Specialists at Seoul Public College have as of late attempted to prepare a man-made brainpower (simulated intelligence) specialist to make collections (i.e., fine arts made by sticking different bits of materials together), imitating prestigious craftsmanship and different pictures. Their proposed model was presented in a paper pre-imprinted on arXiv and introduced at ICCV 2023 in October.
“Arrangement craftsmanship requires high human imaginativeness, and we considered what montage works of art made by simulated intelligence would seem to be,” the writers told Tech Xplore by email. “Existing artificial intelligence picture-age devices like DALL-E or StableDiffusion can as of now produce collection pictures; however, they are simply ‘composition impersonations’ from pixels, not the genuine composition from completing the genuine strides of montage fine art. What we needed to do was prepare simulated intelligence to make a ‘genuine arrangement’.”
In a past report zeroing in on the painting age, scientists utilized support learning (RL) to help artificial intelligence paint following advances like those followed by people. They then, at that point, began contemplating whether this could likewise be accomplished for the production of collections and began chipping away at their support learning-based independent montage craftsmanship generator.
The essential goal of their new paper was subsequently to prepare a computer-based intelligence specialist to make montages that are as objective as possible (e.g., canvases, photos, and so forth) by tearing and gluing various materials, utilizing support learning. These compositions would be made utilizing a bunch of materials given by human clients.
“Our RL model requirements are to cause a specialist to comprehend what a collection is and how to do it competently,” the creators made sense of. “As RL fundamentally requires numerous preliminaries and mistakes, the model needs to acquire experience connecting with a material and delivering a genuine montage.”
As compositions are made of different pieces of material, a specialist first needs to test different reorder choices to eventually figure out which materials produce a collection that best looks like objective pictures. The scientists found that at first, their model performed inadequately, yet over the long run, its abilities fundamentally climbed to the next level.
“The RL specialist figures out how to make the prize greater, where the award is characterized as an improvement in the closeness between their material and an objective picture,” the creators said. “The prize capability additionally continues to advance over the long run, figuring out how to more readily assess the likeness between a specialist-made arrangement and the objective picture.”
During preparation, the specialists’ model took care of a haphazardly relegated irregular picture and attempted to make a composition imitating this picture on white material. At each step of the collection, the specialist chooses an irregular material among accessible choices and picks how to cut it, scrap it, and glue it onto the material.
“Since the objective pictures and materials are haphazardly given in preparation, the specialist becomes ready to manage any objectives and materials at a later stage,” the writers said. “This entire cycle is a piece convoluted for utilizing existing without model RL, so we fostered a differentiable collaging climate to permit the specialist to effectively follow the collection’s elements. This permitted us to apply model-based RL and improve execution.”
The model-based RL preparation plan created by the analysts draws motivation from past work about RL-based canvases. Nonetheless, the group fostered their own model-based RL calculation that tended to the elements related to making montages, which are more complicated than those supporting canvas.
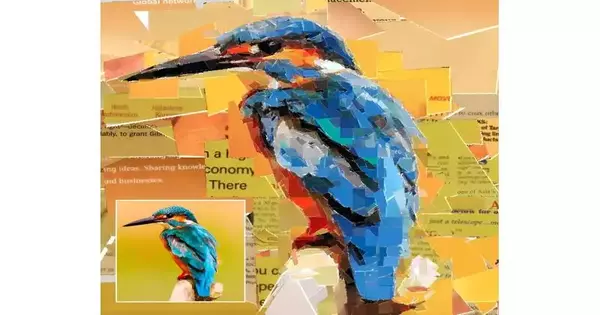
The “Bird” made of papers, target picture from pixabay.com/photographs/kingfisher-bird-close-up-roosted 2046453. Credit: Dai et al.
“While painting utilizes a predefined brushstroke, a composition needs to see how the given material looks and sort out some way to control it to make a legitimate picture part for the complete montage, grasping shape, surface, varieties, and directions,” the creators said. “Since SAC permits a specialist to encounter assorted activities more really in the constant activity space than DDPG, which was utilized in artistic creations, SAC matches our case.”
To successfully create montages, the creators involved their prepared model as an incomplete collection generator unit. This unit was found to create high-goal arrangements that firmly looked like different objective pictures.
“We likewise fostered a module for breaking down target picture intricacy to relegate more responsibility for the halfway montage generator to the spot where the intricacy is high,” Lee made sense of. “This module can improve the stylish nature of compositions.”
A vital benefit of the group’s engineering is that it requires no composition tests or showing information, as it was basically prepared utilizing instances of materials and target pictures. Indeed, these materials and pictures are far more straightforward to gather than unique craftsmanship.
“Without imaginative information, the specialist freely figured out how to make a montage,” the creators said. “The last collaging skill was made by the specialist’s own investigation, which is the remarkable finding of this work; it shows the powerful capacity of RL as an information-free learning space.”
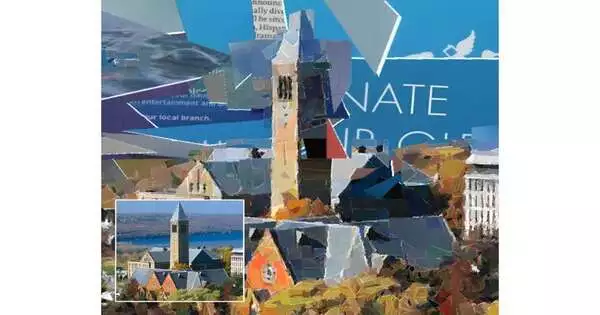
The “congregation” was made of papers. Credit: Dai et al.
As the group’s prepared model bit by bit got a handle on the course of composition-making, it could sum up well across a large number of pictures and situations. Up to this point, it has just been tried in reproductions. Notwithstanding, whenever applied to a humanoid robot or a mechanical hand, the model could likewise give ‘plans’ to the production of actual compositions.
“Building a climate in which the RL specialist can advance appropriately was exceptionally difficult,” the creators said. “We invested a ton of energy in creating and characterizing composition elements and activities that are genuine for RL. Likewise, to save preparation time, we ought to keep them as minimal and effective as could really be expected. Significantly more, we needed to keep the elements differentiable for our model-based RL plot too.”
As workmanship is profoundly abstract, assessing the nature of arrangements created by the model is testing. The specialists at first did a client study, requesting that different human members share their perspectives and criticisms of the simulated intelligence-made compositions.
“We led a client study; however, this may not be sufficient,” the creators said. “After much thought for a more genuine assessment, we chose to utilize Clasp, an enormous vision-language pre-prepared model. Since Clasp is prepared with around 400 million text-picture matches, we accept it can be assessed more impartially than a client study. With client study and Clasp, we contrasted our model and other pixel-based age models by assessing the produced pictures’ arrangement and content consistency.”
The client study and the cluster-based assessment done by the scientists yielded comparable outcomes. In both of these tests, the new model was found to beat different models for arrangement age.

The “young lady with a pearl hoop” was made of papers. Credit: Dai et al.
The model presented in this new paper could before long be grown further and tried to permit tweaked styles utilizing a more extensive scope of pictures and materials. Also, the cooperation could advance the advancement of extra man-made intelligence apparatuses for producing different kinds of craftsmanship.
“We are currently keen on creating procedures that permit our models to adapt to different style inclinations,” the creators added. “As a future work, we consider fostering a client’s intelligent point of interaction, which can mirror the client’s inclination during our model’s making montages.”
More information: Ganghun Lee et al, Neural Collage Transfer: Artistic Reconstruction via Material Manipulation, arXiv (2023). DOI: 10.48550/arxiv.2311.02202
Ganghun Lee et al. From Scratch to Sketch: Deep Decoupled Hierarchical Reinforcement Learning for Robotic Sketching Agent, 2022 International Conference on Robotics and Automation (ICRA) (2022) DOI: 10.1109/ICRA46639.2022.9811858