For more than a century, enzymatic reaction mechanisms have been studied in the test tube, with most studies involving purified enzymes exposed to excessive amounts of substrate and tracking product formation over time.
Enzymes are biological catalysts that aid in biochemical transformations. An international team of bioinformatics researchers has developed a new method for predicting Michaelis constants, which govern reaction kinetics.
Enzymatic activity is measured by the breakdown of substrates and the production of products. Enzymatic activity can be measured using spectrophotometry, fluorescence, or radiolabeling. The enzymatic assay can be direct or indirect, with the latter involving the addition of substrate to the soil system and the determination of the end product formed. The indirect method entails extracting enzyme from soil and assaying it.
An organism would not be able to survive without enzymes. These biocatalysts catalyze a wide range of chemical reactions that produce the building blocks of cells. Enzymes are also widely used in biotechnology and in our homes, where they can be found in detergents, for example.
The Michaelis-Menten equation is used by scientists to describe metabolic processes facilitated by enzymes. The equation describes the rate of an enzymatic reaction as a function of substrate concentration, which is transformed into end products during the reaction. The ‘Michaelis constant,’ which characterizes the enzyme’s affinity for its substrate, is a key factor in this equation.
It takes a long time and a lot of effort to measure this constant in a lab. As a result, only a small number of enzymes have experimental estimates of these constants. We applied deep learning-based approach to 47 model organisms ranging from bacteria to plants and humans.
Prof. Lercher
It takes a long time and a lot of effort to measure this constant in a lab. As a result, only a small number of enzymes have experimental estimates of these constants. A team of researchers from the HHU Institute of Computational Cell Biology and Chalmers University of Technology in Stockholm has now used AI to predict the Michaelis constants from substrate and enzyme structures.
They applied their deep learning-based approach to 47 model organisms ranging from bacteria to plants and humans. Because this method requires training data, the researchers used previously collected data from nearly 10,000 enzyme-substrate combinations. They used Michaelis constants that had not been used during the learning process to test the results.
Prof. Lercher had this to say about the results’ quality: “We were able to demonstrate, using independent test data, that the process can predict Michaelis constants with an accuracy comparable to the differences between experimental values from different laboratories. Computers can now estimate a new Michaelis constant in a matter of seconds without the need for an experiment.”
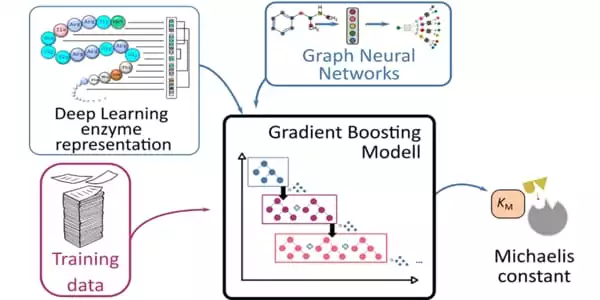
The sudden availability of Michaelis constants for all enzymes in model organisms opens up new avenues for metabolic computer modeling, as highlighted in an accompanying article in the journal PLOS Biology.
Scientists use the Michaelis-Menten equation, which is promoted by enzymes, to describe the metabolic process. This equation depicts the rate of enzymatic reaction as a function of concentration. During the reaction, the substrate is converted to the final product. The “Michaelis constant,” which characterizes the enzyme’s affinity for the substrate, is at the heart of this equation.
In the lab, measuring this constant takes a significant amount of time and effort. As a result, experimental estimates of these constants are only available for a few enzymes. A group of researchers from the HHU Institute for Computational Cell Biology and Chalmers Institute of Technology in Stockholm used AI to predict Michaelis constants from substrate and enzyme structures.
They used a deep learning approach on 47 different model organisms, ranging from bacteria to plants and humans. This method necessitates the use of training data, so the researchers used approximately 10,000 pieces of known data. Enzyme-substrate interaction. They used a Michaelis constant that was not used in the Learning process to test the results.
Our nanokits are used to measure enzyme activity in a single cell, and even in a single cellular compartment. In contrast to current methods that use complicated structural designs or surface functionalization of probes, the nanokits have adapted features from well-established kits and integrated the kit components and detector in a single nano-capillary. As a result, this approach provides a specific device for characterizing enzyme activity at the single cell and subcellular levels.