An infant giraffe or foal should figure out how to stroll on its legs as quickly as conceivable to keep away from hunters. Creatures are brought into the world with muscle coordination networks situated in their spinal lines. Nonetheless, learning the exact coordination of leg muscles and ligaments takes some time. At first, young creatures depend vigorously on permanently set up spinal string reflexes. While engine control reflexes are more important, they help the creature avoid falling and injuring themselves during their most memorable strolling adventures.The accompanying, further developed and exact muscle control should be rehearsed until ultimately the sensory system is all around adjusted to the youthful creature’s leg muscles and ligaments. No more uncontrollable staggering — the young creature can now keep an eye on the adults.
Specialists at the Max Planck Institute for Intelligent Systems (MPI-IS) in Stuttgart directed an examination study to figure out how creatures figure out how to walk and gain from staggering. They constructed a four-legged, canine-sized robot that assisted them with sorting out the subtleties.
“As designers and roboticists, we looked for the response by building a robot that highlights reflexes very much like a creature and gains from botches,” says Felix Ruppert, a previous doctoral understudy in the Dynamic Locomotion research group at MPI-IS. “If a creature staggers, is that an error?” I am assuming it happened once. In any case, on the off chance that it staggers habitually, it provides us with a proportion of how well the robot strolls. “
Felix Ruppert is the first creator of “Learning Plastic Matching of Robot Dynamics in Closed-circle Central Pattern Generators,” which will be distributed on July 18, 2022 in the journal Nature Machine Intelligence.
“As engineers and roboticists, we sought the answer by constructing a robot that displays reflexes just like an animal and learns from mistakes. Is it a mistake if an animal stumbles? Not if it just occurs once. However, if it trips up frequently, it tells us how well the robot walks.”
Felix Ruppert, a former doctoral student in the Dynamic Locomotion research group at MPI-IS
Learning calculation advances A virtual spinal line
In the wake of figuring out how to stroll in only 60 minutes, Ruppert’s robot takes full advantage of its mind-blowing leg mechanics. A Bayesian streamlining calculation directs the learning: the deliberate foot sensor data is coordinated with target information from the demonstrated virtual spinal rope running as a program on the robot’s PC. The robot figures out how to stroll by persistently contrasting sent and anticipated sensor data, running reflex circles, and adjusting its engine control designs.
The learning calculation adjusts the control boundaries of a central pattern generator (CPG). In people and creatures, these focal example generators are organizations of neurons in the spinal cord that produce occasional muscle withdrawals without input from the mind. The age of cadenced tasks such as walking, flickering, or assimilation is aided by focal example generator networks.Besides, reflexes are compulsory engine control activities set off by hard-coded brain processes that associate sensors in the leg with the spinal cord.
However, as longas the youthful creature strolls over an entirely level surface, CPGs can be adequate to control the development signals from the spinal line. A little knock on the ground, notwithstanding, changes the walk. Reflexes kick in and change the development examples to hold the creature back from falling. These flitting changes in the development signals are reversible, or “flexible,” and the development designs return to their unique setup after the aggravation. In any case, in the event that the creature doesn’t quit staggering over many patterns of development — in spite of dynamic reflexes — then the development designs should be relearned and made “plastic,” i.e., irreversible. In the infant creature, CPGs are at first not yet changed, and the creature staggers around, both on an even or lopsided landscape. Yet, the creature quickly figures out how its CPGs and reflexes control leg muscles and ligaments.
Morti, the Labrador-sized robot-canine, performs similarly to expectations.Much more importantly, the robot improves its development designs quicker than a creature, in around 60 minutes. Morti’s CPG is reproduced on a small and lightweight PC that controls the movement of the robot’s legs. This virtual spinal string is put on the quadruped robot’s back where the head would be. During the hour it takes for the robot to walk without a hitch, sensor information from the robot’s feet is consistently contrasted with the normal touch-down anticipated by the robot’s CPG. In the event that the robot staggers, the learning calculation changes how far the legs swing to and fro, how quickly the legs swing, and how lengthy a leg is on the ground. The changed movement likewise influences how well the robot can use its agreeable leg mechanics. During the educational experience, the CPG conveys adjusted engine messages with the goal that the robot hereafter staggers less and advances its strolling. In this structure, the virtual spinal rope has no express information about the robot’s leg configuration, its engines, or springs. Knowing nothing about the material science of the machine, it misses the mark on a robot “model.”
“Our robot is for all intents and purposes’ conceived,’ knowing nothing about its leg life systems or how they work,” Ruppert makes sense of. “The CPG looks like an implicit programmed strolling insight that nature gives and that we have moved to the robot.” The PC produces flags that control the legs’ engines, and the robot at first strolls and staggers. Information streams back from the sensors to the virtual spinal rope where sensor and CPG information are analyzed. In the event that the sensor information doesn’t match the normal information, the learning calculation changes the strolling conduct until the robot strolls well without staggering. Changing the CPG yield while keeping reflexes dynamic and checking the robot’s stagger is a center piece of the educational experience.
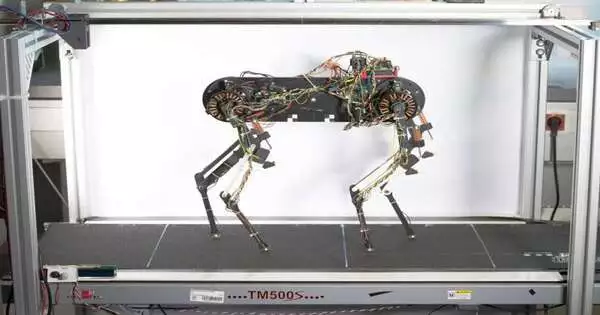
Energy-effective robot canine control
Morti’s PC attracts just five watts of force because of the method involved with strolling. Modern quadruped robots from noticeable producers, which have figured out how to run with the assistance of perplexing regulators, are substantially more power-hungry. Their regulators are coded with information on the robot’s precise mass and body calculation utilizing a model of the robot. They typically draw a few tens or hundreds of watts of power. Both robot types run progressively and effectively, yet the computational energy utilization is far lower in the Stuttgart model. It also provides extensive knowledge of animal life systems.
“We can only, with significant effort, research the spinal rope of a living creature. In any case, we can demonstrate one in the robot, “says Alexander Badri-Spröwitz, who co-created the distribution with Ruppert and heads the Dynamic Locomotion Research Group. “We realize that these CPGs exist in numerous creatures. We understand that reflexes are implanted; however, how can we combine the two so that creatures learn developments using both reflexes and CPGs? This is essential exploration at the crossing point between mechanical technology and science. The mechanical model offers us responses to questions that science alone can’t answer. “
More information: Felix Ruppert, Learning plastic matching of robot dynamics in closed-loop central pattern generators, Nature Machine Intelligence (2022). DOI: 10.1038/s42256-022-00505-4. www.nature.com/articles/s42256-022-00505-4