recorded yet are being caught right now). Some examination groups have consequently been attempting to handle this issue by utilizing elective kinds of calculations, for example, alleged quantum calculations.
Specialists at Nanjing College of Data Science and Innovation and Southeast College in China have, as of late, fostered another quantum calculation for the division of moving focuses in grayscale recordings. This calculation, distributed in Cutting-edge Quantum Advancements, was found to outflank old-style approaches in errands that include the continuous examination of video film.
“Our previous work has been devoted to quantum image segmentation algorithms, and we have published several papers on the subject, but those have all been on static targets. We wondered if we could segment moving targets and extend the application from image to video. We looked into related research on quantum video and discovered that it is rare.”
Wenjie Liu, one of the researchers who carried out the study,
“Our past work has been given to quantum picture division calculations, and we have distributed a few papers; however, those are on static targets,” Wenjie Liu, one of the specialists who completed the review, told Tech Xplore. “We contemplated whether we could additionally portion moving targets and stretch out the application from picture to video. We checked the connected examination on quantum video and observed that the exploration in this space is scant.”
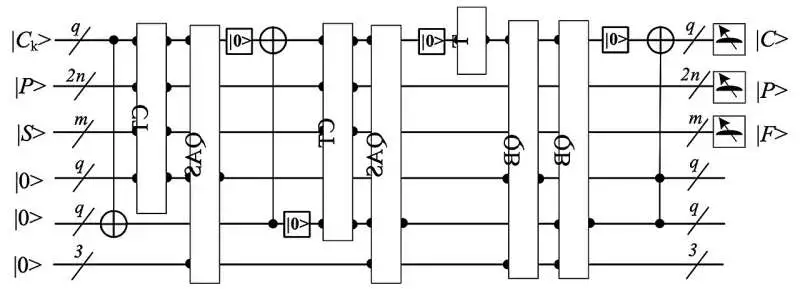
Quantum circuit acknowledgment of the total quantum calculation. Credit: Liu, Wang, and Wu.
The calculation created by Liu and his associates uses quantum mechanical cycles to fragment moving focuses in grayscale recordings quickly. This fragmented individual or protest can then be perceived as objective and observed using extra computational strategies.
“In the first place, we store the traditional grayscale video into some qubits, let the casing and pixel positions be in superposition state, and let the grayscale upsides of the pixels be entrapped with them to acquire the quantum video Vk,” Liu said. “Then the video is circularly moved to get two other new recordings, Vk-1 and Vk+1, in which the places of the casings are moved forward and in reverse by one unit, separately, contrasted with the first video. These three recordings share positional qubits. The two recently gotten recordings are then permitted to do outright esteem deduction activity with the first video, i.e., Bk-1=|Vk-Vk-1|,Bk+1=|Vk-Vk+1|. Then, at that point, Bk-1 and Bk+1 are binarized to get bk-1 and bk+1. bk-1 and bk+1 are added to the eventual outcome, and afterward estimations are performed to reestablish the exemplary video.”
To permit their calculation to segregate among edge and grayscale values, Liu and his partners consolidated a supposed quantum comparator that requires less computational power. Moreover, the group planned different quantum circuit units using fewer quantum pieces and quantum entryways.
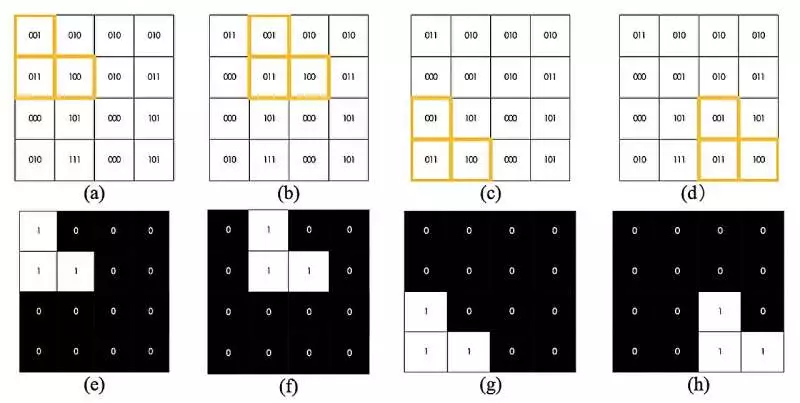
A schematic graph of the first video and the handled video (a-d) is the four back-to-back outlines in the first video, where the noticeable part is the moving objective, and (e-h) is the four successive casings in the fragmented video, where the moving objective is portioned and set apart with 1. Credit: Liu, Wang, and Wu.
“The total quantum circuit was collected in light of these units,” Liu said. “Contrasted with the exemplary partner, we accomplished a remarkable speedup, and the calculation’s intricacy is likewise better than the current quantum calculations. The pixel positions in a picture are put away into quantum bits in the superposition state, and afterward, the quantum pieces of dark worth data are entrapped with the position data quantum bits, so a picture or a video is in the superposition state. The computational procedure on a pixel in the picture (or video) in the superposition state is identical to the computational procedure on the entire picture (or the entire video), so the computational intricacy can be diminished dramatically.”
Liu and his partners assessed their calculation in a progression of tests, contrasting its exhibition with that of a traditional calculation for moving objective division. Their calculation was viewed as essentially quicker than traditional models while holding a similar exactness.
“The intricacy of the calculation is dramatically diminished compared with its old-style partner,” Liu said. “Likewise, we planned a quantum comparator with fewer quantum doors and qubits, which can be utilized in any errand that requires examination of values and can really decrease the intricacy of the calculation.”
The likelihood histogram of the subsequent video The calculation was recreated on an IBM Q. Four casings of video were fragmented, and afterward, the divided video was estimated to get a likelihood histogram. C means the grayscale esteem, P indicates the pixel position, and S means the edge position. Credit: Liu, Wang, and Wu.
Later on, the calculation made by this group of analysts could be grown further and tried on a more genuine film. Simultaneously, this work could motivate the improvement of new, exceptionally performing quantum calculations for other high-level PC vision applications.
“As of now, our examination centers around how to handle pictures all the more just in the spatial space with quantum circuits, and the impact can be additionally improved,” Liu added. “Later on, to get better handling results, we will expand our exploration to handling pictures with quantum brain organizations. What’s more, the current quantum innovation can’t understand the utilization of enormous scope qubits temporarily, so the crossover traditional quantum brain network is likewise a superior decision.”
More information: Wenjie Liu et al. A Quantum Moving Target Segmentation Algorithm for Grayscale Video, Advanced Quantum Technologies (2023). DOI: 10.1002/qute.202300248. On arXiv: DOI: 10.48550/arxiv.2310.03038