Microplastics are surrounding us — in the water we drink, the food we eat, and the air we breathe. In any case, before specialists can comprehend the genuine effect of these particles on wellbeing, they need quicker and more successful ways of evaluating what is there.
Two late investigations by analysts at the University of Toronto’s Faculty of Applied Science and Engineering have proposed new techniques that utilize AI to make the most common way of counting and arranging microplastics simpler, quicker, and more reasonable.
It’s “truly tedious to dissect a water test for microplastics,” says Elodie Passeport, an academic administrator in the divisions of common and mineral designing and substance designing.
It can take as long as 40 hours to completely break down an example the size of a Mason container — and that example is from one moment. It turns out to be particularly troublesome when you need to make correlations over the long run or notice tests from various waterways. “
Last month, the United Nations Environment Program embraced a noteworthy goal to end plastic contamination, which it called “a fiasco really taking shape,” imperiling human wellbeing, marine and coastal species, and worldwide biological systems.
“It’s really time-consuming to analyze a water sample for microplastics, it can take up to 40 hours to fully analyze a sample the size of a Mason jar—and that specimen is from one point in time. It becomes especially difficult when you want to make comparisons over time or observe samples from different bodies of water.”
says Elodie Passeport, an associate professor in the departments of civil and mineral engineering and chemical engineering.
Microplastics can require hundreds of millennia to biodegrade. However, it isn’t simply the noticeable plastic decline that is an issue: Over time, plastic separates into increasingly small particles. Those pieces that are under five millimeters in size yet more prominent than 0.1 micrometers are characterized as microplastics.
Specialists who concentrate on the impacts of microplastics are as yet attempting to comprehend what these little pieces could mean for human and ecological wellbeing in ways that are unique in relation to the mass material.
Although past examinations have exhibited the presence of microplastics in different conditions, the norms for how to measure their levels—and basically, how to analyze various examples over the long haul and space—are as yet arising. Passeport worked with Shuyao Tan, a Ph.D. understudy in substance designing, and Joshua Taylor, an academic administrator in the division of electrical and PC designing, to address the test.
Passeport says, “We found out if there could be an unrefined estimation that could foresee the centralization of microplastics.”
In a joint effort with Professor Taylor, who has skills in AI and improvement, we laid out an expectation model that utilizes a prepared calculation that can appraise microplastic counts from total mass estimations.
“Our technique has ensured blunder-following properties with comparative outcomes to manual counting, yet it’s less expensive and quicker, taking into consideration the investigation of numerous examples from different focuses to assess microplastic contamination,” she adds.
The group’s examination, distributed in January in ACS ES&T Water, enjoys the benefit of permitting analysts to physically handle just a negligible portion of their gathered examples and anticipate the amount of the rest by utilizing a calculation, without presenting extra blunder or fluctuation.
“Analysts chipping away at microplastic examination need to know the number of plastic particles there are, the sorts of particles, the polymers and shapes,” says Tan.
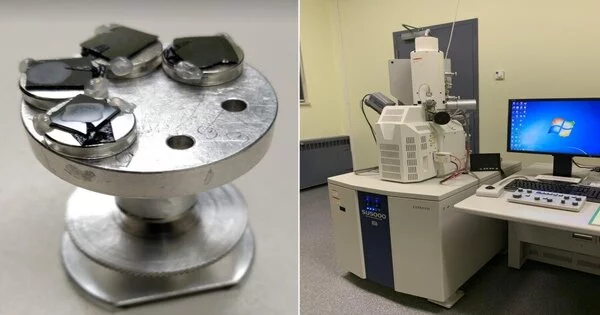
“With this data, they can then concentrate on the impacts of microplastic contamination on residing living beings as well as where this contamination is coming from, so they can manage it at the source.”
Old-style measurement techniques utilizing noticeable light microscopy require the utilization of tweezers to count tests individually under an optical magnifying lens—a labor-intensive task that is inclined to human error.
In an examination distributed in Science of the Total Environment, Ph.D. applicant Bin Shi in the division of materials science and design, who is administered by Associate Professor Jane Howe, utilized profound learning models for the programmed measurement and characterization of microplastics.
Shi utilized examining electron magnifying instruments to fragment pictures of microplastics and arrange their shapes. When compared to visual screening strategies, this method provided a greater depth of field and better surface detail, which can help prevent falsely distinguishing proof of small and straightforward plastic particles.
“Profound learning permits our way to deal with the acceleration of the evaluation of microplastics, particularly since we needed to eliminate different materials that could make misleading recognizable pieces of proof, like minerals, substrates, natural matter, and organic entities,” says Shi.
“We had the option to foster exact calculations that could really evaluate and group the articles in such complex conditions.”
The variety in the substance arrangement and states of microplastics can cause hardships for some specialists, particularly since there is no normalized technique to measure microplastics.
Shi gathered microplastic tests in different shapes and compound arrangements—like dabs, films, strands, froths, and parts—from sources like face wash, plastic jugs, froth cups, washing and drying machines, and clinical covers. He then handled pictures of the singular examples, utilizing the examining electron magnifying instrument to make a library of many pictures.
The undertaking is the principal named open-source dataset for the microplastics picture division, which permits analysts from everywhere in the world to profit from this new technique and foster their own calculations well defined for their examination advantages.
“This strategy can additionally go down to the size of nanoplastics, which are particles less than 0.1 micrometers,” Shi says.
On the off chance that we can keep on extending our library of pictures to incorporate more microplastic tests from various conditions with shifted shapes and morphologies, we can screen and break down microplastic contamination significantly more.
For the present, the objective of Passeport and Tan’s prescient model is to be a symptomatic device that can assist specialists in recognizing regions where they ought to focus their scientific endeavors for more top-to-bottom advancements.
The group also trusts that this strategy can engage resident researchers to screen for microplastic contamination in their own surroundings.
Passeport says, “People can gather tests, channel and dry them to get the weight, and afterward utilize a prepared calculation to anticipate how much microplastics there will be.”
As we proceed with our work, we need to present some programmed preparation test determination strategies that will allow people to simply click a button and consequently select the preparation test, adds Tan.
“We need to make our strategies simple so they can be utilized by anybody without requiring any information on AI or science.”