Researchers used neuroimaging and artificial intelligence to identify novel brain signatures specific to each antidepressant response. UT Southwestern University-led research has identified MRI brain imaging biomarkers that will allow for greater precision in prescribing the most effective antidepressants.
Antidepressant response is typically associated with a 2–4 week lag before improvement is seen, and while improvement may occur during the first 2 weeks of treatment, it is difficult to differentiate true improvement from a placebo effect. Antidepressants typically take 4 to 6 weeks to take effect. Speak with your doctor if you are still experiencing symptoms after this time period. You might need to increase the dose of your current antidepressant medication or switch to a different one entirely.
The outcome predictive models were developed in part using data from a large multi-center study funded by the National Institute of Mental Health and published in the journal Biological Psychiatry. The findings provide compelling evidence that the current trial-and-error approach used in clinical practice to select the best antidepressant can be replaced by this new precision medicine approach.
It is a clear improvement over the current standard prediction approaches. We’ve also reached a point where our findings are stable and can be used to guide future research.
Dr. Trivedi
“This is a significant step forward. It is not invasive. It can and should be used right away. ” One of the pillars of the Peter O’Donnell Jr. Brain Institute is Madhukar Trivedi, M.D., Professor of Clinical Psychiatry and Director of the Center for Depression Research and Clinical Care.
According to Dr. Trivedi, the new biomarkers could save patients suffering from severe depression two to three months of taking the wrong medication. Severe depression over a long period of time can lead to job loss, marital dissolution, and even death by suicide. The study compared the commonly used antidepressant drug sertraline to a placebo group. Patients who did not respond to sertraline after eight weeks were switched to the antidepressant bupropion. Researchers measured changes in brain circuit reactions while study participants performed a rewarding task in the scanner. Non-invasive functional magnetic resonance imaging (fMRI) was performed on over 300 participants to assess changes in brain function at rest and during the rewarding task.
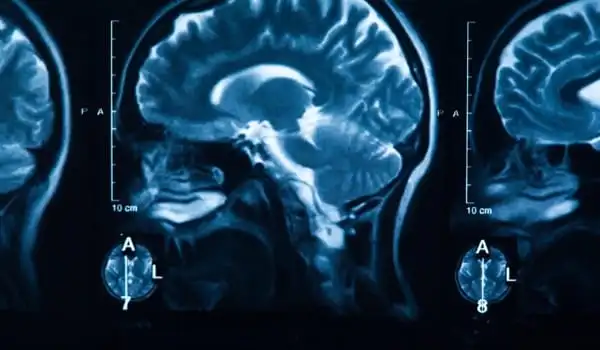
The study used that data and new innovations to build new machine learning models that tell scientists and clinicians which specific brain regions and circuits are associated with treatment response prediction for each medication.
“The signatures that we discovered are unique to each antidepressant’s response,” said Albert Montillo, Ph.D., Assistant Professor of the Lyda Hill Department of Bioinformatics, whose lab produced the 10,000 lines of code to efficiently tune new predictive models and sophisticated data cleaning methods to suppress fMRI head motion and achieve levels of accuracy not seen in previous lab tests.
“Because of the inherent complexity of the human brain, neuroscientists typically discover that brain activity can explain 15% of the variance in symptom relief. That would be a significant scientific breakthrough. 20% is a lot of money “Dr. Montillo stated “In this study, we can explain 48 percent of the variation in symptom relief from sertraline, 34 percent from bupropion, and 28 percent from placebo.”
Dr. Trivedi stated that the findings are highly credible because the underlying data used in the study is broadly representative of clinical data heterogeneity, including data from Massachusetts General Hospital in Boston, Columbia University in New York, and the University of Michigan, as well as the rigor of the analytic approach with the use of deep learning models.
Dr. Montillo developed methods to amplify the original fMRI data tenfold for the study, which is one of the first applications of deep machine learning to antidepressant outcome prediction. His work created models that predict outcomes reliably, particularly on patients who were not used to train the models.
“It is a clear improvement over the current standard prediction approaches,” Dr. Trivedi said. “We’ve also reached a point where our findings are stable and can be used to guide future research.”
“The analytical approach we have developed can easily be adapted to identify biomarker signatures and predict outcomes for other treatments of depression, both pharmacological and non-pharmacological,” Dr. Montillo added.
With a noninvasive approach and a wealth of evidence, Drs. Trivedi and Montillo believe clinicians should adopt this approach right away. They will seek additional funding to advance the research and determine whether it is compatible with blood biomarkers developed by Dr. Trivedi.