According to a new study from Oregon State University’s College of Engineering, machine learning techniques can provide powerful new tools for advancing personalized medicine, which is care that optimizes outcomes for individual patients based on unique aspects of their biology and disease features.
According to Oregon State’s Brian D. Wood, who conducted the study with then-OSU Ph.D. student Ehsan Taghizadeh and Helen M. Byrne of the University of Oxford, the research with machine learning, a branch of artificial intelligence in which computer systems use algorithms and statistical models to look for trends in data, addresses long-unsolvable problems in biological systems at the cellular level.
“Those systems tend to be complex,” said Wood, an environmental engineering professor. “First, because of the large number of individual cells, and second, because of the highly nonlinear way in which cells can behave. Nonlinear systems pose a challenge for upscaling methods, which are the primary means by which researchers can accurately model biological systems at larger scales, which are frequently the most relevant.”
Our research takes advantage of what are known as deep neural networks to upscale the nonlinear processes found in transport and reactions within tissues. The promises of personalized medicine are quickly becoming a reality. Multiple disciplines, including molecular biology, applied mathematics, and continuum mechanics, are being combined in novel ways to make this possible.
Brian D. Wood
In science or mathematics, a linear system is one in which any change in the system’s input results in a proportional change in the output; for example, a linear equation might describe a slope that gains 2 feet vertically for every foot of horizontal distance. Nonlinear systems do not operate in this manner, and many systems in the world, including biological ones, are nonlinear.
According to Wood, the new study, which was funded in part by the US Department of Energy and published in the Journal of Computational Physics, is one of the first examples of using machine learning to address issues with modeling nonlinear systems and understanding complex processes that may occur in human tissues.
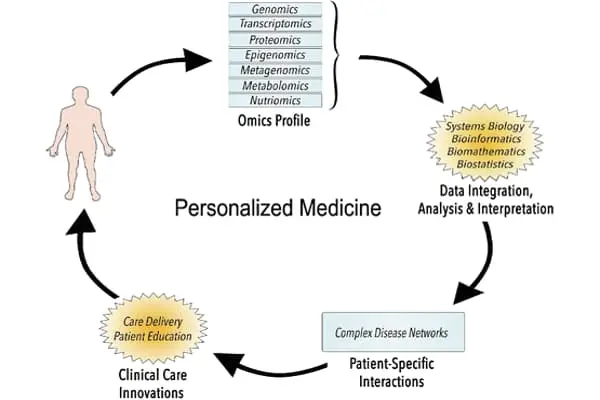
“With the advent of machine learning, we now have a new tool in our arsenal to solve problems that we could not solve before,” he explained. “While the tools themselves are not necessarily novel, the applications we have are quite distinct. We are beginning to apply machine learning in a more constrained manner, which is allowing us to solve physical problems that we previously could not solve.”
Because it is not possible to individually model each cell in an organ (a cubic centimeter of tissue may contain a billion cells), researchers must rely on what is known as up-scaling. up-scaling aims to reduce the amount of data needed to analyze or model a specific biological process while retaining the fidelity (the degree to which a model accurately reproduces something) of the core biology, chemistry, and physics occurring at the cellular level.
Wood observes that biological systems resist traditional up-scaling techniques, which is where machine learning methods come in. Researchers can better analyze and model the impact or response of those cells with high fidelity by reducing the information load for a very complicated system at the cellular level, rather than having to model each individual one. Wood describes it as “reducing a computational problem with tens of millions of data points to thousands of data points.”
The new method may pave the way for potential patient treatments based on numerical model results. Researchers were able to use machine learning and develop a novel method to solve classic nonlinear problems in biological and chemical systems in this study.
“Our research takes advantage of what are known as deep neural networks to upscale the nonlinear processes found in transport and reactions within tissues,” Wood explained. Wood is also involved in another research project that uses machine learning techniques to model blood flow in the body.
“The promises of personalized medicine are quickly becoming a reality,” he said. “Multiple disciplines, including molecular biology, applied mathematics, and continuum mechanics, are being combined in novel ways to make this possible. One of the most important aspects of this will undoubtedly be the ongoing advancements in machine learning methods.”