A worldwide group of specialists has planned and fabricated a chip that runs calculations straightforwardly in memory and can run a wide assortment of AI applications-all for a portion of the energy consumed by registering stages for broadly useful AI figures.
The NeuRRAM neuromorphic chip presents to AI a bit nearer to running on a wide scope of edge gadgets, separated from the cloud, where they can perform refined mental undertakings anyplace and whenever without depending on an organization association with a concentrated server. Applications have large amounts of each and every side of the world and each feature of our lives, and range from brilliant watches, to VR headsets, shrewd headphones, savvy sensors in manufacturing plants, and wanderers for space investigation.
The NeuRRAM chip isn’t just two times as energy productive as the cutting edge “process in-memory” chips, a creative class of mixture chips that run calculations in memory, it likewise conveys results that are similarly as precise as customary computerized chips. Ordinary AI stages are much bulkier and normally require enormous information servers working in the cloud.
Likewise, the NeuRRAM chip is profoundly flexible and upholds various brain network models and designs. Subsequently, the chip can be utilized for the majority of various applications, including picture acknowledgment and reproduction as well as voice acknowledgment.
“The usual notion is that better efficiency of compute-in-memory comes at the expense of versatility, but our NeuRRAM technology achieves efficiency without sacrificing adaptability,”
Weier Wan
“The customary way of thinking is that the higher proficiency of figure in-memory is at the expense of flexibility, yet our NeuRRAM chip gets effectiveness while not forfeiting flexibility,” said Weier Wan, the paper’s most memorable comparison creator and a new Ph.D. graduate of Stanford University who dealt with the chip while at UC San Diego, where he was co-prompted by Gert Cauwenberghs in the Department of Bioengineering.
The examination group, co-driven by bioengineers at the University of California, San Diego, presents their outcomes in the Aug. 17 issue of Nature.
Right now, AI registering is both power-hungry and computationally costly. Most AI applications for nervous gadgets include moving information from the gadgets to the cloud, where the AI processes and investigates it. Then the outcomes are moved back to the gadget. That is on the grounds that most edge gadgets are battery-controlled and, subsequently, just have a limited measure of force that can be devoted to processing.
This NeuRRAM chip could prompt more vigorous, astute, and open-edge gadgets and more astute assembling by lowering the power utilization required for AI deduction at the edge.It may also result in improved information security, as the transfer of data from devices to the cloud entails increased security risks.
On AI chips, moving information from memory to processing units is one significant bottleneck.
“It’s what could be compared to doing an eight-hour drive for a two-hour work day,” Wan said.
To address this data transfer issue, experts used resistive irregular access memory, a type of non-unstable memory that considers calculation directly inside memory rather than in separate calculating units.RRAM and other arising memory advancements utilized as neurotransmitter clusters for neuromorphic figuring were spearheaded in the lab of Philip Wong, Wan’s counsel at Stanford and a primary supporter of this work. Calculation with RRAM chips isn’t really new, yet by and large it prompts a decline in the exactness of the calculations performed on the chip and an absence of adaptability in the chip’s engineering.
“Register in-memory has been normal practice in neuromorphic design since it was presented over quite a while back,” Cauwenberghs said. “What’s happening with NeuRRAM is that the outrageous proficiency presently goes along with extraordinary adaptability for different AI applications with practically no misfortune in exactness over standard advanced universally useful figure stages.”
A painstakingly created procedure was vital to the work, with different degrees of “co-streamlining” across the deliberation layers of equipment and programming, from the plan of the chip to its setup to run different AI errands. The group made a point of representing different requirements that range from memory gadget physical science to circuits and organizational design.
“This chip presently gives us a stage to resolve these issues across the stack, from gadgets and circuits to calculations,” said Siddharth Joshi, an associate professor of software engineering and design at the University of Notre Dame, who began dealing with the task as a Ph.D. understudy and postdoctoral scientist in the Cauwenberghs lab at UC San Diego.
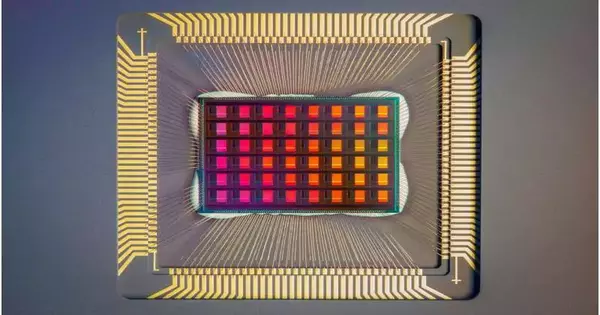
A close up of the NeuRRAM chip.
Chip performance
Scientists estimated the chip’s energy effectiveness by an action known as energy-postponed item, or EDP. EDP consolidates both how much energy is consumed for each activity and how much time it takes to finish the activity. By this action, the NeuRRAM chip accomplishes 1.6 to 2.3 times lower EDP (lower is better) and 7 to multiple times higher computational thickness than cutting-edge chips.
Analysts ran different AI undertakings on the chip. It accomplished close to 100% exactness on a written by-hand digit acknowledgment task; 85.7% on a picture characterization task; and 84.7% on a Google discourse order acknowledgment task. Moreover, the chip likewise accomplished a 70% decrease in picture reproduction mistakes on a picture recuperation task. These outcomes are equivalent to existing computerized chips that perform calculations with a similar level of accuracy, but with radical reserve funds in energy.
Analysts call attention to that one critical commitment of the paper is that every one of the outcomes included is acquired straightforwardly on the equipment. AI benchmark results were frequently obtained somewhat by programming recreation in numerous previous works of registered in-memory chips.
Subsequent stages incorporate further developing models and circuits and scaling the plan to further develop innovation hubs. Scientists similarly plan to handle different applications, for example, spiking brain organizations.
“We can improve at the gadget level, further develop circuit plans to carry out extra elements and address different applications with our dynamic NeuRRAM stage,” said Rajkumar Kubendran, an associate teacher for the University of Pittsburgh, who began work on the undertaking while a Ph.D. understudy in Cauwenberghs’ exploration group at UC San Diego.
Furthermore, Wan is a well-known individual from a startup that specializes in productizing register in-memory innovation.”As a scientist and a specialist, my desire is to bring research developments from labs into practical use,” Wan said.
New engineering
The key to NeuRRAM’s energy effectiveness is an imaginative technique to detect yield in memory. Ordinary methodologies use voltage as information and measure current as the outcome. Be that as it may, this prompts the requirement for more intricate and power-hungry circuits. In NeuRRAM, the group designed a neuron circuit that detects voltage and performs simple to-computerized changes in an energy-effective way. This voltage-mode detecting can execute every one of the lines and every one of the segments of a RRAM cluster in a solitary processing cycle, permitting higher parallelism.
In the NeuRRAM architecture, CMOS neuron circuits are actually interleaved with RRAM loads. It contrasts from regular plans where CMOS circuits are normally on the fringe of RRAM weights. The neuron’s associations with the RRAM exhibit can be arranged to act as one or the other information or result of the neuron. This permits brain network derivation in different information stream headings without causing overheads in region or power utilization. This thus makes the design simpler to reconfigure.
To ensure that the precision of the AI calculations can be protected across different brain network structures, scientists have fostered a bunch of equipment calculation co-enhancement strategies. The methods were confirmed on different brain networks, including convolutional brain organizations, long transient memory, and limited Boltzmann machines.
As a neuromorphic AI chip, NeuroRRAM performs equal conveyed handling across 48 neurosynaptic centers. At the same time, to accomplish high flexibility and high productivity, NeuRRAM upholds information parallelism by planning a layer in the brain network model onto various centers for equal derivation of numerous pieces of information. Likewise, NeuRRAM offers model-parallelism by planning various layers of a model onto various centers and performing deduction in a pipelined style.
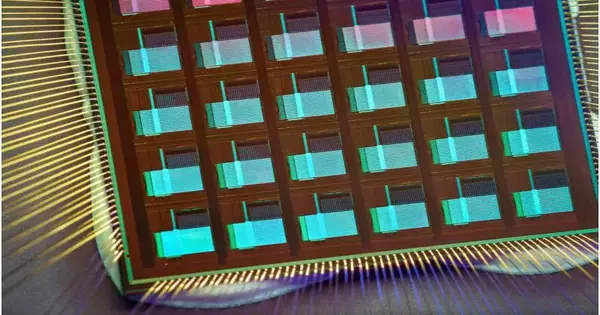
The NeuRRAM chip utilizes creative engineering that has been co-improved across the stack.
A worldwide examination group
The work is the aftereffect of a global group of scientists.
The UC San Diego group planned the CMOS circuits that carry out the brain capabilities communicating with the RRAM clusters to help the synaptic capabilities in the chip’s engineering for high proficiency and flexibility. Working intimately with the whole group, he carried out the plan; described the chip; prepared the AI models; and executed the tests. Wan likewise fostered a product toolchain that maps AI applications onto the chip.
The RRAM neurotransmitter cluster and its working circumstances were broadly portrayed and upgraded at Stanford University.
The RRAM cluster was created and incorporated into CMOS at Tsinghua University.
The team at Notre Dame added to both the plan and engineering of the chip and the ensuing AI model plan and preparation.
More information: Weier Wan, A compute-in-memory chip based on resistive random-access memory, Nature (2022). DOI: 10.1038/s41586-022-04992-8. www.nature.com/articles/s41586-022-04992-8
Journal information: Nature