Fluorescence microscopy permits analysts to concentrate on unambiguous designs in complex natural examples. Be that as it may, the picture made utilizing fluorescent tests experiences obscuring and foundational commotion. The most recent work from NIBIB analysts and their partners presents a few novel picture reclamation procedures that make sharp pictures with fundamentally diminished handling time and figuring power. The examination is distributed in Nature Techniques.
The utilization of man-made reasoning, most notably brain networks that use profound understanding to eliminate obscuring and foundational disturbance in a picture, is the foundation of modern picture handling.The fundamental system is to show the profound learning organization what a hazy, noisy picture would look like without the haze and commotion. The organization should be prepared to do this with enormous datasets of sets of sharp and fluffy adaptations of a similar picture. The time and cost required to create large preparation informational collections is a critical barrier to using brain networks.
3D delivery of a cleared-mind tissue piece (typically 1.4 2.3 0.5 mm3) communicating td Tomato in axons gained with 0.7/0.7 NA cleared-tissue diSPIM, compared to crude single view, double view joint deconvolution, and RLN forecast. In comparison to the crude information, the RLN expectation further develops picture goal and differentiation. The joint deconvolution yield is intriguing and shows many fewer neurites than the crude information and RLN forecast, which is logical given the disappointments of enlistment between the two crude perspectives.
“It’s incredibly interesting to see how well we’re doing with using synthetic data to train neural networks. Creating or collecting training data sets has been a huge barrier in image processing. This work’s findings—that synthetic data works, especially when combined with RLN—have the potential to herald in a new era in image processing, which we are actively pursuing.”
Hari Shroff, Ph.D., one of the lead authors of the study.
Images were cleaned up using conditions prior to the use of brain organizations, a process known as deconvolution. Richardson-Lucy Deconvolution (RLD) utilizes a condition that utilizes information on the obscuring effect imparted by the magnifying lens to clear up the picture. The image is manipulated through the situation several times in order to further develop it. Each pass through the situation is known as an “emphasis,” and numerous emphases are expected to make a reasonable picture. The assets and time that it takes to run a picture through numerous emphases are a fundamental downside of the RLD approach.
RLD is viewed as material science-driven on the grounds that it portrays the actual properties that cause the obscuring and commotion in a picture. Brain networks are supposed to be information-driven in light of the fact that they should take a gander at a ton of pictures (information) to realize what is a fluffy or clear picture. The NIBIB group looked to use the benefits and moderate the burdens of each strategy by consolidating them. The outcome is a brain network that likewise utilizes RLD, or the Richardson-Lucy Organization (RLN).
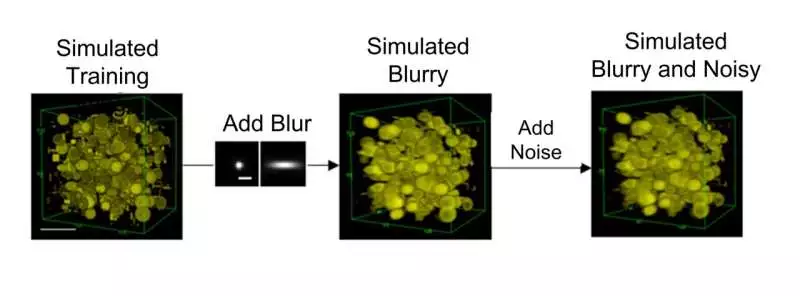
Commotion and obscurity were added to computer-generated images of spots, circles, and circles to create engineered data used to prepare brain organizations to clean up fluffy images. Credit: Li, et al. Nat Techniques 19, 1427–1437 (2022), and Innovative Lodge Attribution 4.0 Global Permit
A brain network identifies characteristics in the matched matches that will help it figure out how to make a fluffy picture understandable. Surprisingly, the researchers who designed these organizations generally have no idea what specific characteristics the organization is utilizing to achieve this accomplishment. What is known is that the organization’s recognized highlights are put together in some measure to a limited extent in relation to the actual properties of the magnifying lens and can thus be addressed by conditions.
The team developed a routine for integrating RLD-like conditions into the brain network, which adds data about the actual properties twisting the picture.The most supportive conditions are reused throughout the organization, speeding up the educational experience. Consequently, the iterative conditions of RLD were incorporated into the brain organization to make RLN.
“We consider this methodology “directing” the brain organization’s way of learning,” which makes sense to Yicong Wu, Ph.D., a senior creator of the review. “Set forth clearly, the direction assists the organization in learning all the more quickly.”
Tests on pictures of undeveloped worms showed that RLN worked on both preparation and handling time, in contrast with other profound learning programs currently being used. The number of boundaries required to prepare the organization to use RLN was drastically reduced from several million to under 20,000.The handling time to get clear pictures of the developing organisms was also significantly reduced, with RLN requiring a couple of moments to determine a picture versus 20 seconds with a few minutes utilizing other well-known brain organizations.
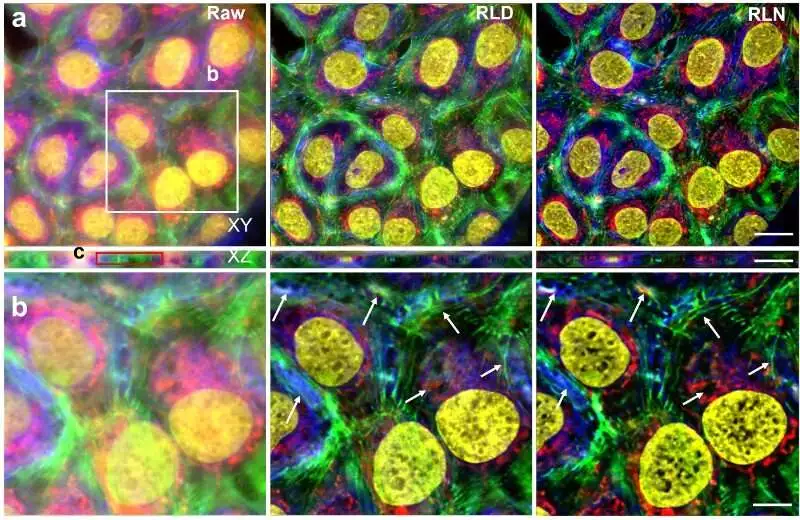
Wide view and close-up of crude pictures of human malignant growth cells handled with RLD and RLN Bolts in the nearby view show better detail in pictures handled with RLN. Credit: Li, et al. Nat Strategies 19, 1427-1437 (2022), Creative Commons Attribution 4.0 International Permit
In spite of the fact that RLN speeds up the preparation cycle, the fluffy and clear datasets expected to prepare the organization are hard to get or make without any preparation. To resolve the issue, the scientists ran RLD conditions backward to quickly make engineered informational collections for preparation. PC-produced pictures were made with a combination of dabs, circles, and circles—so-called blended engineered information. Obscure was added to the manufactured images based on estimates from out-of-center cells. Foundation commotion was additionally added to make hazy, uproarious pictures of the PC’s engineered shapes. The sets of clear and fluffy engineered blended shapes were used to prepare a brain organization for the reestablishment of genuine images of live cells.
The examination showed that RLN’s preparation of engineered information beat RLD in making clear pictures of the out-of-center cells. Amazingly, RLN cleared up many fine designs in the pictures that RLD neglected to distinguish.
“The success we are having with utilizing engineered data to prepare brain networks is extremely energizing,” said Hari Shroff, Ph.D., one of the review’s lead creators.”Making or acquiring informational indexes for preparation has been a colossal bottleneck in picture handling.” This blend of the discoveries in this work—that engineered information truly works, particularly when utilized with RLN—can possibly introduce another period in picture handling that we are enthusiastically chasing after.
The gathering is very amped up for one more part of the work. They found that engineered informational indexes made to reestablish pictures of a particular subject, for example, live cells, were likewise ready to reestablish fluffy pictures of totally different things like human minds. They depict this as the “generalizability” of manufactured preparation. The group is presently pushing forward at maximum speed to perceive how far such generalizability can be taken to speed up the production of great pictures for natural examination.
More information: Yue Li et al, Incorporating the image formation process into deep learning improves network performance, Nature Methods (2022). DOI: 10.1038/s41592-022-01652-7
Journal information: Nature Methods