To enhance user engagement and interaction on their platforms, social media algorithms use features of human social behavior and learning patterns. Humans learned from members of our ingroup or from more prestigious individuals in prehistoric civilizations since this information was more likely to be credible and result in group success. However, with the rise of diverse and dynamic modern communities, particularly social media, these biases have grown less effective.
For example, someone we interact with online may not be trustworthy, and people can easily simulate grandeur on social media. A group of social scientists describes how the functions of social media algorithms are misaligned with human social instincts supposed to facilitate collaboration, which can lead to large-scale polarization and disinformation in a review published in the journal Trends in Cognitive Science.
“Several user surveys on Twitter and Facebook now indicate that most users are exhausted by the political content they see. A lot of people are dissatisfied, and there are a lot of reputational issues that Twitter and Facebook must deal with when it comes to elections and the dissemination of disinformation,” says first author William Brady, a social psychologist at Northwestern’s Kellogg School of Management.
It’s not that the algorithm is designed to disrupt cooperation. It’s just that its goals are different. And in practice, when you put those functions together, you end up with some of these potentially negative effects.
William Brady
“We wanted to put out a systematic review that’s trying to help understand how human psychology and algorithms interact in ways that can have these consequences,” says Brady. “One of the things that this review brings to the table is a social learning perspective. As social psychologists, we’re constantly studying how we can learn from others. This framework is fundamentally important if we want to understand how algorithms influence our social interactions.”
Humans are prejudiced to learn from others in a way that fosters collaboration and communal problem-solving, which is why they tend to learn more from people they consider to be prestigious and members of their ingroup. Furthermore, when learning biases initially emerged, ethically and emotionally charged information was prioritized since it was more likely to be relevant to maintaining group rules and guaranteeing collective survival.
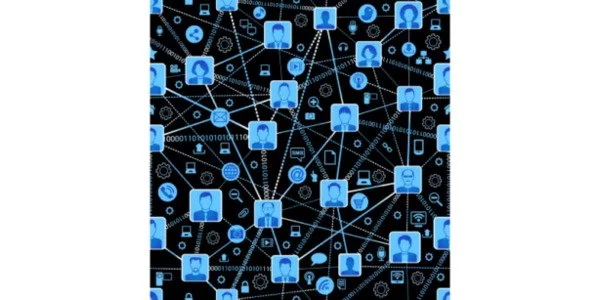
In contrast, algorithms typically select information that increases user engagement in order to increase advertising revenue. This means that algorithms amplify the very information that humans are biased to learn from, and they can oversaturate social media feeds with what the researchers call Prestigious, Ingroup, Moral, and Emotional (PRIME) information, regardless of the content’s accuracy or representativeness of a group’s opinions. As a result, radical political information or controversial themes are more likely to be amplified, and if users are not exposed to outside perspectives, they may develop a misleading sense of the majority opinion of certain groups.
“It’s not that the algorithm is designed to disrupt cooperation,” says Brady. “It’s just that its goals are different. And in practice, when you put those functions together, you end up with some of these potentially negative effects.”
To remedy this issue, the research team suggests that social media users become more aware of how algorithms function and why specific content appears in their feed. Social media businesses normally do not provide the exact specifics of how their algorithms choose for material, but one place to start may be by providing explanations for why a user is being displayed a specific post. Is it, for example, because the user’s friends are engaging with the content or because the content is popular in general? Aside from social media firms, the study team is creating their own interventions to teach people how to be more conscientious social media consumers.
Furthermore, the researchers advise that social media businesses adjust their algorithms to make them more effective at establishing community. Rather of emphasizing only PRIME information, algorithms should limit the amount of PRIME information they amplify and prioritize presenting people with a varied variety of content. These modifications may continue to boost interesting material while preventing more controversial or politically radical content from becoming overrepresented in feeds.
“As researchers, we understand the tension that companies face when it comes to making these changes and their bottom line. That’s why we actually think these changes could theoretically still maintain engagement while also disallowing this overrepresentation of PRIME information,” says Brady. “User experience might actually improve by doing some of this.”