A specialist has recently wrapped up composing a logical paper. She realizes her work could be profitable, according to another viewpoint. Did she ignore something? Or, on the other hand, maybe there’s a use for her exploration she hadn’t considered. A second arrangement of eyes would be perfect; however, even the most amicable of partners probably won’t have the option to save an opportunity to peruse all the expected foundation distributions to make up for lost time.
Kevin Yager, head of the electronic nanomaterials bunch at the Middle for Utilitarian Nanomaterials (CFN), a U.S. Division of Energy (DOE) Office of Science Client Office at DOE’s Brookhaven Public Research facility, has envisioned how late advances in computerized reasoning (computer-based intelligence) and AI (ML) could help logical conceptualizing and ideation. To achieve this, he has fostered a chatbot with information about the sorts of science he’s participated in.
Fast advances in simulated intelligence and ML have given rise to programs that can produce imaginative text and valuable programming code. These universally useful chatbots have, as of late, caught the public’s creative minds. Existing chatbots—in view of huge, different language models—need itemized information on logical sub-spaces.
“For a long time, CFN has been researching new ways to use AI/ML to accelerate nanomaterial discovery. It is currently assisting us in quickly identifying, cataloging, and selecting samples, automating tests, controlling equipment, and discovering new materials. Esther Tsai, a CFN scientist in the electronic nanomaterials group, is working on an AI companion to aid in the speeding up of materials research activities at the National Synchrotron Light Source II (NSLS-II).”
NSLS-II is another DOE Office of Science User Facility at Brookhaven Lab.
By utilizing a report recovery strategy, Yager’s bot is proficient in areas of nanomaterial science that different bots are not. The subtleties of this task and how different researchers can use this computer-based intelligence associate for their own work have, as of late, been distributed in Advanced Disclosure.
Ascent of the robots
“CFN has been investigating better approaches to using man-made intelligence and ML to speed up nanomaterial revelation for quite a while. Presently, it’s assisting us with rapidly distinguishing, indexing, and picking tests; computerizing tests; controlling gear; and finding new materials. Esther Tsai, a researcher in the electronic nanomaterials bunch at CFN, is fostering a computer-based intelligence ally to assist with accelerating materials research tests at the Public Synchrotron Light Source II (NSLS-II).” NSLS-II is one more DOE Office of Science Client Office at Brookhaven Lab.
At CFN, there has been a ton of work on simulated intelligence/ML that can assist with driving investigations using computerization, controls, mechanical technology, and examination; however, having a program that was capable of logical text was something that scientists hadn’t investigated as profoundly. Having the option to rapidly record, comprehend, and pass on data about a trial can help in various ways, from separating language hindrances to saving time by summing up bigger parts of work.
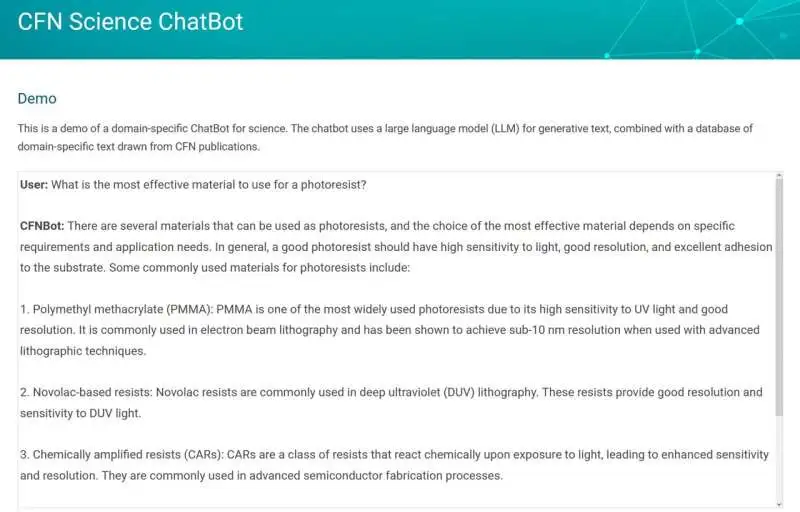
A demo of Yager’s chatbot responding to an inquiry connected with CFN’s exploration. Credit: Brookhaven Public Research Facility
Watching your language
To construct a particular chatbot, the program required explicit text—language taken from regions the bot is expected to zero in on. In this situation, the text is logically distributed. Space explicit text assists the simulated intelligence with demonstrating new wording and definitions and acquainting it with boondocks logical ideas. In particular, this organized arrangement of records empowers the computer-based intelligence model to ground its thinking on confided-in realities.
To copy regular human language, man-made intelligence models are prepared on existing text, empowering them to gain proficiency with the design of language, retain different realities, and foster a crude way of thinking. As opposed to difficultly retraining the simulated intelligence model on nanoscience text, Yager empowered it to look into pertinent data in an organized arrangement of distributions. Giving it a library of important information was just 50% of the fight. To utilize this text precisely and successfully, the bot would require a method for interpreting the right setting.
“A test that is normal with language models is that occasionally they ‘fantasize’ conceivable-sounding, however false things,” made sense of Yager. “This has been a central issue to determine for a chatbot utilized in research rather than one following through with something like composing verse. We don’t believe it should create reality or references. This should have been tended to. The answer to this was the sort of thing we call ‘inserting,’ an approach to classifying and connecting data rapidly in the background.”
Installing is a cycle that changes words and expressions into mathematical qualities. The subsequent “implanting vector” measures the significance of the text. At the point when a client asks the chatbot an inquiry, it’s likewise shipped off the ML implanting model to compute its vector esteem. This vector is utilized to look through a pre-figured information base of text pieces from logical papers that were comparatively implanted. The bot then utilizes text bits it finds that are semantically connected with the inquiry to get a more complete comprehension of the specific situation.
The client’s question and the message bits are joined into a “fast” that is shipped off a huge language model, a far-reaching program that makes messages demonstrated in regular human language, which produces the last reaction. The implanting guarantees that the text being pulled is significant with regards to the client’s inquiry. By giving text pieces from the assortment of confided-in archives, the chatbot creates answers that are authentic and obtained.
“The program should resemble a reference bookkeeper,” said Yager. “It is necessary to vigorously depend on the records to obtain replies. It should have the option to precisely decipher what individuals are asking and have the option to successfully sort out the setting of those inquiries to recover the most pertinent data. While the reactions may not be great yet, responding to testing questions and triggering a few fascinating contemplations while arranging new tasks and research is as of now capable.”
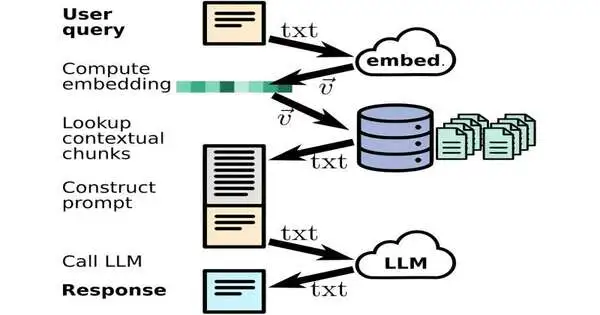
Credit: Brookhaven Public Lab
Bots enable people
CFN is creating simulated intelligence and ML frameworks as apparatuses that can free human analysts to figure out additional difficult and fascinating issues and to get a greater amount of their restricted time while PCs robotize dull errands behind the scenes. There are as yet numerous questions about this better approach to working, yet these inquiries are the beginning of significant conversations researchers are having right now to guarantee simulated intelligence/ML use is protected and moral.
“There are various errands that a space-explicit chatbot like this could clear from a researcher’s responsibility. Characterizing and coordinating reports, summarizing distributions, bringing up important data, and finding a workable pace in another effective region are only a couple of expected applications,” commented Yager. “However, I’m eager to see where all of this will go. We would never have thought where we are currently a long time ago, and I’m anticipating where we’ll be a long time from now.”
For analysts keen on giving this product a shot for themselves, the source code for CFN’s chatbot and related devices can be tracked down in this GitHub store.
More information: Kevin G. Yager, Domain-specific chatbots for science using embeddings, Digital Discovery (2023). DOI: 10.1039/D3DD00112A