Nobody likes sitting at a red light. Yet, signalized crossing points aren’t simply a minor annoyance for drivers; vehicles consume fuel and discharge ozone-harming substances while trusting that the light will change.
Consider a scenario in which drivers could plan their trips so that they arrive at the intersection when the light is green.While that may be a godsend for a human driver, it very well may be accomplished all the more reliably by an independent vehicle that utilizes computerized reasoning to control its speed.
In another review, MIT analysts show an AI approach that can figure out how to control an armada of independent vehicles as they approach and travel through a signalized convergence such that keeps traffic streaming without a hitch.
Utilizing recreation, they found that their methodology diminishes fuel utilization and emissions while further developing normal vehicle speed. The procedure obtains the best outcomes in the event that all vehicles out and about are independent. However, regardless of whether just 25% utilizes their control calculation, it actually prompts significant fuel and discharge benefits.
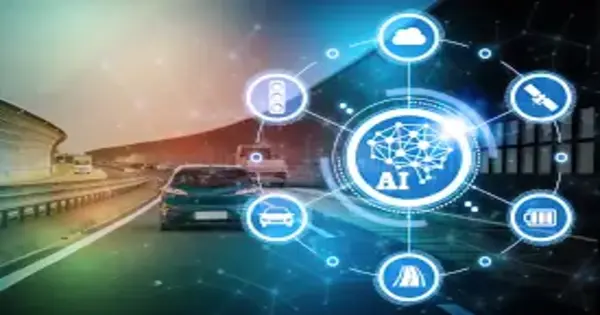
“This is a truly fascinating spot to intercede.” Nobody’s life has been better since they were stuck at a crossing point. With a great deal of other environmental change intercessions, there is a personal satisfaction contrast that is normal, so there is a boundary to section there. “Here, the obstruction is a lot lower,” says senior creator Cathy Wu, the Gilbert W. Winslow Career Development Assistant Professor in the Department of Civil and Environmental Engineering and an individual from the Institute for Data, Systems, and Society (IDSS) and the Laboratory for Information and Decision Systems (LIDS).
The lead creator of the review is Vindula Jayawardana, an alumni understudy in LIDS and the Department of Electrical Engineering and Computer Science. The exploration will be introduced at the European Control Conference.
Convergence complexities
While people might drive past a go-ahead without really thinking about it, crossing points can introduce billions of various situations depending upon the quantity of paths, how the signs work, the quantity of vehicles and their paces, the presence of walkers and cyclists, and so on.
Regular methodologies for handling convergence control issues utilize numerical models to settle on one straightforward, optimal crossing point. That looks great on paper, yet it won’t hold up in reality, where traffic designs are frequently similarly untidy.
“This is a fascinating area in which to intervene. Nobody’s life has improved as a result of being stuck at an intersection. A quality-of-life difference is expected with many other climate change initiatives, thus there is a barrier to entry there. The barrier is substantially lower here.”
senior author Cathy Wu, the Gilbert W. Winslow Career Development Assistant Professor in the Department of Civil
Wu and Jayawardana changed gears and moved toward the issue, utilizing a model procedure known as profound support learning. Support learning is an experimentation strategy where the control calculation figures out how to pursue a group of choices. It is compensated when it tracks down a decent arrangement. With profound support learning, the calculations use suspicions advanced by a brain organization to track down easy routes to great successions, regardless of whether there are billions of conceivable outcomes.
This is valuable for taking care of a long-skyline issue like this; the control calculation should issue upwards of 500 speed increase directions to a vehicle throughout a drawn out time span, Wu makes sense of.
“What’s more, we need to get the succession just before we realize that we have worked effectively at moderating discharges and getting to the crossing point at a decent speed,” she adds.
Yet, there’s an extra flaw. The scientists believe the framework should get familiar with a technique that diminishes fuel utilization and limits the effect on movement time. These purposes can struggle.
To lessen travel time, we maintain that the vehicle should go quickly. To diminish emissions, we believe the vehicle should dial back or not move by any means. Those contending prizes can be exceptionally confounding to the learning specialist, “Wu says.”
While it is trying to tackle this issue in its full oversimplification, the scientists utilized a workaround utilizing a procedure known as remuneration molding. With remuneration forming, they give the framework some space to acquire information it can’t learn all alone. For this situation, they punished the framework at whatever point the vehicle arrived at a stand-still, so it would figure out how to stay away from that activity.
Traffic tests
When they cultivated a powerful control calculation, they assessed it by utilizing a traffic reenactment stage with a solitary crossing point. The control calculation is applied to an armada of associated independent vehicles, which can speak with impending traffic signals to get signal stage and timing data and notice their prompt environmental elements. The control calculation advises every vehicle on how to speed up and decelerate.
Their framework made no unpredictable traffic as vehicles moved toward the crossing point. (Unpredictable traffic happens when vehicles are compelled to reach a standstill because of halted traffic ahead. In recreations, more vehicles endured on a solitary green stage, which outflanked a model that reproduces human drivers. When compared with other improvement strategies additionally intended to keep away from unpredictable traffic, their method brought about greater fuel utilization and outflow decreases. Assuming that each vehicle out and about is independent, their control framework can reduce fuel utilization by 18% and carbon dioxide emissions by 25%, while supporting travel speeds of 20%.
“A solitary intercession having a 20 to 25% decrease in fuel or outflows is truly unimaginable.” Yet, what I see as fascinating, and what I truly wanted to see, is this non-straight scaling. Assuming we just control 25% of vehicles, that gives us half of the advantages with regards to fuel and discharge decrease. “That implies we don’t need to hold on until we get to 100 percent independent vehicles to get benefits from this methodology,” she says.
Not too far off, the specialists need to concentrate on association impacts between numerous convergences. They also plan to investigate how different crossing point set-ups (number of paths, signals, timings, and so forth) can impact travel time, discharges, and fuel utilization. Also, they plan to concentrate on what their control framework could mean for wellbeing when independent vehicles and human drivers share the street. For example, despite the fact that autonomous vehicles might drive uniquely in contrast to human drivers, more slow streets and streets with additional reliable rates could further develop wellbeing, Wu says.
While this work is still in its beginning phases, Wu sees this methodology as one that could be all the more practically carried out in the near term.
“The point of this work is to make at least some difference in reasonable portability. We need to dream, too, yet these frameworks are large beasts of inactivity. “Discharging points of mediation that are little changes to the framework yet have critical effect is something that gets me up toward the beginning of the day,” she says.