A computer-guided design approach can generate more solutions while compensating for human inexperience and design fixation. Engineers deal with complex design situations every day, from building software to designing cars. ‘It’s a very difficult problem to optimize a technical system, whether it’s to make it more usable or energy-efficient,’ says Antti Oulasvirta, professor of electrical engineering at Aalto University and the Finnish Center for Artificial Intelligence.
Designers frequently use a combination of intuition, experience, and trial and error to guide them. Aside from being inefficient, this process can result in ‘design fixation,’ focusing on familiar solutions while leaving new avenues unexplored. A ‘manual’ approach will also not scale to larger design problems and is heavily reliant on individual skill.
Oulasvirta and colleagues investigated an alternative computer-assisted method that employs an algorithm to search through a design space, which is the set of possible solutions given multidimensional inputs and constraints for a specific design problem. They hypothesized that by scanning a broader range of solutions and balancing out human inexperience and design fixation, a guided approach could produce better designs.
We put a Bayesian optimizer in the loop with a human, who would try a combination of parameters. The optimizer then suggests some other values, and they proceed in a feedback loop. This is great for designing virtual reality interaction techniques.
Professor Antti Oulasvirta
The researchers set out with collaborators from the University of Cambridge to compare traditional and assisted approaches to design, using virtual reality as their laboratory. They used Bayesian optimization, a machine learning technique that both explores and steers towards promising solutions.
‘We put a Bayesian optimizer in the loop with a human, who would try a combination of parameters. The optimizer then suggests some other values, and they proceed in a feedback loop. This is great for designing virtual reality interaction techniques,’ explains Oulasvirta. ‘What we didn’t know until now is how the user experiences this kind of optimization-driven design approach.’
To find out, Oulasvirta’s team recruited 40 inexperienced designers to participate in their virtual reality experiment. The subjects had to figure out how to map the location of their real hand, which was holding a vibrating controller, to the virtual hand seen in the headset. In the process, half of these designers were free to follow their instincts, while the other half were given optimizer-selected designs to evaluate. Both groups were required to select three final designs that captured accuracy and speed in the 3D virtual reality interaction task. Finally, subjects expressed their confidence and satisfaction with the experience, as well as their sense of control over the process and the final designs.
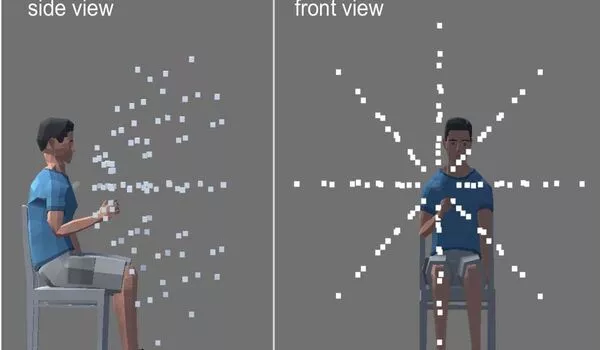
‘Objectively, the optimizer helped designers find better solutions, but designers did not like being hand-held and commanded,’ the results showed. It stifled their creativity and sense of agency,’ Oulasvirta reports. When compared to the manual approach, the optimizer-led process allowed designers to explore more of the design space, resulting in more diverse design solutions.
Designers who collaborated with the optimizer reported less mental strain and effort during the experiment. In comparison to the designers who did the experiment without a computer assistant, this group also scored lower on expressiveness, agency, and ownership.
‘There is undoubtedly a cost,’ says Oulasvirta. ‘With the optimizer, designers created better designs and covered a broader range of solutions with less effort. Their creativity and sense of ownership over the outcomes, on the other hand, were diminished.’ These findings have implications for the development of AI that aids humans in decision-making. People, according to Oulasvirta, need to be engaged in tasks like assisted design in order to maintain a sense of control, avoid boredom, and gain more insight into how a Bayesian optimizer or other AI is actually working.
‘We’ve seen that inexperienced designers, in particular, can benefit from an AI boost when participating in our design experiment,’ Oulasvirta says. ‘Our goal is that optimization becomes truly interactive without compromising human agency.’