As the use of artificial consciousness spreads, more computation must occur — and more productively with less energy utilization — on neighborhood gadgets rather than in geologically distant server farms to overcome disappointing delays.A group of University of Tokyo engineers have interestingly tried the utilization of hafnium-oxide ferroelectric materials for actual repository registering—a kind of brain network that maps information onto actual frameworks and may achieve unequivocally such a development—on a discourse acknowledgment application.
They portrayed their outcomes in a paper introduced at the half-yearly 2022 IEEE Symposium on VLSI Technology and Circuits, held in Honolulu, Hawaii, June 12-17.
The advancement of computerized reasoning (AI) innovation and its slew of applications has recently exploded, but a significant impediment to its further development comes from enormous calculation cost and energy utilization, particularly where such calculation is completed by programming whose actual area lies in server farms, a significant separation from the client.
Indeed, even with information going through networks at the speed of light, there can be postponements of a few seconds or longer between a client’s solicitation and the conveyance of an application’s reaction. This is because photons travel long distances from client to server farm — sometimes half a world away — and then back.For purchaser applications, from computer games to voice collaborators, this little postponement can be disappointing. However, for strategic applications in government, from medical services to protection, such deferrals — known as idleness — can cost lives.
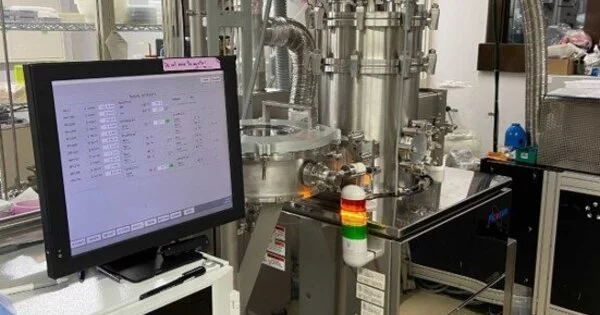
This machine is utilized to store a slender film of hafnium oxide-based ferroelectric materials to deliver ferroelectric entryway semiconductors (FeFETs) utilized in another actual repository registering engineering formulated by University of Tokyo specialists.
PC researchers and specialists center around two lines of assault concerning conquering this test: moving, in any event, a portion of the calculation expected from programming to equipment, and from the concentrated server farms, or cloud, back to a neighborhood gadget.
The principal methodology is fundamental since it’s a horrible idea to just endeavor to acquire effectiveness in the projects one is running and not likewise in the machines that they run on. The subsequent methodology, known as edge registering, diminishes inactivity as there is essentially less distance for information to travel. When your cell phone plays out the calculations engaged with a biometric check (and not the server farm some distance away), this is an illustration of edge registering’s scattering of calculations from the cloud back to the gadget.
Recently, actual repository registering (PRC) — in which effectiveness gains are accomplished in the neighborhood gadget’s equipment — has drawn a lot of consideration from designing specialists for its capacity to progress both these lines of assault. PRC is an outgrowth of the improvement of intermittent brain organizations (RNNs), a kind of AI that is appropriate to the handling of information over the long run (transient information) as opposed to that of static information. This is on the grounds that RNNs consider data from past contributions to think about ongoing information (consequently “intermittent”) and, from that, the result. As a result of this capacity to manage transient information, RNNs are reasonable for applications whose ends (or derivations) are delicate to the information’s succession or time-sensitive setting, for example, discourse acknowledgment, regular language handling, or language interpretation, and are utilized by applications like Google Translate or Siri.
In actual repository registering, the information is planned onto designs in some actual framework, or supply (like the examples in the construction of an attractive material, an arrangement of photons, or a mechanical gadget) that partakes in a higher layered space than the information. (A piece of paper is a space with one aspect greater than a piece of string, and a container has another aspect greater than the piece of paper.)Then, an example investigation is performed on spatio-transient examples on the last readout “layer” to figure out the condition of the repository. Since the AI isn’t prepared on the intermittent associations inside the repository, but just on the readout, more straightforward learning calculations are attainable, emphatically diminishing the number of calculations required, empowering high velocity learning and bringing down the energy utilization.
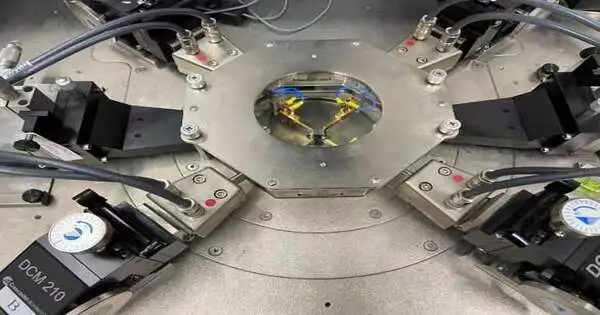
The framework is utilized to quantify the presentation of another equipment engineering utilizing hafnium-oxide ferroelectrics for the arising idea of actual repository registering.
The University of Tokyo engineers had already formulated another PRC design that utilizes ferroelectric entryway semiconductors (FeFETs) made of hafnium oxide-based ferroelectric materials. A great many people know all about ferromagnetism, in which an iron magnet is charged for all time in a specific polar bearing (one piece of the magnet turns into its “north” and the opposite end into its “south”). Ferroelectricity includes a practically equivalent peculiarity wherein certain materials—for this situation, hafnium oxide and zirconium oxide—experience an electric polarization (a shift of positive and negative electric charge) that can consequently be switched by the utilization of an outside electric field. This switchable polarization can, along these lines, store memory like any semiconductor. In 2020, the experts demonstrated that a fundamental activity of repository registering was also possible using these materials.
“These materials are as of now ordinarily utilized in semiconductor incorporated circuit fabricating processes,” said Shinichi Takagi, a co-creator of the paper and a teacher in the Department of Electrical Engineering and Information Systems at the University of Tokyo. “This implies that FeFET repositories are supposed to be incorporated with enormous scope into semiconductor coordinated circuit manufacture with little trouble compared with some more original material.”
While hafnium oxide-based ferroelectric materials have partaken in a lot of consideration in the semiconductor business as a result of their ferroelectricity, what kind of utilization FeFET-based actual repository registering was reasonable for and how well it acted in genuine applications presently couldn’t seem to be researched.
Having demonstrated their PRC engineering plausible quite a while back, the specialists then, at that point, tried it on a discourse acknowledgment application. They viewed it as 95.9% precise for discourse acknowledgment of the numbers zero to nine. This demonstrated the convenience of the innovation in a certifiable application.
The examples of ferroelectric entryway semiconductors (FeFETs) displayed here are utilized in an original, actual repository registering engineering formulated by a group of University of Tokyo specialists.
The specialists presently need to check whether they can expand the registered execution of their FeFET repositories as well as test them on different applications.
Eventually, the specialists wish to demonstrate that an AI chip with the hafnium oxide-based ferroelectric PRC engineering can accomplish an elevated degree of execution as far as incredibly low power utilization and continuous handling compared with traditional AI computation strategies and equipment.
More information: E. Nako, K. Toprasertpong, R. Nakane, M. Takenaka, and S. Takagi, “Experimental Demonstration of Novel Scheme of HZO/Si FeFET Reservoir Computing with Parallel Data Processing for Speech Recognition,” 2022 IEEE Symposium on VLSI Technology & Circuits: June 12-17, 2022.