Simulated intelligence devices in view of fake brain organizations (ANNs) are being presented in a developing number of settings, assisting people with handling numerous issues quicker and more proficiently. Electronic engineers have been looking into the possibility of running these algorithms on other platforms, such as diffractive optical devices, despite the fact that the majority of them are implemented on conventional digital devices and computers.
A brand-new programmable neural network based on the so-called spoof surface plasmon polariton (SSPP), a surface electromagnetic wave that travels along planar interfaces, was recently developed by a team led by Prof. Tie Jun Cui at Southeast University in China. This recently proposed surface plasmonic brain organization (SPNN) engineering, presented in a paper in Nature Gadgets, can identify and deal with microwaves, which could be valuable for remote correspondence and other mechanical applications.
Qian Ma, one of the study’s authors, told Tech Xplore, “In digital hardware research for the implementation of artificial neural networks, optical neural networks and diffractive deep neural networks recently emerged as promising solutions.” High-level programmability and nonlinear computing can be difficult to achieve simultaneously, as previous optical neural network research has demonstrated. As a result, these ONN devices have typically only been utilized for straightforward recognition tasks (i.e., linear problems) or have been restricted to particular tasks that do not allow for programmability.
“Optical neural networks and diffractive deep neural networks have recently emerged as promising solutions in digital hardware research for the implementation of artificial neural networks,”
Qian Ma, one of the researchers who carried out the study,
The recent work done by these researchers’ primary goal was to make neural networks suitable for a wide range of applications and further enhance their performance on complex nonlinear problems. Their proposed architecture, SPNN, can be programmed to work with a variety of weight configurations, so it should theoretically work well for a variety of tasks.
The examination group led by Prof. Cui has been creating programmable satire surface plasmonic gadgets and investigating their utilization for electromagnetic guidelines for a considerable length of time. They decided to use one of these plasmonic devices to create a neural network with programmable weights and activation functions, inspired by their previous findings. On a basic level, the engineering they proposed could accomplish surprising handling speeds, moving toward the speed of light.
Ma explained, “The SPNN was created layer by layer, with multiple programmable SSPP supercells in each layer.” Eight programmable SSPP cells make up each supercell’s fully connected network of four in and four out. We cleverly realize the full connection’s characteristics in our three-dimensional composite structure.”
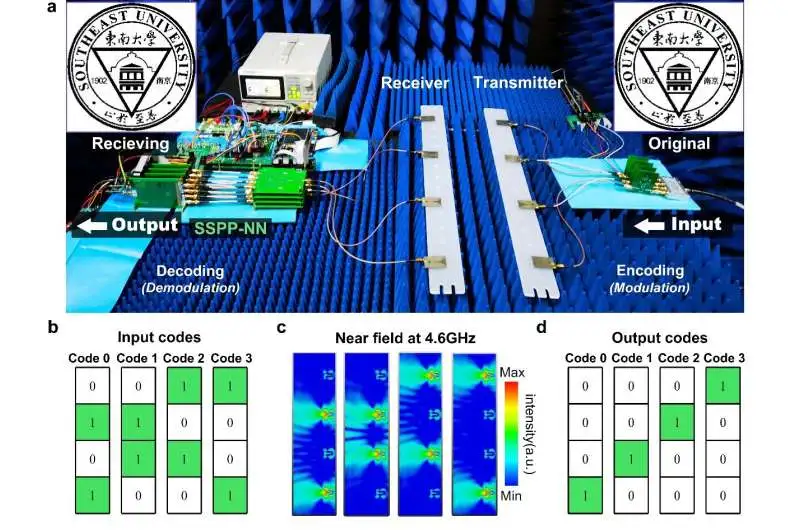
The deciphering component in a remote correspondence framework utilizing the SSPP supercell photo of the correspondence framework, in which a Southeast College logo has been sent and received by the SPNN. b, in the SSPP supercell’s encoding process, the input code mapping between channel signals and two-bit symbols c, the near-field patterns that occur between the transmitting and receiving antenna arrays under four different coding scenarios. a.u., inconsistent units d, The result code planning between the good-for-nothing images and direct signals in the unraveling system of the SSPP supercell The original code mapping is transformed into a simplified injective one through the decoding procedure that the SSPP supercell uses in the electromagnetic domain. Credit: Gao et al.
Each of the programmable supercells that the scientists used to make their foundation is comprised of a SSPP power divider and a coupler. This exceptional plan permits it to heartily control electromagnetic waves and then use them to acknowledge plasmonic brain organizations.
Ma stated, “The voltages of varactors loading on the couplers are adjusted to adjust the weight parameters of neural networks.” More importantly, the activation function can be altered by feeding the threshold back to an amplifier and detecting the input intensity with detectors. Additionally, the SPNN can be used to develop a wireless communication system for decoding and recovering images as well as a task for image classification.”
The team’s SPNN architecture’s programmable weights and activation functions, which could make it easier to apply to a wide range of tasks, are its most notable features. A few past works acknowledged programmable brain networks utilizing stage-change materials, yet this approach was found to bring about restricted power ranges.
“Albeit diffractive profound brain organizations can deftly tweak and cycle electromagnetic waves, their absence of nonlinear initiation works likewise restricts their capability to deal with additional perplexing issues like selective or (XOR) rationale activities,” Mama said.
“Some novel concepts may be introduced into this field by our proposed programmable SPNN based on digital surface plasmonic devices.” It is possible to construct programmable neural networks using SSPP devices with a simple architecture, low cost, and high efficiency for controlling electromagnetic waves. Additionally, we can implement programmable activation functions by utilizing a closed-loop feedback system between amplifier bias circuits and detection ports.”
Later on, the SPNN created by this group of scientists could be utilized to identify and deal with microwaves for a huge scope, subsequently possibly opening additional opportunities for 5G and 6G remote correspondences. Conversely, with a portion of the ANN-based answers for microwave recognition presented before, SPNN can straightforwardly regulate electromagnetic waves at speeds that approach the speed of light.
Prof. Cui, Ma, and their colleagues demonstrated in their paper that the same architecture also excels at other tasks, such as accurately classifying handwritten digits. In their next works, they intend to assess SPNN on different assignments while likewise expanding its intricacy so it can handle further developed issues.
Ma continued, “The prototype implemented in this work is based on a relatively low-cost, fully neural network.” The SPNN structure looks like a circuit framework, and that implies that the size of SPNN layers could be broadened without expanding the impression of the gadget. For instance, a cubic primary structure could be made to diminish the actual size of the somewhat associated framework, accomplishing super-high spatial utility in three-layered space. By enhancing the operating frequency band, we can also reduce the network’s size.”
More information: Xinxin Gao et al, Programmable surface plasmonic neural networks for microwave detection and processing, Nature Electronics (2023). DOI: 10.1038/s41928-023-00951-x
Journal information: Nature Electronics