Lately, profound learning calculations have accomplished striking outcomes in various fields, including creative disciplines. Truth be told, numerous PC researchers have effectively evolved models that can produce creative works, including sonnets, artworks, and portrayals.
Scientists at Seoul National University have as of late presented another creative profound learning system, which is intended to upgrade the abilities of a drawing robot. Their system, presented in a paper introduced at ICRA 2022 and pre-distributed on arXiv, permits a drawing robot to learn both stroke-based delivering and engine control all the while.
“The essential inspiration for our exploration was to make something cool with non-rule-based systems like profound learning; we thought drawing is something cool to show in the event that the drawing entertainer is an educated robot rather than a human,” Ganghun Lee, the main creator of the paper, told TechXplore. “Late profound learning methods have shown amazing outcomes in the creative region, yet the majority of them are about generative models which yield entire pixel results immediately.”
Rather than fostering a generative model that produces imaginative works by creating explicit pixel examples, Lee and his partners made a system that addresses drawing as a successive choice cycle. This successive cycle looks like the manner by which people would define individual boundaries, utilizing a pen or pencil to make a sketch slowly.
The scientists then wanted to apply their system to a mechanical drawing specialist so it could create portraits continuously utilizing a genuine pen or pencil. While different groups made profound learning calculations for “robot craftsmen” before, these models commonly required huge preparation datasets containing portrayals and drawings, as well as reverse kinematic ways to deal with helping the robot control a pen and sketch with it.
The system made by Lee and his partners, however, was not based on any true drawing models. All things considered, it can independently foster its own drawing systems over the long haul, through a course of experimentation.
“Our structure likewise doesn’t utilize reverse kinematics, which makes robot development a bit more severe, but it likewise allows the framework to find its own development stunts (changing joint qualities) to make development style as normal as could be expected,” Lee said. “As such, it straightforwardly moves its joints without natives while numerous automated frameworks usually use natives to move.”
Credit: Lee et al.
The model made by this group of analysts incorporates two “virtual specialists,” specifically the privileged and lower-class specialists. The privileged specialist’s job is to learn new drawing stunts, while the lower-class specialist learns viable development systems.
The two virtual specialists were prepared separately utilizing support learning methods and were just coupled whenever they had finished their individual preparation. Lee and his partners then tried their joined execution in a progression of genuine tests, utilizing a 6-DoF mechanical arm with a 2D gripper on it. The outcomes achieved in these underlying tests were extremely uplifting, as the calculation permitted the automated specialist to create great portrayals of explicit pictures.
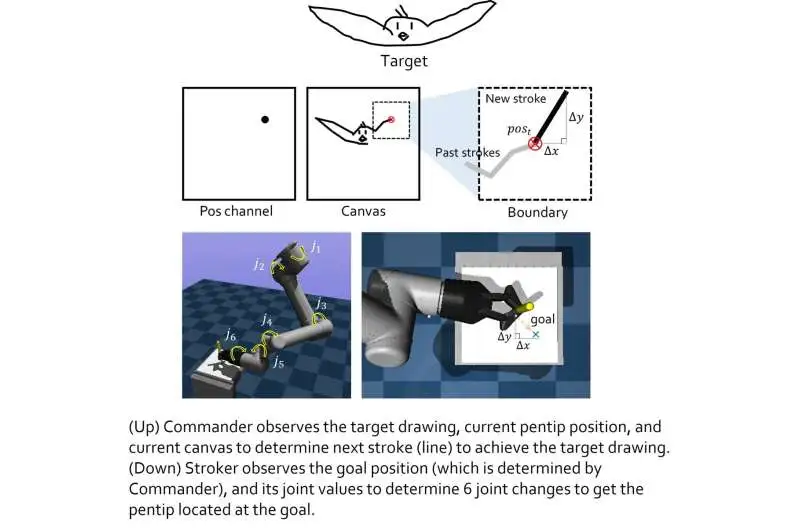
Credit: Lee et al.
“We find that the support learning-based modules prepared for every goal can be converged to accomplish greater cooperative targets,” Lee made sense of it. “In a progressive setting, choices from the upper specialist can be the’middle state,’ which permits the lower specialist to see to pursue lower choices. In the event that every specialist level is thoroughly prepared and summed up to the point of each expressing space, then an entire framework made of every module can do extraordinary things. In any case, that’s what the base condition is, as all support learning approaches have; thus, reward capabilities for every specialist ought to be very much molded (it’s difficult). “
Later on, the system made by Lee and his partners could be utilized to work on the exhibition of both existing and recently created automated drawing specialists. Meanwhile, Lee is creating comparable inventive support learning-based models, including a framework that can deliver imaginative montages.
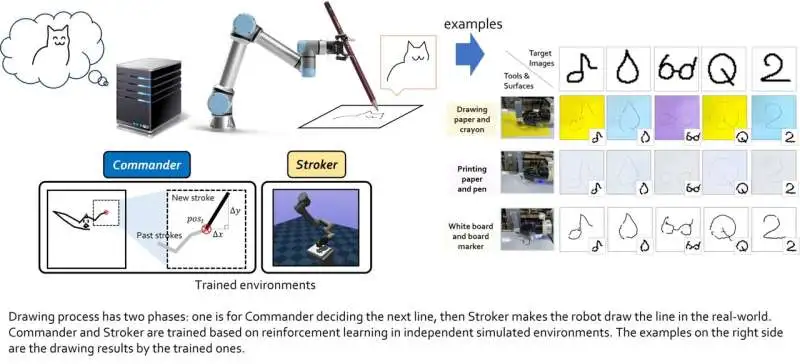
Credit: Lee et al.
“We might also want to broaden the errand to more muddled automated drawings like artworks, but I am currently leaning toward the useful issues of supporting learning applications themselves,” Lee added. “I trust our paper turns into a tomfoolery and significant illustration of unadulterated support for learning-based applications, particularly outfitted with robots.”
More information: Ganghun Lee, Minji Kim, Minsu Lee, Byoung-Tak Zhang, From scratch to sketch: deep decoupled hierarchical reinforcement learning for robotic sketching agent. arXiv:2208.04833v1 [cs.RO], arxiv.org/abs/2208.04833