Cybercriminals are thinking of increasingly canny ways of upsetting web-based administrations, accessing touchy information, or crashing web clients’ gadgets. A digital assault that has become extremely normal over the course of the last many years is the purported Conveyed Forswearing of Administration (DDoS) assault.
This sort of assault includes a progression of gadgets associated with the web, which are by and large alluded to as a “botnet.” This “bunch” of associated gadgets is then used to flood an objective server or site with “counterfeit” traffic, upsetting its activity and blocking it off to genuine clients.
Organizations and different clients generally use firewalls, hostile malware programming, or traditional interruption identification frameworks to protect their site or servers from DDoS assaults.However, identifying these assaults can be very challenging today, as they are often completed utilizing generative antagonistic organizations (GANs) and AI procedures that can figure out how to reasonably imitate the actions of genuine clients and authentic client demands.
“Our study paper was based on the challenge of identifying DDoS attacks, which are a sort of cyber-attack that can cause considerable damage to online services and network connectivity,”
Ali Mustapha, one of the researchers who carried out the study,
As a result, many existing malware frameworks eventually fail to attract clients.
Experts at the Institut Polytechnique de Paris, Telecom Paris (INFRES) have recently developed another computational strategy that could detect DDoS attacks more precisely and consistently.This strategy, described in a paper published in PCs and Security, is based on a long-term transient memory (LSTM) model, a type of repetitive brain organization (RNN) that can recognize long-term conditions in event successions.
“Our investigation paper was based on the issue of recognizing DDoS assaults, a type of digital assault that can cause significant harm to online administrations and business correspondence,” Ali Mustapha, one of the scientists who completed the review, told Tech Xplore.”While previous research has looked into the use of deep learning calculations to identify DDoS assaults, these methodologies may still be ineffective against attackers who use AI and deep learning strategies to make malicious assault traffic fit for bypassing identification frameworks.”
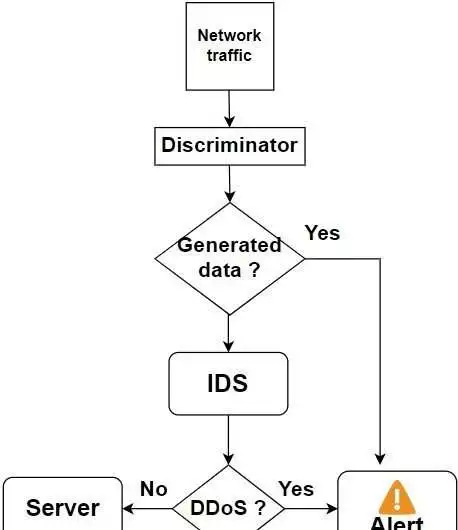
As a component of their review, Mustapha and his partners set off on a mission to devise a completely new AI-based approach that could work on the versatility of DDoS recognition frameworks. The technique they proposed depends on two separate models that can be coordinated into a solitary interruption identification framework.
“The main model is intended to decide if the approaching organization traffic is antagonistic and block it if it is thought to be fake,” Mustapha explained.” In any case, it is then sent to the subsequent model, which is responsible for recognizing whether it comprises a DDoS assault.” “Contingent upon the result of this examination, a related set of rules and a ready framework are utilized.”
The DDoS discovery apparatus proposed by this group of specialists enjoys various advantages over other interruption location frameworks created previously. Most prominently, it is vigorous and can recognize DDoS assaults with elevated degrees of exactness; it is versatile, and it could likewise be custom fitted to meet the exceptional requirements of explicit organizations or clients. Furthermore, it may be easily transmitted by internet service providers (ISPs), protecting them from both benign and hostile DDoS attacks.
“Our review yielded a few essential outcomes and achievements,” Mustapha made sense of. “At first, we assessed superior execution models that are prepared to recognize standard DDoS assaults, testing them against ill-disposed DDoS assaults produced through generative antagonistic organizations (GANs).” We saw that the models were generally incapable of identifying these sorts of assaults; notwithstanding, we had the option to refine our methodology and upgrade it to distinguish these assaults with an exactness surpassing 91%.
Beginning tests directed by Mustapha and his colleagues yielded extremely encouraging results, demonstrating how their framework could also distinguish more complex tasks from those explicitly designed to fool AI calculations.To further demonstrate their device’s true capability, the experts ran a series of tests in real time.They found that the framework fulfilled the constant DDoS assault location necessities, removing and breaking down network parcels in a restricted amount of time and without causing significant organization traffic delays.
The promising technique introduced in this paper could, before long, be coordinated inside existing and recently created security frameworks. Furthermore, it could motivate the improvement of comparative AI strategies for distinguishing DDoS assaults.
“As we look forward to future work, it will be fundamental to evaluate the viability of our IDS when tested with antagonistic assaults produced by elective models,” Mustapha added. “Moreover, we want to investigate the execution of web-based learning calculations, which empower the IDS to constantly refresh its model as it breaks down new information.” “By coordinating a gradual update highlight, the IDS could maintain its viability in recognizing advancing assault methods.”
More information: Ali Mustapha et al, Detecting DDoS attacks using adversarial neural network, Computers & Security (2023). DOI: 10.1016/j.cose.2023.103117