A more realistic model of the visual system could aid neuroscientists and clinicians in developing more effective treatments for alexia, prosopagnosia, and agnosia. Damage to the inferotemporal (IT) cortex, which processes visual information in the brain, can be severe, especially in adults. Those who are affected may lose their ability to read (a condition known as alexia), recognize faces (prosopagnosia), or distinguish objects (agnosia), and there is currently little doctors can do.
A more precise model of the visual system could aid neuroscientists and physicians in developing more effective treatments for various diseases. Researchers from Carnegie Mellon University created a computational model that allows them to recreate the spatial organization or topography of the IT and learn more about how adjoining clusters of brain tissue are formed and interact. This may also assist them in understanding how damage to that area affects their ability to recognize faces, objects, and scenes.
The model was described in the Proceedings of the National Academy of Sciences by Nicholas Blauch, a Ph.D. student in the Program in Neural Computation, and his advisors David C. Plaut and Marlene Behrmann, both professors in the Department of Psychology and the Neuroscience Institute at CMU.
We’ve been wondering for a long time whether we should think of the network of regions in the brain that respond to faces as a separate entity only for detecting faces, or if we should think of it as part of a broader neural architecture for object identification.
Nicholas Blauch
According to Blauch, the article could assist cognitive neuroscientists answer long-standing issues regarding how different areas of the brain interact.
“We’ve been wondering for a long time whether we should think of the network of regions in the brain that respond to faces as a separate entity only for detecting faces, or if we should think of it as part of a broader neural architecture for object identification,” Blauch said. “We’re attempting to approach this problem through a computational model that assumes this simpler, broad organization, and seeing if this model can then explain for the specialization we see in the brain through learning to perform tasks.”
To do this, the researchers created a deep learning model with extra aspects of biological brain connectivity, hypothesizing that the model may reveal the spatial arrangement, or topography, of the IT.
“The brain does not have an infinite volume,” Blauch explained. “It needs to try to keep the amount of white matter – used to connect different areas of the brain – to a minimum required for optimal communication, so there is space for more gray matter – or neurons – to compute information.”
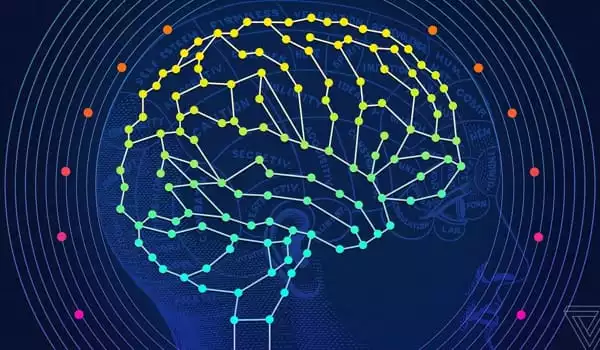
Blauch further explained that most connections between parts of the brain are mediated by excitatory neurons, whereas connections within a brain area are mediated by both excitatory and inhibitory neurons. In most deep learning models, artificial neurons may both excite and inhibit other neurons.
Following these guidelines, the researchers created a simple network architecture and a cost function that prioritizes learning to recognize images while attempting to keep links as short as possible. The scientists taught the model, known as an Interactive Topographic Network, to detect photos from many domains, including faces, objects, and settings. They discovered that once the model learnt to recognize these images, it formed specific spatial zones for each domain, as seen in the brain.
Following that, they replicated lesions, or brain injury, in each location. When they introduced lesions to the part of the model that was selective for face identification, they noticed a significant decrease in the model’s capacity to distinguish faces. They obtained the same conclusion for the object and scene domains. They also noticed that the damage was not fully consistent.
“There’s some lingering damage to the other domains,” Blauch admitted. “It is modest in comparison to the chosen domain, but it demonstrates that specialization within these networks can be strong but also mixed. This, together with the general principles used by the entire system, suggests that it is better thought of as a single system with internal specialization rather than a collection of discrete modules.”
In comparison to adults with equal injury, a generic, flexible system may be more capable of rearrangement after damage, as observed in children who largely recover visual function following impairment early in life.
The researchers intend to expand the model to study further concerns concerning the visual system, such as links between IT organization and other sections of the brain.