Utilizing registered assets at the National Energy Research Scientific Computing Center (NERSC) at Lawrence Berkeley National Laboratory (Berkeley Lab), analysts at Argonne National Laboratory have prevailed with regards to investigating significant materials science questions and have exhibited progress utilizing AI to take care of troublesome inquiry issues.
By adjusting an AI calculation from prepackaged games, for example, AlphaGo, the scientists created force fields for nanoclusters of 54 components across the intermittent table, a sensational jump toward grasping their one-of-a-kind properties and verification of ideas for their inquiry strategy. The group published its outcomes in Nature Communications in January.
Contingent upon their scale—mass frameworks of 100+ nanometers versus nanoclusters of under 100 nanometers—materials can show various properties, including optical and attractive properties, discrete energy levels, and upgraded photoluminescence. These properties might lend themselves to new logical and industrial applications, and researchers can find out about them by creating force fields—computational models that gauge the possible energies between iotas in a particle and between atoms—for every component or compound. In any case, materials researchers can spend years investigating the designs and properties of iotas in nanoclusters of a single component using traditional physical science-based approaches.
“We wanted to look at nanoscale dynamics, and normally we’d use quantum calculus and density functional theory for that,” says the researcher. “However, those are computationally very expensive calculations.”
Scientist Sukriti Manna,
“We needed to take a gander at the nanoscale elements, and for that, typically we’d utilize a few quantum math and thickness useful hypotheses, yet those are computationally over the top expensive estimations,” said materials researcher Sukriti Manna, essential creator of the paper. of the meticulous work of looking for and tracking down the boundaries of possible models.
Applying AI is one possible method for reducing that expense. In any case, the accessible calculations come from discrete hunting spaces like games, where the quantity of search branches and potential results is limited. In a constant activity space like power fields for substance component nanoclusters, the quantity of conceivable pursuit branches is limitless, and savage power — the capacity to run each situation to track down the best result — just doesn’t work.
Working smarter, not harder
To make a current calculation maintain a flexible mindset, AI expert Troy Loeffler utilized a sort of support learning called Monte Carlo tree search (MCTS). Support learning is a type of AI that permits a calculation to connect straightforwardly with its current circumstance, learning through discipline and reward, fully intent on acquiring the most combined compensation after some time. MCTS utilizes an “investigate and take advantage of” technique—at first looking arbitrarily, then, at that point, figuring out how to disregard less useful pursuit ways, or playouts, and center around additional useful ones. Loeffler also added a couple of new capacities to make the calculation more effective: a uniqueness capacity to eliminate repetitive pursuits; a window scaling plan to correspond the tree profundity to the activity space to give a valuable piece of design; and playout extension, which assists the calculation in focusing on irregular quests that were closer to something that had previously proven useful.
“A ton of the work we did was really fostering the calculation for consistent activity spaces, where you don’t have decent, discrete prepackaged game spaces; you have boundaries that can move anyplace in the specific scene,” said Loeffler. “The center thought is that you’re utilizing a mix of both complete haphazardness and somewhat of a deterministic component, with the AI, to sort it out.”
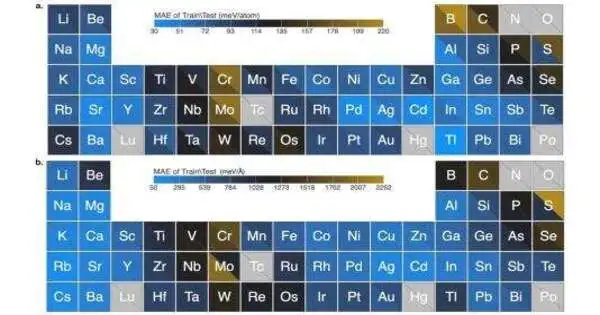
Credit: NERSC
The blend worked, yielding power fields for 54 components in a small part of the time it once would have taken to find boundaries for only one component and demonstrating that support learning can be a helpful device in consistent activity spaces.
The group utilized the Cori supercomputer at NERSC to play out their computations and produce both preparation and fitting datasets, basically utilizing Vienna Ab initio Simulation Package (VASP) programming for nuclear scale materials demonstration and the old style sub-atomic elements code LAMMPS. This task is only one of numerous at NERSC from the Theory and Modeling group at Argonne, who regularly exploit NERSC’s computational power, insignificant lines, and solid support.
For components like carbon, boron, and phosphorous, we require a ton of datasets and we require great quality. For this specific work, I use NERSC for producing lots of enormous datasets as a result of their primary variety. “Cori is an extremely quick PC, and when I was utilizing it, the line time was exceptionally short, so we finished that work rapidly,” said Manna. Furthermore, he said, “Assuming we have 100 percent responsibility for computational time, we rely upon NERSC for 90% of that responsibility.”
AI expert Rohit Batra, who fostered an AI structure to examine the blunder patterns in likely capacities across the occasional table, agreed. “I seriously love Cori—I use it for a few purposes,” he said. “It’s all around us that we’re keeping up with.”In some cases, in different groups, there can be issues that make them disconnected for a surprisingly long time. I think NERSC is very much kept up with and truly solid in that. “
The eventual fate of MCTS dives deep and wide.
Now that the utilization of MCTS in nonstop pursuit space has been illustrated, what comes next? From a materials science viewpoint, there’s more work to be done investigating more perplexing materials.
“According to an application point of view and a power field improvement point of view, we’ve investigated essential stuff and a couple of parallel composites, so soon we’ll investigate mixes, similar to oxides and sulfites, and foster those power fields,” said Manna. “In view of the strong calculation, all we really want is time and other preparation informational collections.”
Yet, materials science isn’t the main use of MCTS torn open by this work — and some portion of the following stage includes testing the broadness and limits of the calculation’s utility.
“We’re taking MCTS and applying it to many circumstances,” said Loeffler. “We have 10 or 11 distinct tasks that we or our colleagues are keen on involving the calculation for,” including further games-arranged research and extra power field fitting. He added that interaction has met with progress, and its future looks splendid. “We’re searching for a great deal of things to give it a shot. In any case, up to this point, all that we’ve given it a shot, it’s functioned admirably. “