AI plays a critical role in optimizing the manufacturing processes of perovskite solar cells, contributing to their efficiency, reliability, and scalability. Perovskite solar cells have received a lot of attention due to their high efficiency and low cost of production.
Perovskite semiconductor-based tandem solar cells convert sunlight to electricity more efficiently than conventional silicon solar cells. Further improvements in terms of stability and manufacturing processes are required before this technology can be commercialized.
Researchers from the Karlsruhe Institute of Technology (KIT) and two Helmholtz platforms, Helmholtz Imaging at the German Cancer Research Center (DKFZ) and Helmholtz AI, have discovered a way to predict the quality of the perovskite layers, and thus the quality of the resulting solar cells: It is possible to assess their quality from variations in light emission already during the manufacturing process, thanks to Machine Learning and new Artificial Intelligence (AI) methods.
Perovskite tandem solar cells combine a perovskite solar cell with a conventional silicon-based solar cell. These cells are considered next-generation technology because their efficiency is currently greater than 33%, which is significantly higher than that of conventional silicon solar cells. Furthermore, they use low-cost raw materials and are simple to manufacture. To achieve this level of efficiency, an extremely thin high-grade perovskite layer, only a fraction of the thickness of human hair, must be created.
Manufacturing these high-grade, multi-crystalline thin layers without any deficiencies or holes using low-cost and scalable methods is one of the biggest challenges.
Professor Ulrich W. Paetzold
“Manufacturing these high-grade, multi-crystalline thin layers without any deficiencies or holes using low-cost and scalable methods is one of the biggest challenges,” says tenure-track professor Ulrich W. Paetzold who conducts research at the Institute of Microstructure Technology and the Light Technology Institute of KIT.
Even under apparently perfect lab conditions, there may be unknown factors that cause variations in semiconductor layer quality: “This drawback eventually prevents a quick start of industrial-scale production of these highly efficient solar cells, which are needed so badly for the energy turnaround,” explains Paetzold.
AI Finds Hidden Signs of Effective Coating
To find the factors that influence coating, an interdisciplinary team consisting of the perovskite solar cell experts of KIT has joined forces with specialists for Machine Learning and Explainable Artificial Intelligence (XAI) of Helmholtz Imaging and Helmholtz AI at the DKFZ in Heidelberg. The researchers developed AI methods that train and analyze neural networks using a huge dataset. This dataset includes video recordings that show the photoluminescence of the thin perovskite layers during the manufacturing process.
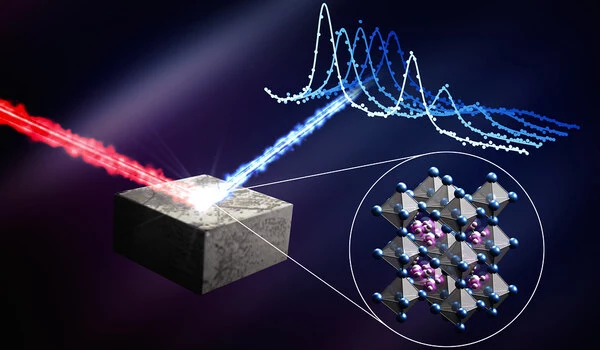
Photoluminescence is the radiant emission of semiconductor layers that have been excited by an external light source. “Because even experts couldn’t see anything special on the thin layers, the idea was born to train an AI system for Machine Learning (Deep Learning) to detect hidden signs of good or poor coating from the millions of data items on the videos,” explain Lukas Klein and Sebastian Ziegler from Helmholtz Imaging at the DKFZ.
The researchers then used Explainable Artificial Intelligence methods to filter and analyze the widely dispersed indications produced by the Deep Learning AI system.
“A Blueprint for Follow-Up Research”
The researchers found out experimentally that the photoluminescence varies during production and that this phenomenon has an influence on the coating quality. “Key to our work was the targeted use of XAI methods to see which factors have to be changed to obtain a high-grade solar cell,” Klein and Ziegler say. This is not the usual approach. In most cases, XAI is only used as a kind of guardrail to avoid mistakes when building AI models.
“This is a change of paradigm: Gaining highly relevant insights in materials science in such a systematic way is a totally new experience.” The conclusion drawn from the photoluminescence variation enabled the researchers to proceed to the next step. The AI was able to predict whether each solar cell would achieve a low or high level of efficiency based on which variation of light emission occurred at what point in the manufacturing process after the neural networks had been trained accordingly.
“These are extremely exciting results,” Ulrich W. Paetzold emphasizes. “We have a solid clue and know which parameters need to be changed in the first place to improve production thanks to the combined use of AI.” We can now conduct more targeted experiments and are no longer forced to look blindfolded for the needle in a haystack. This is a roadmap for future research that applies to many other aspects of energy research and materials science.”