As robots are slowly brought into different true conditions, engineers and roboticists should guarantee that they can securely work around people. Recently, they have presented different methodologies for assessing the positions and anticipating the development of robots continuously.
Scientists at the Universidade Federal de Pernambuco in Brazil have as of late developed another profound learning model to gauge the posture of automated arms and foresee their development. This model, presented in a paper pre-distributed on arXiv, is explicitly intended to upgrade the security of robots while they are teaming up or connecting with people.
“Roused by the need to anticipate mishaps during human-robot connection (HRI), we investigate a system that works on the security of individuals working in close proximity to robots,” one of the review’s analysts, Djamel H. Sadok, told TechXplore.”Act location is seen as a significant part of the general arrangement.” To this end, we propose another design for Pose Detection in view of Self-Calibrated Convolutions (SCConv) and Extreme Learning Machines (ELM). “
“Motivated by the need to foresee mishaps during human-robot interaction (HRI), we investigate a framework that improves the safety of people working in close proximity to robots. Pose detection is viewed as an important component of the overall solution. To that aim, we offer a new architecture for Pose Detection based on Self-Calibrated Convolutions (SCConv) and Extreme Learning Machines (ELM).”
Djamel H. Sadok
Assessing a robot’s posture is a fundamental stage for foreseeing its future developments and goals and thus lessening the gamble of it crashing into objects in its area. The methodology for present assessment and development expectations presented by Sadok and his partners has two key components, specifically a SCConv and an ELM model.
The SCConvs part further develops their model’s general spatial and channel conditions. The ELM approach, then, is known to be an effective way to deal with order information.
“We saw that there were no current examinations that joined these two advances with regards to our application,” Sadok made sense of. “As a result, we chose to test whether such a combination works on our application.”We likewise worked on the system by applying development forecast, taking into account the posture location, and utilizing repetitive brain organizations (RNN). “
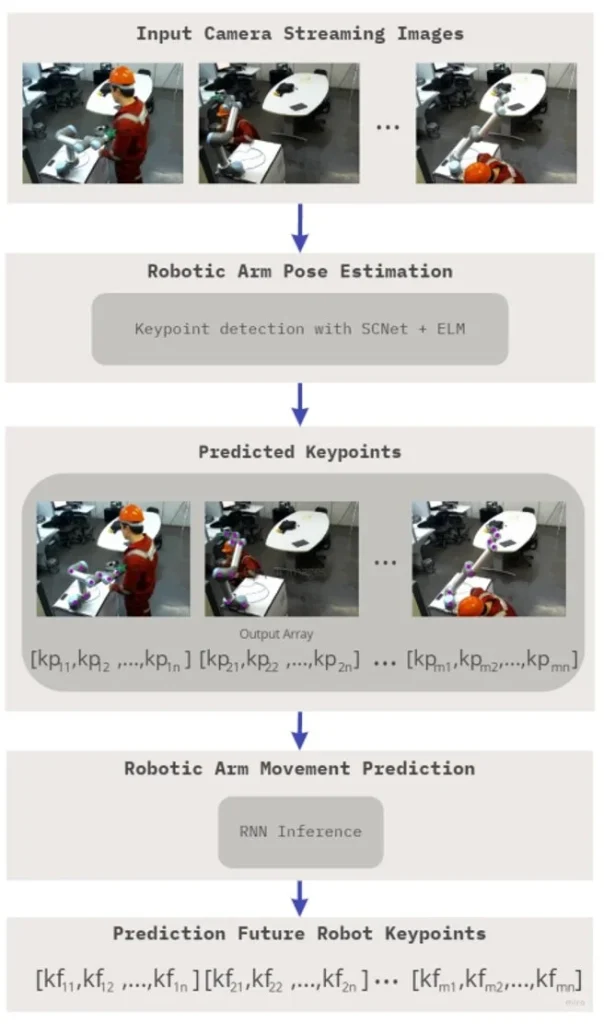
To begin, Sodok and his colleagues requested a custom dataset containing images of scenes in which a mechanical arm connects with a nearby human client.To make these pictures, they explicitly utilized the UR-5, a mechanical arm made by Universal Robots.
The analysts explained these pictures, especially the automated arm’s edges. This then permitted them to utilize the new dataset to prepare SCNet, their system’s SCConv-based part.
“Our objective was to work on the noticed blunder when contrasted with other referred to models, like VGG or ResNet,” Sadok said. To remove highlights, we utilized SCNet and applied the EML at the organization’s end. We next utilized the Long Short-Term Memory (LSTM) calculation and the Gated Recurrent Unit (GRU) to foresee the development. We look at this as another way to deal with tackling this issue.
Sadok and his partners assessed their system’s exhibition in a progression of starting tests, where they attempted to gauge the posture and foresee future developments of a UR-5 arm, as it was helping a human client with upkeep-related errands. They found that it accomplished extremely encouraging outcomes, identifying the automated arm’s posture and foreseeing its future developments with great degrees of exactness.
“We accept that our primary commitments are the age of a system fit for identifying the posture of a mechanical arm and its developments, thus working on the security of the arm,” Sadok said. “We likewise extended the relevance of SSConv and EML and approved their joined abilities.”
Later on, the structure created by this group of analysts could be utilized to work on the security of both existing and recently created automated frameworks. Also, the SCConv and ELM calculations they utilized could be adjusted and applied to different errands like human posture assessment, object location, and item order.
“We currently plan to grow our system to human posture location and mutually give a robot and posture assessment,” Sadok added. By consolidating the two pieces of information, we can deal with the forecast of the two developments, forestalling much further dangers coming about because of their connection as in a production line plant, and better controlling the degree of hazard.
More information: Iago Richard Rodrigues et al, A framework for robotic arm pose estimation and movement prediction based on deep and extreme learning models. arXiv:2205.13994v1 [cs.RO], arxiv.org/abs/2205.13994