To handle different errands, robots ought to have the option to deal with and control various items and materials, including paper. While roboticists have successfully worked on the ability of humanoid robots or mechanical grippers to deal with a variety of materials, paper collapsing remains a little-studied topic in the mechanical technology field.
An exploration group at the University of California, Los Angeles (UCLA) has as of late made another computational structure that permits a robot to handle paper collapsing and the Oriental specialty of origami. This structure, presented in a paper pre-distributed on arXiv, consolidates a profound learning calculation with material science reproductions.
“In spite of the fact that there has been significant mechanical technology research on deformable materials dealing with control, the control of paper has been a somewhat neglected point, regardless of the pervasiveness of paper in day-to-day existence,” Dezhong Tong and Andrew Choi, two of the scientists who did the review, told Tech Xplore.
“To the best of our knowledge, our framework is the first to take advantage of this synergistic approach, which we believe can be broadly applied in a wide range of deformable manipulation tasks, such as cable management, knot tying, robotic kirigami, and so on,”
Dezhong Tong and Andrew Choi, two of the researchers who carried out the study,
“The two most applicable examinations on this point were directed by research groups at Aalto College in Finland and at Bielefeld College in Germany. “The principal manages textures, which are a lot more straightforward to control than paper, while the second spotlights collapsing paper, yet fosters a convoluted robot situation, including human-like controllers, that is computationally grave.”
The new work by Tong, Choi, and their associates was propelled by the absence of straightforward and powerful automated paper collapsing frameworks. The group’s main goal was to create a simple yet supportive framework that could overlay pieces of paper with a single mechanical controller.
The robot control system proposed by the scientists “educates” the robots’ ways of behaving from an actual viewpoint so they can more readily handle errands that include genuinely wise control. All the more explicitly, the group prepared a fake brain organization (ANN) inside a disconnected climate, including material science reproductions of collapsing paper. During preparation, the organization figured out how paper “acts” when it is exposed to various handles from various actual positions.
“The preparation information was produced simply by means of material science-based virtual experiences,” Tong and Choi made sense of. “Consequently, on the web and continuously, the prepared brain network quickly made forecasts of that outcome in ideal control directions.” Another notable difference is our use of scaling investigation, a strategy adopted from numerical physical science, to nondimensionalize brain organization forecasts.
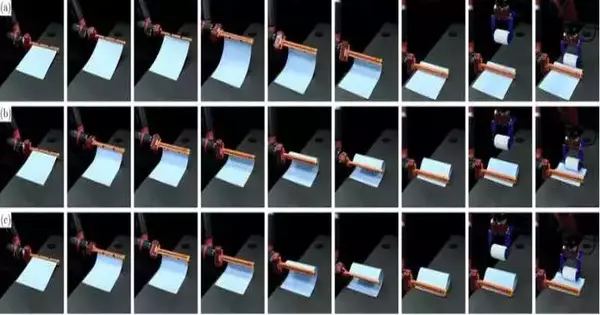
From solid (card) paper, half a valley collapses.(a) The instinctive gauge control bombs severely as the firmness of the paper causes unreasonable sliding during the collapsing system. (b) Our open-circle control calculation accomplishes a huge improvement over the pattern with insignificant sliding. (c) Our closed circle control calculation accomplishes an almost wonderful overlay.
In this article, the expression “non-dimensionalization” alludes to making the information and result free of their units through a numerical physical science strategy. The non-dimensionalized amount is unitless. Subsequently, changing the units of the framework won’t impact the investigation.
With regards to this review, this interaction enjoys prominent benefits. It works on the speculation of the control system, permitting the robot to overlay pieces of paper of various thicknesses or with various calculations without requiring preparation for every one of these kinds of paper.
Furthermore, non-dimensionalization diminishes the “dimensionality” of the collapsing paper issue. At the end of the day, it makes it more straightforward to prepare the brain network while working on its constant execution.
“Given the profoundly nonlinear behavior of numerous deformable items, roboticists have attempted to cultivate powerful strategies to control robots in ways that fulfill genuine control errands, for example, paper collapsing,” Tong and Choi explained.
“An interesting finding of our work is that AI calculations and physical science-based scaling investigation are integral and exceptionally viable for the motivations behind automated deformable item control.” From one point of view, traditional numerical material science models suffer from unmanageable computational expense when managing articles such as paper, making web delivery impossible.However, relying on AI to solve the problem results in a control plot that appears only for situations within the scope of the preparation information (i.e., execution will decline dramatically regardless of whether the material of the controlled deformable item is changed).
The new work by this group of scientists features the benefit of consolidating scaling investigation with profound realization while tending to certifiable automated object control issues. Their underlying discoveries are extremely encouraging, both with regards to exactness and proficiency.
Later on, the system made by Tong, Choi, and their associates could serve to fundamentally upgrade the item control abilities of robots. For example, it could permit them to deal with a more extensive scope of articles in cutting-edge ways, including pieces of paper and things made of other deformable materials.
“As far as anyone is concerned, our system is quick to take advantage of this synergistic methodology, which we accept can be generally utilized in an expansive assortment of deformable control undertakings, like link the executives, tie tying, mechanical kirigami, and so forth,” Tong and Choi added. “We might want to extend our work to encompass more intricate collapsing undertakings, like mechanical origami.” “A fascinating objective is to empower a robot to overlay a piece of paper into various designs, for example, paper planes, paper frogs, and so on.”
More information: Dezhong Tong et al, Deep Learning of Force Manifolds from the Simulated Physics of Robotic Paper Folding, arXiv (2023). DOI: 10.48550/arxiv.2301.01968
Vladimir Petrik et al, Feedback-based Fabric Strip Folding, 2019 IEEE/RSJ International Conference on Intelligent Robots and Systems (IROS) (2020). DOI: 10.1109/IROS40897.2019.8967657