The vulnerability of AI networks to malicious assaults is a major problem, and academics and specialists in the field are constantly working to comprehend and handle these issues. Artificial intelligence capabilities hold promise for applications ranging from driverless vehicles to medical image interpretation. However, a new study reveals that these AI tools are more vulnerable to targeted attacks than previously assumed, effectively forcing AI systems to make incorrect decisions.
The issue is what are known as “adversarial attacks,” in which someone manipulates the data input into an AI system in order to confuse it. For example, someone may be aware that placing a certain sort of sticker at a given location on a stop sign will effectively render the stop sign invisible to an AI system. A hacker may also install code on an X-ray machine that modifies image data in such a way that an AI system makes incorrect diagnoses.
“For the most part, you can make all sorts of changes to a stop sign, and an AI that has been trained to identify stop signs will still know it’s a stop sign,” says Tianfu Wu, co-author of the new paper and associate professor of electrical and computer engineering at North Carolina State University. “However, if the AI has a vulnerability, and an attacker knows the vulnerability, the attacker could take advantage of the vulnerability and cause an accident.”
Wu and his colleagues conducted a new investigation to determine how widespread these types of adversarial weaknesses are in AI deep neural networks. They discovered that the vulnerabilities are far more widespread than originally assumed.
This is incredibly important, because if an AI system is not robust against these sorts of attacks, you don’t want to put the system into practical use – particularly for applications that can affect human lives.
Tianfu Wu
“What’s more, we found that attackers can take advantage of these vulnerabilities to force the AI to interpret the data to be whatever they want,” Wu says. “Using the stop sign example, you could make the AI system think the stop sign is a mailbox, or a speed limit sign, or a green light, and so on, simply by using slightly different stickers — or whatever the vulnerability is.
“This is incredibly important, because if an AI system is not robust against these sorts of attacks, you don’t want to put the system into practical use – particularly for applications that can affect human lives.”
To test the vulnerability of deep neural networks to these adversarial attacks, the researchers developed a piece of software called QuadAttacK. The software can be used to test any deep neural network for adversarial vulnerabilities.
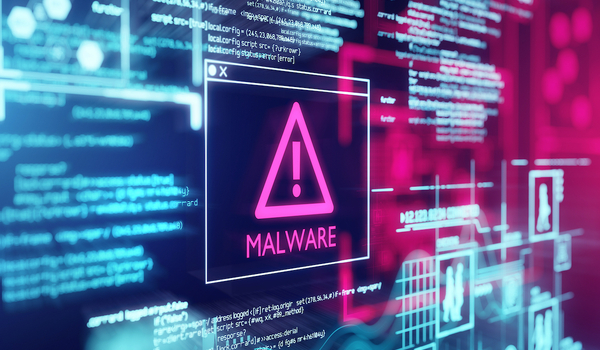
“Essentially, if you have a trained AI system and test it with clean data, the AI system will behave as predicted.” QuadAttack observes these activities and learns how the AI makes data-related judgments. This enables QuadAttacK to determine how the data can be altered to trick the AI. QuadAttack then begins feeding modified data to the AI system to test how the AI reacts. If QuadAttacK discovers a vulnerability, it can swiftly make the AI perceive anything QuadAttacK wants it to see.”
In proof-of-concept testing, the researchers used QuadAttacK to evaluate four deep neural networks: two convolutional neural networks (ResNet-50 and DenseNet-121) and two vision transformers (ViT-B and DEiT-S). These four networks were chosen because they are in widespread use in AI systems around the world.
“We were surprised to find that all four of these networks were very vulnerable to adversarial attacks,” said Wu. “We were particularly surprised at the extent to which we could fine-tune the attacks to make the networks see what we wanted them to see.”
QuadAttackK has been made freely available by the research team so that the research community can use it to test neural networks for weaknesses. You may find the program at https://thomaspaniagua.github.io/quadattack_web/.
“Now that we can better identify these vulnerabilities, the next step is to find ways to minimize those vulnerabilities,” Wu said. “We already have some potential solutions — but the results of that work are still forthcoming.”