Misleadingly savvy brain organizations, prepared by pictures and recordings accessible on the web, can perceive faces and articles, and that’s just the beginning. Yet, there’s a serious downside. Showing AI calculations how to recognize individuals or things by depending exclusively on the visual library of appearances and articles found online underrepresents financial and segment gatherings.
A Harvard College AI scientist and teammates from ML Commons and Coactive man-made intelligence made a different dataset utilizing pictures of items tracked down in families all over the planet and prepared a brain organization to sort objects in view of that dataset. Their discoveries—introduced at the Meeting on Brain Data Handling Frameworks—uncover that the utilization of pictures from low-asset populations can emphatically support the item acknowledgment execution of AI frameworks.
“There haven’t yet been serious areas of strength for a value and equivalent portrayal to be incorporated into AI frameworks,” says Vijay Janapa Reddi, academic administrator at Harvard’s John A. Paulson School of Designing and Applied Sciences (Oceans) and a senior creator of the paper. “That is the higher perspective we’re attempting to catch with this exploration.”
“We must be aware of deeper biases in machine learning algorithms. The same word may be used to describe stoves all over the world, but what is labeled a stove in underserved areas and what is seen in wealthy homes can appear and function very differently.”
Vijay Janapa Reddi, associate professor at Harvard’s John A.
Reddi, who is likewise a vice president and board member at ML Hall, a consortium of scholastic and industrial pioneers in simulated intelligence, collaborated with partners to prepare a brain network utilizing a dataset of 38,479 pictures of family objects. The assortment of photos taken in 404 homes across 63 nations in Africa, America, Asia, and Europe is known as “Dollar Road” and was first evolved by the Gapminder Establishment. The Swedish-based element sent photographic artists all over the planet to hoard pictures of toothbrushes, latrines, televisions, ovens, beds, lights, and different articles tracked down in the homes of families with month-to-month salaries between the U.S. equivalent of $26.99 and $19,671.
“We should be perceptive of more profound predispositions in our AI frameworks,” Reddi says. “A similar word may be given to depict ovens all over the planet, yet on the off chance that you see what is known as an oven in underrepresented regions versus what’s found in well-off homes, those items can look and work totally differently in an unexpected way.”
In their paper, the scientists portray one more striking model: in a few unfortunate homes all over the planet, an individual could utilize their hand to clean their teeth. In the Dollar Road dataset, then, at that point, an image of somebody’s hand may be named both “hand palm” and “toothbrush.”
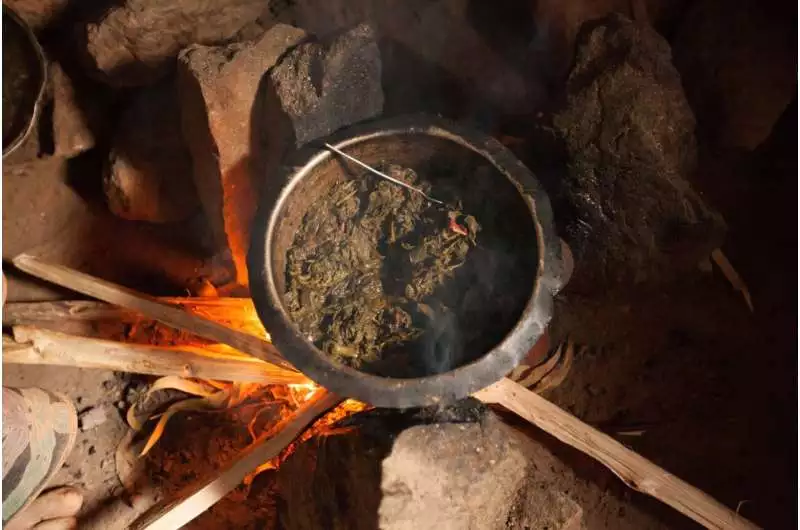
A family in Burundi purchased an oven for $37 USD per month.
Utilizing the Dollar Road picture assortment, which was formed by MLCommons into a strong dataset containing object names and labels, geographic information, and family month-to-month pay, the group found that their prepared brain network performed radically better compared to driving edge frameworks at precisely characterizing family things, particularly protests tracked down in homes with lower salaries. Their AI calculation accurately recognized objects by 65%, which is all the more impressive every now and again when contrasted with the generally utilized brain organizations—including ImageNet and Open Pictures—prepared on less assorted datasets obtained from the web.
“It’s stunning to see what the best-in-class AI models underestimate and how ineffectively they perform at accurately distinguishing objects in lower-asset settings,” Reddi says.
As industry and government depend progressively on AI frameworks to handle data and simply decide, Reddi says this evidence of idea research exhibits the risk of brain networks being prepared without comprehensive information addressing low-asset populations.
“Dollar Road has been an incredible asset for combating human erroneous judgments and prejudices, and we believe it can possibly do the same for machines,” says Cody Coleman, co-senior creator of the paper and President and primary supporter of Coactive’s artificial intelligence.
“Dollar Road shows the significance of information in AI from an overall perspective, and explicitly the capacity of painstakingly chosen information to outsizedly affect predisposition,” says David Kanter, a co-creator on the paper, who is organizer and leader head of MLCommons. “My expectation is that by facilitating and maintaining Dollar Road, we will engage the research community and industry to foster methods so that AI benefits everyone around the world, particularly in less developed areas.”
“Misleadingly shrewd frameworks, on the off chance that they are not assembled impartially and comprehensively, will speed up the split between the high-asset networks and the low-asset ones,” Reddi says. “While you’re building datasets to prepare AI frameworks and you’re fabricating that information from a high-asset place and not making a special effort to secure and incorporate information from lower-asset regions, the ramifications for learned inclination become much greater.” “Mindful man-made intelligence implies making AI all around the world available and internationally accessible.”
More information: The Dollar Street Dataset: Images Representing the Geographic and Socioeconomic Diversity of the World. openreview.net/forum?id=qnfYsave0U4