We hear a ton about how environmental change will change the land, ocean, and ice. In any case, what will it mean for the mists?
“Low mists could evaporate and recoil like the ice sheets,” says Michael Pritchard, teacher of Earth System science at UC Irvine. “Or, on the other hand, they could thicken and turn out to be more intelligent.”
These two situations would bring about totally different future environments. What’s more, that, Pritchard says, is a contributor to the issue.
“When you ask two different climate models what the future will look like if we add a lot more CO2, they give you two very different answers. The main reason for this is the way clouds are represented in climate models.”
Michael Pritchard, professor of Earth System science
“On the off chance that you ask two distinct environmental models what the future will resemble when we add significantly more CO2, you will find two totally different solutions.” What’s more, the critical justification behind this is how mists are remembered for environmental models. “
Nobody rejects that mists and sprayers—pieces of ash and residue that nucleate cloud beads—are a significant piece of the environmental condition. The issue is that these peculiarities happen on a length-and-time-scale that the present models can’t possibly replicate. They are, in this way, remembered as models through an assortment of approximations.
Mists are the most common source of vulnerability and flimsiness, according to studies of global environmental models.
Retooling community codes
While the most progressive U.S. worldwide environmental models are battling to move toward a 4-kilometer worldwide goal, Pritchard gauges that models need a goal of something like 100 meters to catch the fine-scale fierce swirls that structure shallow cloud frameworks—multiple times more settled toward each path. It could take until 2060, as per Moore’s regulation, before the registering power is accessible to catch this degree of detail.
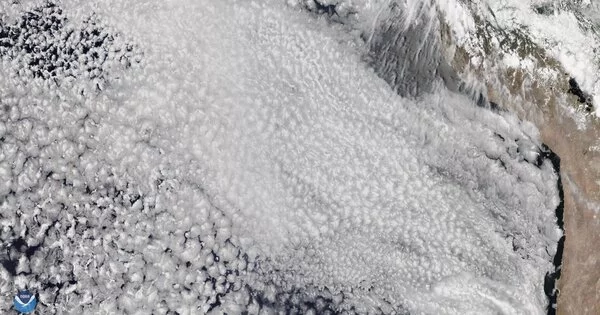
Credit: Creative commons
Pritchard is attempting to fix this glaring hole by breaking the environment displaying issue into two sections: a coarse-grained, lower-goal (100km) planetary model and many little fixes with a 100-to 200-meter goal. The two recreations run autonomously and afterward trade information like clockwork to ensure that neither reenactment goes off course nor becomes unreasonable.
His group accounted for the consequences of these endeavors in the Journal of Advances in Modeling Earth Systems in April 2022.
This environmental reproduction strategy, known as a “Multiscale Modeling Framework (MMF),” has been around since around 2000 and has long been a choice within the Community Earth System Model (CESM) model developed at the National Center for Atmospheric Research. thought has of late been partaking in a renaissance at the Department of Energy, where scientists from the Energy Exascale Earth System Model (E3SM) have been pushing it to new computational boondocks as a component of the Exascale Computing Project. Pritchard’s co-creator, Walter Hannah, from the Lawrence Livermore public lab, helps lead this work.
“The model does an end-go around the most difficult issue—the entire planet demonstrating,” Pritchard made sense of. “It has a great many little micromodels that catch things like reasonable shallow cloud arrangements that just arise in exceptionally high goals.”
“The Multiscale Modeling Framework approach is likewise great for DOE’s impending GPU-based exascale PCs,” said Mark Taylor, Chief Computational Scientist for the DOE’s Energy Exascale Earth System Model (E3SM) project and an examination researcher at Sandia National Laboratories. “Each GPU has the pull to run many of the micromodels while as yet matching the throughput of the coarse-grained lower-goal planetary model.”
Pritchard’s exploration and new methodology are made conceivable to a limited extent by the NSF-supported Frontera supercomputer at the Texas Advanced Computing Center (TACC). The quickest college supercomputer on the planet, Pritchard can run his models on Frontera at a time-and-length-scale open just on a small bunch of frameworks in the U.S. What’s more, he can test their true capacity for cloud displaying.
“We fostered a way for a supercomputer to best separate crafted by reenacting the cloud physical science over various regions of the planet that merit various measures of goal… so it runs a lot quicker, “the group composed.
Mimicking the climate in this manner gives Pritchard the goal of catching the actual cycles and fierce swirls engaged in cloud development. The analysts showed that the multi-model methodology didn’t deliver undesirable side results even where patches utilizing different cloud-settling network structures met.
“We were cheerful to the point that the distinctions were small,” he said. “This will give new adaptability to all clients of environmental models who need to concentrate on high goals in better places.”
Unraveling and reconnecting the different sizes of the CESM model was one test that Pritchard’s group survived. One more included reinventing the model so it could exploit the steadily expanding number of processors accessible in current supercomputing frameworks.
Pritchard and his group — UCI postdoctoral researcher Liran Peng and University of Washington research researcher Peter Blossey — handled this by breaking the inward spaces of the CESM’s implanted cloud models into more modest parts that could be tackled in equal parts using MPI, or message passing connection point, an approach to trading messages between various PCs running an equal program across circulated memory — and coordinating these estimations to utilize a lot more processors.
This appears to already provide a four-time average with incredible proficiency.” implies I can be multiple times as aggressive for my cloud-settling models,” he said. “I’m truly hopeful that this fantasy about regionalizing and MPI decaying is prompting a very surprising scene of what’s conceivable.”
Machine learning clouds
Pritchard sees one more encouraging methodology in AI, which his group has been investigating since 2017. “I’ve been extremely inspired by how performantly a stupid sheet of neurons can imitate these incomplete differential conditions,” Pritchard said.
Pritchard’s exploration and new methodology are made conceivable to some degree by the NSF-financed Frontera supercomputer at TACC. Furthermore, he can demonstrate their true capacity for cloud computing.
In a paper submitted the previous fall, Pritchard, lead creator Tom Beucler of UCI, and others portray an AI approach that effectively predicts environmental circumstances even in environmental systems it was not prepared for, where others have attempted to do as such.
This “environment invariant” model integrates actual information on environmental processes into the AI calculations. Their analysis, which used Stampede2 at TACC, Cheyenne at the National Center for Atmospheric Research, and Expanse at the San Diego Supercomputer Center, demonstrated that the AI strategy can maintain high precision across a wide range of environments and geologies.
“In the event that AI high-goal cloud physical science at any point succeeded, it would change everything about how we do environmental recreation,” Pritchard said. “I’m keen on perceiving how reproducibly and dependably the AI approach can prevail in complex settings.”
Pritchard is strategically situated to do as such. He is on the Executive Committee of the NSF Center for Learning the Earth with Artificial Intelligence and Physics, or LEAP, another Science and Technology Center supported by NSF in 2021 and coordinated by his long-lasting teammate on this point, Professor Pierre Gentine. Jump unites environment and information researchers to limit the scope of vulnerability in environmental demonstrations, giving more exact and noteworthy environmental projections that accomplish prompt cultural effects.
“All of the exploration I’ve done before is my idea of ‘throughput-restricted.'” Pritchard said. “My occupation was to create 10-to 100-year recreations. That compelled all my network decisions. Nonetheless, assuming the objective is to deliver short reenactments to prepare AI models, that is an “alternate scene.”
Pritchard hopes to eventually use the results of his 50-meter implanted models to fire develop a massive preparation library.”It’s a truly pleasant dataset to do AI on.”
Be that as it may, will AI mature quickly enough? There isn’t a moment to spare to sort out the fate of the mists.
Assuming those mists shrivel away, similar to ice sheets will, uncovering more obscure surfaces, that will enhance an Earth-wide temperature boost and every one of the perils that accompany it. Yet, assuming they do the alternate extremes of ice sheets and thicken up, which they could, that is less risky. Some have assessed this as a multi-trillion dollar issue for society. Furthermore, this has been referred to for quite a while, “Pritchard said.
Reproduction by reenactment, governmentally financed supercomputers are assisting Pritchard and others in moving toward the solution to this basic inquiry.
“I’m torn between certified appreciation for the U.S. public processing framework, which is so mind-boggling at aiding us create and run environmental models,” Pritchard said, “and feeling that we want a Manhattan Project level of new government financing and interagency coordination to take care of this issue in fact.”