Researchers collaborated to create a machine-learning program that can be linked to a human brain and used to control a robot. Two EPFL research teams collaborated to create a machine-learning program that can be linked to a human brain and used to control a robot. Based on electrical signals from the brain, the program adjusts the robot’s movements. The hope is that tetraplegic patients will be able to do more day-to-day activities on their own as a result of this invention.
Tetraplegic patients are prisoners of their own bodies, unable to speak or move even the smallest of movements. For years, researchers have been working to develop systems that can assist these patients in performing some tasks on their own. “People with spinal cord injuries frequently experience permanent neurological deficits and severe motor disabilities that prevent them from performing even the most basic tasks, such as grasping an object,” explains Prof. Aude Billard, head of EPFL’s Learning Algorithms and Systems Laboratory. “Robot assistance could help these people regain some of their lost dexterity, because the robot can execute tasks in their place.”
Prof. Billard collaborated with Prof. José del R. Millán, who was the head of EPFL’s Brain-Machine Interface laboratory at the time but has since moved to the University of Texas. The two research teams collaborated to create a computer program that can control a robot using electrical signals emitted by a patient’s brain. There is no need for voice or touch control; patients can move the robot simply by thinking about it. The findings were published in Communications Biology, an open-access journal published by Nature Portfolio.
The robot’s AI program can learn quickly, but you have to tell it when it makes a mistake so that it can correct its behavior. One of the most difficult technical challenges we faced was developing detection technology for error signals.
Iason Batzianoulis
Avoiding obstacles
The researchers began developing their system with a robotic arm that had been developed several years before. This arm can move from right to left, reposition objects in front of it, and maneuver around obstacles in its path. “In our study, we programmed a robot to avoid obstacles, but we could have chosen any other task, such as filling a glass of water or pushing or pulling an object,” Prof. Billard explains.
The engineers began by fine-tuning the robot’s obstacle-avoidance mechanism to make it more precise. “At first, the robot would choose a path that was too wide for some obstacles, taking it too far away, and not wide enough for others, keeping it too close,” Carolina Gaspar Pinto Ramos Correia, a Ph.D. student in Prof. Billard’s lab, explains. “Because our robot’s goal was to assist paralyzed patients, we needed to find a way for users to communicate with it that didn’t require speaking or moving.”
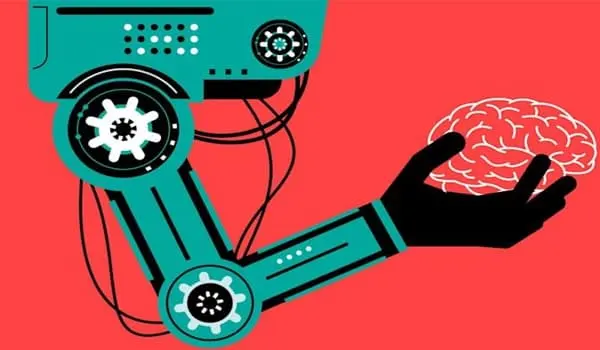
An algorithm that can learn from thoughts
This entailed creating an algorithm capable of adjusting the robot’s movements solely based on a patient’s thoughts. The algorithm was linked to a head cap outfitted with electrodes, which was used to conduct electroencephalogram (EEG) scans of a patient’s brain activity. All the patient has to do to use the system is look at the robot. If the robot makes an incorrect move, the patient’s brain will send an “error message” via a distinct signal, as if the patient is saying, “No, not like that.”
The robot will then realize that what it is doing is incorrect, but it will not know why at first. Did it, for example, get too close to or too far away from the object? The error message is fed into the algorithm, which uses an inverse reinforcement learning approach to figure out what the patient wants and what actions the robot needs to take. This is accomplished through a trial-and-error process in which the robot tries out various movements to determine which one is correct. The process is relatively quick; usually, only three to five attempts are required for the robot to figure out the correct response and carry out the patient’s wishes.
“The robot’s AI program can learn quickly,” Prof. Millán says, “but you have to tell it when it makes a mistake so that it can correct its behavior.” “One of the most difficult technical challenges we faced was developing detection technology for error signals.” The study’s lead author, Iason Batzianoulis, adds: “In our study, the most difficult part was connecting a patient’s brain activity to the robot’s control system – or, in other words, ‘translating’ a patient’s brain signals into robot actions. We accomplished this by using machine learning to associate a specific brain signal with a specific task. The tasks were then linked to individual robot controls, allowing the robot to perform the task that the patient had in mind.”
Next step: a mind-controlled wheelchair
The researchers hope to use their algorithm to control wheelchairs in the future. “For the time being, there are still a lot of engineering hurdles to overcome,” Prof. Billard says. “Wheelchairs, on the other hand, presents an entirely new set of challenges, because both the patient and the robot are in motion.” The team also intends to apply their algorithm to a robot that can read various types of signals and coordinate data from the brain with those from visual-motor functions.