An exploration group led by Teacher Hwangbo Jemin of the KAIST Branch of Mechanical Design has fostered a quadrupedal robot control innovation that can walk heartily with deftness even in deformable landscapes like a sandy oceanside.
Teacher Hwangbo’s examination group fostered an innovation to demonstrate the power gained by a mobile robot on granular ground, such as sand, and mimic it with a quadrupedal robot.Likewise, the group chipped away at a fake brain network structure that was fit for pursuing ongoing choices to adjust to different kinds of ground surfaces without earlier data while strolling simultaneously and applied it to support learning.
The prepared brain network regulator is supposed to grow the extent of quadrupedal strolling robots by demonstrating its vigor in evolving territory, including the capacity to move fast even on a sandy beach and walk and turn on delicate grounds like a pneumatic bed without losing balance.
“It has been demonstrated that giving a learning-based controller with close contact experience with genuine deforming terrain is critical for applicability to deforming terrain. Because the proposed controller can be employed without prior knowledge of the terrain, it can be used to a variety of robot walking investigations.”
First author Suyoung Choi
This exploration, with Ph.D. Understudy Soo-Young Choi of the KAIST Branch of Mechanical Designing as the main creator, was distributed in January in Science Advanced Mechanics under the title “Learning quadrupedal motion on deformable territory.”
The exhibition of the newly created regulator on a delicate pneumatic bed.
Support learning is a man-made intelligence learning strategy used to make a machine that gathers information on the consequences of different activities in an erratic circumstance and uses that arrangement of information to play out an errand. Since the amount of information expected to support learning is so huge, a strategy for gathering information through recreations that approximate the actual peculiarities of the genuine climate is widely utilized.
Learning-based regulators in the field of strolling robots, in particular, have been applied to genuine conditions following learning through information gathered in recreations to perform strolling controls in various landscapes effectively.
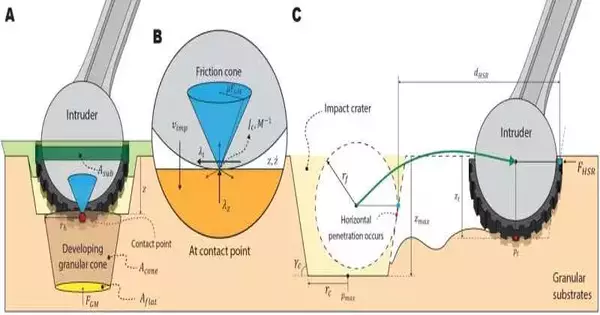
Contact model definition (A) Based on the gatecrasher’s entrance profundity and speed, the landscape model predicts an increase in the ashore response force. The calculation involves creating a granular cone underneath the interloper, as Aguilar et al. (27) proposed. (B) The surface contact between the interloper and the nearby substrates is approximated as a point contact at the most profound point. The mass of unrelated power from the substrates is accepted to be Coulomb contact. (C) The flat-stroke resistive power model is familiar with simulating the response from the substrates when the gatecrasher moves evenly in the substrates. The force is calculated using the movement distance dHSR and the continuous entrance profundity zt.
However, because the display of the learning-based regulator rapidly diminishes when the genuine climate contains any errors from the learned recreation climate, it is critical to execute a climate similar to the genuine one in the information gathering stage. Hence, to make a learning-based regulator that can keep up with balance in a twisting territory, the test system should give a comparable insight into contact.
The exploration group characterized a contact model that anticipated the power created upon contact from the movement elements of a mobile body in view of a ground response force model that considered the extra mass impact of granular media characterized in past examinations.
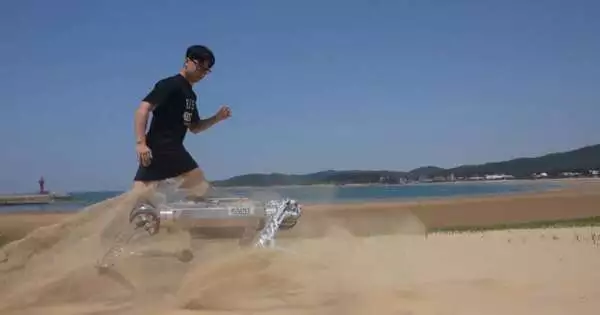
RaiBo on an oceanside run The Korea Progressed Foundation of Science and Innovation (KAIST) is responsible for this image.
Besides, by computing the power created from one or a few contacts at each time step, the twisting landscape was effectively mimicked.
The exploration group likewise presented a fake brain network structure that certainly predicts ground qualities by utilizing a repetitive brain network that dissects time-series information from the robot’s sensors.
The learned regulator was mounted on the robot RaiBo, which was used by the exploration team to demonstrate fast walking of up to 3.03 meters per second on a sandy oceanside with the robot’s feet completely lowered in the sand.In any event, when applied to more earnest grounds, for example, lush fields and a running track, RaiBo had the option to run steadily by adjusting to the qualities of the ground with no extra programming or update to the controlling calculation.
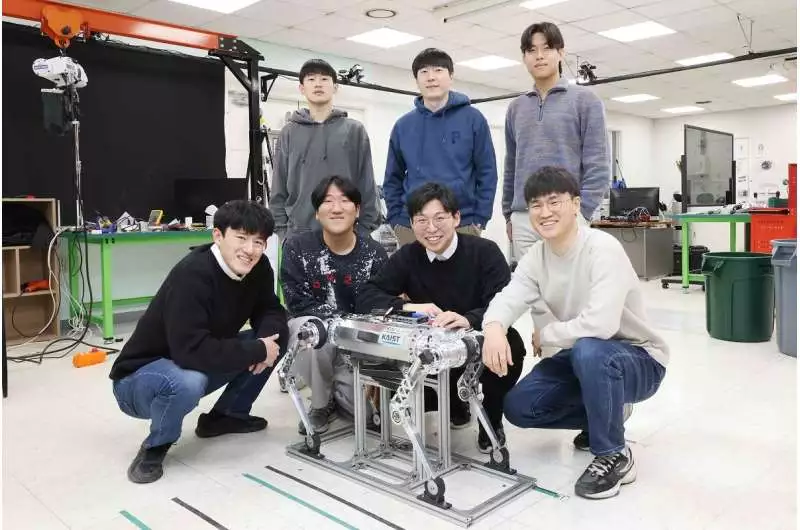
RAI Lab Group in the background, with Teacher Hwangbo.
Also, it pivoted with dependability at 1.54 rad/s (around 90° each second) on a pneumatic bed and exhibited this fast flexibility even in the circumstances in which the landscape abruptly turned delicate.
The research team demonstrated the importance of providing a reasonable contact insight during the growing experience by examining a regulator that expected the ground to be unbending and demonstrating that the proposed repetitive brain network changes the regulator’s strolling strategy based on the ground properties.
The recreation and learning system created by the examination group is supposed to add to robots performing viable errands as it grows the scope of landscapes that different strolling robots can work on.
First creator Suyoung Choi said, “It has been shown that furnishing a learning-based regulator with a nearby contact insight with genuine twisting ground is fundamental for application to misshaping territory.” He proceeded to add that “the proposed regulator can be utilized without earlier data on the landscape, so it tends to be applied to different robot strolling studies.”
More information: Suyoung Choi et al, Learning quadrupedal locomotion on deformable terrain, Science Robotics (2023). DOI: 10.1126/scirobotics.ade2256
Journal information: Science Robotics