Sir Donald Bradman utilized a batting strategy that fans cherished but a few pundits pummeled. A basic part was his parallel batting backlift method. Exceptionally effective players used it right up until today.
Man-made reasoning (AI) can now tell straight backlift hitters from parallel ones, utilizing video as it were. Analysts from the University of Johannesburg (UJ) assembled the main profound learning PC vision AI model to do this. Their examination is distributed in Scientific Reports.
Later on, coaches may give more specific criticism to players who use such technology or more effectively distinguish players with sidelong backlifts.
Incredible procedure
In that brief instant not long before a cricket bowler delivers a ball at the wrinkle, the player starts an unpretentious development, moving their bat into position. They select it based upon the arrangement of the game and the sort of bowler moving toward them. Most quick bowlers will more often than not bowl at somewhere in the range of 140 and 150 km/h (87 to 93 mph), says Prof. Habib Noorbhai.
Noorbhai is presently overseer of the Biomedical Engineering and Healthcare Technology (BEAHT) Research Center at the University of Johannesburg (UJ). He is additionally a games researcher and has worked with local and worldwide cricket crews beginning in 2010. His doctoral dissertation was the first to hypothesize the parallel batting backlift procedure in cricket.
Another element is the hitter’s senses and preparation. Will they raise the bat straight with its face toward the ground or the stumps? Or, on the other hand, will they point the essence of the bat outward towards second slip or the wicket guardian? This is the procedure that the amazing hitter Sir Donald Bradman utilized. Effective test cricket hitters likewise used it right up until today.
Artificial intelligence assists mentors
The scientists, Tevin Moodley, Dustin van der Haar, and Habib Noorbhai, all played cricket at school.
Moodley is a doctoral understudy and Van der Haar is an example of an acknowledgment scientist. Both are at the Academy of Computer Science and Software Engineering (ACSSE), University of Johannesburg.
Moodley played common cricket in elementary school and kept playing cricket all through high school. He has always had a passion for the game and has combined AI with cricket, determined to make significant contributions to the cricketing scene.He utilized AI to perceive different batting strokes in past exams.
UJ analysts fabricated the primary profound learning AI model to recognize which backlift style cricket hitters use—straight or parallel. It robotizes the method involved with dissecting players’ batting styles, requiring video as it were. The best cricket batsman, Sir Donald Bradman, utilized a parallel backlift. Photographer: Therese van Wyk, University of Johannesburg
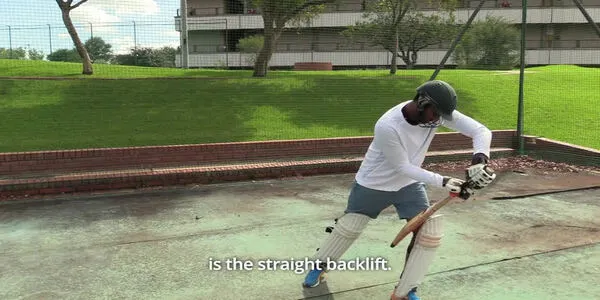
For this review, he zeroed in on getting AI to do the unpretentious errand of recognizing batting backlift style.
“I was contemplating whether I could mechanize that interaction,” says Moodley.
“For what reason does a mentor need to think, ‘Is that straight or parallel?’ If they know, they can say “You have a horizontal backlift.” I can now help you as such. ” I’m attempting to take extremely manual processes and robotize them, so we can assist batsmen with their procedures. “
Extraordinary and self-trained
Sir Bradman was brought into the world in 1908 in the little country town of Cootamundra in Australia, around 350km from Sydney. He was self-educated. As a teenager with academic credentials, he detonated on the Australian test group.
Bradman’s whimsical parallel batting backlift batting procedure was fairly questionable in his time. He was cruelly condemned by some. Yet, onlookers cherished what he brought to the game, and he set records that still stand.
Right up to the present day, a sidelong backlift can be a critical benefit in global Test level cricket. Yet, the batting style is generally not perceived as a component of standard preparation. Players at cricket institutes are primarily taught the straight backlift, with others not putting a lot of consideration into the backlift.
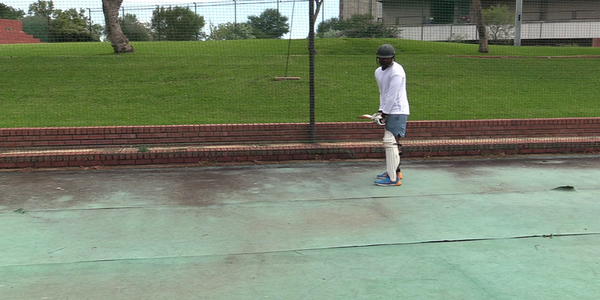
Upper hand
“What we find is that a sidelong backlift furnishes a hitter with a more ideal position while confronting the ball.” especially with quick bowlers. It gives the player an additional opportunity to get behind the ball, “says Noorbhai.
Furthermore, the sidelong backlift enjoys one more essential benefit.
“A cricket field is round, so we utilize a ‘cart wheel’ to demonstrate in which regions players score runs. At the point when hitters have a straight backlift, they generally just score in specific regions. This has been demonstrated. It will be more towards the straight side of the cart wheel, called the ‘V,’ “adds Noorbhai.
“At the point when they have a horizontal backlift, they score an additional region inside the cart wheel.”
UJ specialist Tevin Moodley shows how a sidelong cricket backlift varies from a straight backlift. Moodley is co-creator of the examination distributed in Scientific Reports: UJ analysts fabricated the principal profound learning AI model to recognize which backlift style cricket hitters use—straight or parallel. It mechanizes the most common way of examining players’ batting styles, utilizing video as it were. The best cricket batsman, Sir Donald Bradman, exhibited a parallel backlift method. Photographer: Therese van Wyk, University of Johannesburg
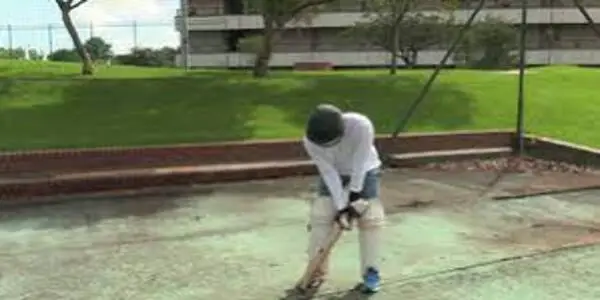
Tracking down hitters for the AI
In the first place, Moodley needed to track down video footage for their AI to gain from, since no open datasets were accessible. He sifted through web-based video films of first-class international cricket tests. He used the years 1995 to 2021.
From that point, he chose 10 players with a straight backlift: Babar Azam, Themba Bavuma, Rahul Dravid, JP Duminy, Dean Elgar, Mahela Jayawardene, Ajinkya Rahane, Joe Root, Rory Burns, Ben Stokes, and David Warner.
Furthermore, one more 10 with a parallel backlift: AB de Villiers, Hashim Amla, Quintin de Kock, Faf du Plessis, Kevin Pieterson, Kumar Sangakara, Brian Lara, Ricky Ponting, Steve Smith, and Virat Koli.
Altogether, Moodley physically chose 160 edges (pictures) from the video cuts as the AI’s “instructional course.” He chose one more forty as the “testing class.”
Simulated intelligence advances without anyone else.
“The excellence of profound learning in AI is that you don’t need to advise the AI what to search for,” says Moodley. “A mentor would have to zero in on where the bat’s face is, the point of the bat, where the player’s feet and head are, etc.”
The brain network in a profound learning AI can advance without anyone else, sorting out what elements are engaged with each backlift style. It is necessary to see great batting pictures for both the straight and parallel backlift from a decent dataset, obviously. In the event that there is more information, it can show itself better. It doesn’t need to know what a variable is called, such as downswing or backlift plot, for instance.
Parallel versus straight:
Next, Moodley ran his profound learning model on four different AI structures: AlexNet, Inception V3, Inception Resnet V2, and Xception. Could the more seasoned AlexNet best the fresher Xception or not? This was achieved on a 7-year-old i7 processor PC.
Yet, 160 pictures is next to no information for an AI to gain from. It is a genuinely mind-boggling endeavor to recognize the straight and parallel backlift.
According to a mentor’s point of view, they would initially check out at the point of the bat. Is the bat’s face calculated towards the subsequent slip? “
Prof. Dustin van der Haar makes sense of how AI PC vision can dissect video of competitors in various sports. Van der Haar played cricket at school. He utilizes PC vision procedures to break down competitor execution and clinical imaging. He is an example acknowledgment specialist at the Academy of Computer Science and Software Engineering (ACSSE), University of Johannesburg. He is the related creator of the examination distributed in Scientific Reports. UJ analysts fabricated the primary profound learning AI model to detect which backlift style cricket players use-straight or sidelong. It mechanizes the most common way of investigating players’ batting styles, utilizing video as it were. The best cricket batsman, Sir Donald Bradman, was a horizontal backlift player. Photographer: Therese van Wyk, University of Johannesburg
Or, on the other hand, is the bat faced towards the stumps or the ground — for a straight backlift? Inside, sidelong and straight, there are further varieties also. There are additionally different things that influence it, similar to hand position and how high you raise your bat, “says Moodley.
In any event, a human mentor might require a very long time to precisely differentiate, in any event, when supported by video investigation programming.
Preparing for AI
This is where Moodley and Van der Haar utilized “move figuring out how” to make up for the small dataset.
“Profound learning strategies require critical measures of information to perform well,” says Van der Haar. “Assuming that we prepared the model without any preparation, without utilizing an all-around prepared brain organization, it wouldn’t be exceptionally precise.” There would be too little information. “
“With machine learning, you pre-train a brain network with additional overall information as a beginning stage.” Then, at that point, you train it further, utilizing more unambiguous information, for example, horizontal and straight backlifts, “he adds.
They pre-prepared the model with machine learning so it would advance well from just 160 pictures, and then perform well in a test with 40 pictures.
Inception vs Xception:
The model utilizing Xception AI engineering emerged as the most reliable. It could accurately recognize players with a horizontal backlift procedure from those who would generally play with a straight backlift 98.2% of the time. Close behind it was Inception Resnet V2 with an accuracy of 96.1%.
“The Xception engineering takes every video outline and forcefully applies arrangements of square changes in a brain organization to assist with isolating the sidelong and straight backlift classifications,” says Van der Haar.
When the model is prepared, it conveys’start to finish’ acknowledgment among straight and horizontal lines, says Moodley. With the press of one button on the PC, the whole cycle is finished.
“There are no various little advances. “It resembles what we share with the AI: ‘Here are the pictures, go learn, done,'” he adds.
Prof. Habib Noorbhai finished his doctoral dissertation on the backlift in cricket and was among the first researchers to hypothesize the sidelong batting backlift method in cricket. Prof. Noorbhai is an Associate Professor and Director of the Biomedical Engineering and Healthcare Technology (BEAHT) Research Center at the University of Johannesburg (UJ). He is additionally a games scientist and has worked with nearby and global cricket crews since 2010. He is the co-creator of the exploration distributed in Scientific Reports: UJ specialists assembled the principal profound learning AI model to detect which backlift style cricket players use-straight or sidelong. It automatizes the most common way of investigating players’ batting styles, utilizing video as it were. The greatest cricket batsman ever, Sir Donald Bradman, was a parallel backlift player. Photographer: Therese van Wyk, University of Johannesburg
Grass identification using arms and legs
A mentor, dissecting video film to all the more likely help a player, needs to manage a ton of “clamor,” says Van der Haar. There are different players on the field, and different items. So an AI must be great at perceiving stuff applicable to batting strategy in the video outline.
Assuming the AI model just glances at the surface in the video casing to recognize grass and a player, it will come up short a great deal of the time. The same is true for AI searching for advances in light and dull — the examination would be extremely incorrect.
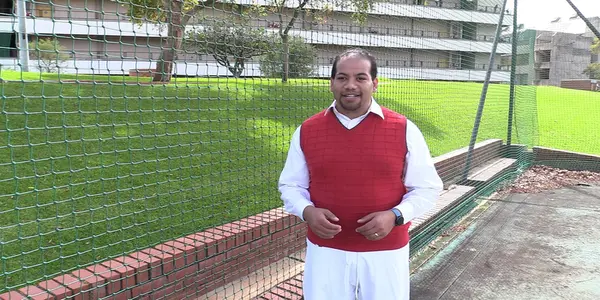
“What makes profound realizing AI so incredible is that it doesn’t stress over all that. “It sorts out whether or not a region of the video outline is significant or not,” says Van der Haar.
The brain network utilized for this AI model has a few layers, he says. The primary layer sees low-level stuff like surfaces or changes from light to dull, called slopes.
Each higher level layer of the brain net looks at something that is more similar to what we would see.At the most elevated level, the brain net is seeing things like arms and legs and bat points.
When the brain net sorts out the various layers in a video outline, it can then say, “This is a horizontal or straight backlift,” says Van der Haar.
Instructing knowledge from AI:
Traditionally, cricket trainers depend on examination apparatuses to see what their players are doing. It is a three-or four-venture process. They need to take a camera and record the players. They then, at that point, send out that recording to investigation programming. Then, at that point, they break down the recording and give proposals to the players, says Noorbhai.
“What profoundly realizing AI does is a type of computerization where the four stages are consolidated into one. All they would then need to do in the future is take that video film and utilize the profound learning AI as a type of computerizing how the situation is playing out, “he adds.
“I don’t think this exploration is tied in with breaking down additional players. I think it is tied in with furnishing mentors and players with more understanding than they are as of now getting, “says Noorbhai.
Prof. Habib Noorbhai finished his doctoral proposition on the backlift in cricket and was among the first scientists to conjecture the parallel batting backlift procedure in cricket. Prof. Noorbhai is an associate professor and director of the Biomedical Engineering and Healthcare Technology (BEAHT) Research Centre at the University of Johannesburg (UJ). He is additionally a game researcher and has worked with neighborhood and international cricket crews since 2010. He is co-creator of an examination distributed in Nature Scientific Reports: UJ analysts fabricated the primary profound learning AI model to recognize which backlift style cricket hitters use—straight or horizontal. It automatizes the most common way of examining players’ batting styles, utilizing video as it were. The greatest cricket batsman ever, Sir Donald Bradman, was a parallel backlift player. University of Johannesburg is to blame.
Undeveloped players utilize horizontal
Undeveloped players frequently instinctually utilize a horizontal backlift, says Noorbhai.
“What we have found is that in the event that youthful players are not instructed utilizing conventional strategies, they don’t get the bat straight. They get the bat on a parallel course. This, in a roundabout way, suggests that a straight backlift is anything but a characteristic development. “
Changing from direct to parallel
At the opposite end of the range, a few profoundly fruitful players utilize both a straight and a horizontal backlift.
“At the actual beginning of a player’s innings, we observe that they have a greater amount of straight backlift.” Why? “Since they must be somewhat more cautious in their methodology, so they can become accustomed to the pitch, the bowlers, and the circumstances,” says Noorbhai.
“When they foster trust in their innings, they begin to open up, and then their backlift heads more in a parallel path with the open face of the bat.”
Moodley, Van der Haar, and Noorbhai’s exploration shows the way that it is as of now conceivable to robotize some cricket instructing. Their model can assist mentors with promising and laid-out cricket players, utilizing video alone.
The incredible Bradman’s backlift was known as a “circle backlift” or “rotational batting procedure” in his time. Today we remember it as a horizontal backlift, as experimentally guessed by Noorbhai and Noakes in 2016. The style is utilized instinctually by undeveloped players—and decisively or innately by effective experts.