Analysts at Children’s Hospital of Philadelphia (CHOP) have fostered another device to assist scientists with interpreting the clinical meaning of substantial transformations in disease. The device, known as CancerVar, integrates AI structures to go beyond just recognizing physical disease changes and deciphering the expected meaning of those transformations concerning malignant growth finding, visualization, and targetability. A paper depicting CancerVar was distributed today in Science Advances.
“CancerVar will not replace human interpretation in a clinical setting, but it will significantly reduce human reviewers’ manual work in classifying variants discovered through sequencing and drafting clinical reports in the practice of precision oncology,” says the company.
Kai Wang, Ph.D., Professor of Pathology and Laboratory Medicine
“CancerVar won’t supplant human understanding in a clinical setting, yet it will essentially decrease the manual work of human commentators in ordering variations recognized through sequencing and drafting clinical reports in the act of accuracy oncology,” said Kai Wang, Ph.D., Professor of Pathology and Laboratory Medicine at CHOP and senior creator of the paper. “CancerVar archives and orchestrates different kinds of clinical evidence, including drug data, distributions, and pathways for physical changes, exhaustively.” By giving normalized, reproducible, and exact results for deciphering substantial variations, CancerVar can assist scientists and clinicians with focusing on transformations of concern. “
“Substantial variation grouping and translation are the most tedious strides of growth genomic profiling,” said Marilyn M. Li, MD, Professor of Pathology and Laboratory Medicine, Director of Cancer Genomic Diagnostics and co-creator of the paper. “CancerVar gives an integral asset that computerizes these two basic advances.” Clinical execution of this apparatus will essentially further develop the test time required to circle back and execute consistency, making the tests more effective and reasonable for all pediatric disease patients. “
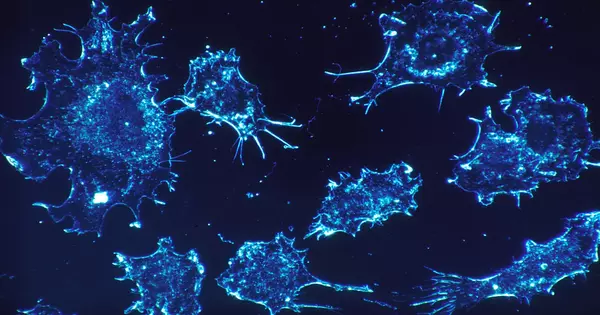
The development of cutting-edge sequencing (NGS) and accuracy in medication has prompted the distinguishing proof of millions of substantial disease variations. To more readily comprehend whether those changes are connected with or influence the clinical course of sickness, scientists have laid out a few data sets that inventory these variations. Nonetheless, those information bases didn’t give normalized translations of substantial variations, so in 2017, the Association for Molecular Pathology (AMP), American Society of Clinical Oncology (ASCO), and College of American Pathologists (CAP) together proposed principles and rules for deciphering, detailing, and scoring physical variations.
However, even with these rules, the AMP/ASCO/CAP arrangement plot didn’t determine how to execute these principles, so unique information bases were giving various outcomes. To tackle this issue, the CHOP specialists, including CHOP information researcher and co-senior creator of the paper Yunyun Zhou, Ph.D., created CancerVar, a better substantial variation understanding instrument utilizing order line programming called Python with a built-in web server. With an easy-to-use web server, CancerVar incorporates clinical proof for 13 million physical malignant growth variations from 1,911 disease evaluation qualities that were mined through existing investigations and data sets.
The device makes use of profound understanding to work on clinical understanding of those transformations. Clients can inquire about clinical translations for variations utilizing data, for example, the chromosome position or protein change, and intuitively calibrate how explicit scoring highlights are weighted in view of earlier information or extra client-indicated measures. The CancerVar web server produces computerized elucidating understandings, for example, whether the transformation is pertinent for conclusion or visualization or to a continuous clinical preliminary.
“This instrument demonstrates the way that we can utilize computational apparatuses to mechanize human-created rules and, furthermore, the way in which AI can direct navigation,” Wang said. “Future exploration ought to investigate applying this structure to different areas of pathology too.”