Hui Wang, left, an affiliate professor of business engineering, and An-Tsun Wei, a Ph.D. student, are co-authors of a paper that describes how research cloud knowledge gathered from networked 3D printers increases high quality management and printing accuracy. FAMU-FSU Engineering/M. Wallheiser
Researchers at the FAMU-FSU Faculty of Engineering are improving 3D printing capabilities by teaching machines to learn from one another.
Researchers demonstrated how knowledge from one printer can be used by different machines to improve effectivity and high quality in a recent study published in the IEEE Transactions on Automation Science and Engineering.
“Cloud manufacturing,” like the Internet of Things (IoT), is a new technology, according to Hui Wang, an affiliate professor on the FAMU-FSU Faculty of Engineering. “The technology indicates that data created by different production units can be shared with each other in a timely manner, and manufacturing may be encapsulated as online services to fulfill a variety of market demands.”
“The technology indicates that data created by different production units can be shared with each other in a timely manner, and manufacturing may be encapsulated as online services to fulfill a variety of market demands.”
Hui Wang, an affiliate professor on the FAMU-FSU Faculty of Engineering
According to the on-demand manufacturing platform Hubs 2021 3D Printing Traits Report, the global 3D printing market rose by 21% in 2020, regardless of the outcome of the global pandemic. This burgeoning industry is hastening the printing of everything from metal to organic materials. The race to optimize these procedures for maximum benefit is on.
Wang and his colleagues are striving to create new learning algorithms and strategies for managing the printing process. Tiny differences in the movement of a printer’s nozzle can create discrepancies in processing and faults in the finished product. To reduce printing flaws, their solution takes advantage of machine-shared information.
Wang and Wei demonstrated, via interconnected 3D printers, that switch learning is a way of obtaining “group intelligence” in which a group of learners collaborate to surpass a single learner. The skill can be applied to a wide range of products made from various materials. FAMU-FSU Engineering/M. Wallheiser
The researchers connected many printers on a cloud platform, then had the machines share knowledge about proper processing, which reduced the time required to organize and calibrate them.
The researchers also created a mathematical model to better understand the printing process, according to An-Tsun Wei, a doctoral student in the school’s Division of Industrial and Mechanical Engineering and a paper co-author.
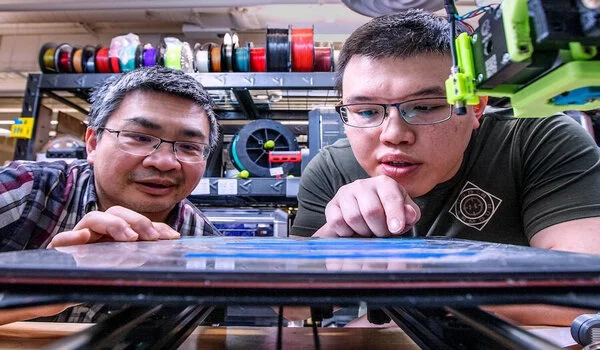
“We can estimate geometric print quality and any associated flaws that may occur with the model,” she explained. “The data can be utilized to compute adjustments in the input printing settings that are required to compensate for such faults.”
Traditional machine learning necessitates a large amount of experimental knowledge, which can be difficult to get. Printers used in manufacturing must be quickly modified to handle new tasks. Transfer studying expertise allows different printing methods to share experience, which speeds up the process. The study demonstrates the viability of exploiting previously shared knowledge from interconnected 3D printers to reduce testing time and improve the finished output.
With less testing, we can improve quality control faster and, as a result, swiftly adjust the printing processes to satisfy a variety of market demands, Wang explained. “This is particularly ideal for mass production of personalized goods, a manufacturing paradigm that is anticipated sooner or later.”
Wang refers to switch learning as a technique for obtaining “group intelligence,” in which a group of learning agents (learners) combine to surpass a single learner. The skill can be applied to a wide range of products made from various materials.