Little atoms called immunomodulators can assist with making more powerful immunizations and more grounded immunotherapies to treat disease.
In any case, finding the atoms that affect the right safe reaction is troublesome—the quantity of medication like little particles has been assessed to be 1060, a lot higher than the quantity of stars in the noticeable universe.
In a likely first for the field of immunization configuration, AI directed the disclosure of new resistant pathway-upgrading particles and found one specific little particle that could beat the best immunomodulators available. The outcomes are distributed in the journal Synthetic Science.
“We utilized man-made reasoning techniques to direct a pursuit of a colossal substance space,” said Prof. Aaron Esser-Kahn, co-creator of the paper who drove the tests. “In doing so, we found particles with record-level execution that no human would have recommended we attempt. We’re eager to share the diagram for this cycle.”
“AI is utilized vigorously in drug plans; however, it doesn’t seem to have been recently utilized thusly for immunomodulator revelation,” said Prof. Andrew Ferguson, who drove the AI. “It’s a pleasant instance of moving devices, starting with one field, then onto the next.”
“We used artificial intelligence methods to guide a search of a vast chemical space, and as a result, we discovered molecules with record-breaking performance that no human would have suggested we try. We’re happy to provide the process blueprint.“
Said Prof. Aaron Esser-Kahn, co-author of the paper who led the experiments.
AI to screen atoms
Immunomodulators work by changing the flagging movement of inborn resistance pathways inside the body. Specifically, the NF-κB pathway plays a part in irritation and safe enactment, while the IRF pathway is fundamental in antiviral reactions.
Recently, the PME group directed a high-throughput screen that took a gander at 40,000 mixes of particles to check whether any impacted these pathways. They then, at that point, tried the top up-and-comers, finding that when those particles were added to adjuvants—fixtures that assist with supporting the safe reaction in immunizations—the particles expanded the immunizer reaction and decreased irritation.
To find more competitors, the group utilized these outcomes, joined by a library of almost 140,000 financially accessible little particles, to direct an iterative computational and exploratory cycle.
Graduate understudy Yifeng (Oliver) Tang utilized an AI strategy called dynamic realizing, which mixes both investigation and double-dealing, to explore exploratory screening through sub-atomic space productively. This approach gains from the information recently gathered and views potential high-performing particles as tentatively tried while likewise bringing up regions that have been under-investigated and may contain a few important competitors.
The cycle was iterative; the model brought up expected great competitors or regions where it required more data, and the group led a high-throughput investigation of those particles and afterward took care of the information back into the dynamic learning calculation.
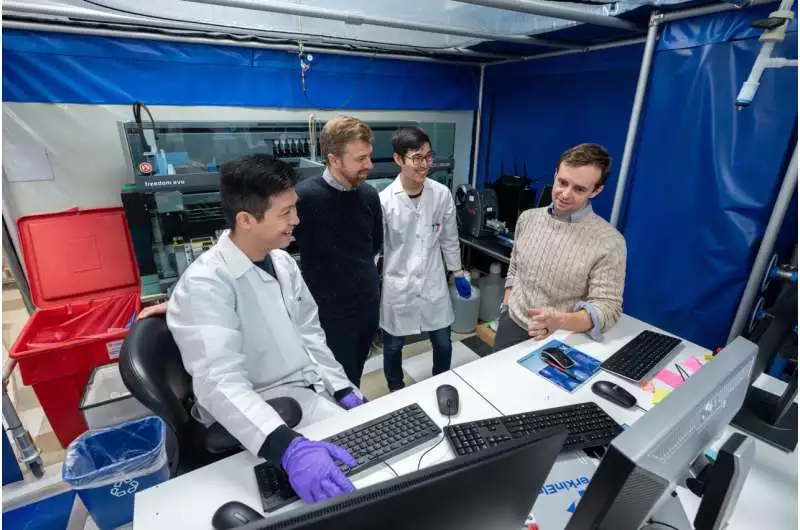
(From left): Graduate understudy Yifeng (Oliver) Tang, Assoc. Prof. Andrew Ferguson, graduate understudy Jeremiah Kim, and Prof. Aaron Esser-Kahn survey the consequences of the great throughput trial screening. Credit: Jason Smith for the College of Chicago
Particles that outflank the rest
After four cycles—and eventually testing just around 2% of the library—the group found high-performing little particles that had never been found. These top-performing competitors further developed NF-κB movement with every available ounce of effort, raised IRF action by 83%, and smothered NF-κB action by 128%.
One particle instigated a three-fold upgrade of IFN-β creation when conveyed with what’s known as a STING (trigger of interferon qualities) agonist. STING agonists advance more grounded, safe reactions inside growths and are a promising therapy for disease.
“The test with STING has been that you can’t get sufficient resistant action in the cancer, or you have askew movement,” Esser-Kahn said. “The particle we tracked down beat the best-distributed atoms by 20%.”
They likewise saw as a few “generalists” immunomodulators fit for changing pathways when co-conveyed with agonists, synthetic substances that initiate cell receptors to create an organic reaction. These little particles could at last be utilized in antibodies all the more comprehensively.
“These generalists could be great across all antibodies and hence could be more straightforward to bring to advertise,” Ferguson said. “That is very invigorating—that one particle could assume a multi-layered part.”
To all the more likely comprehend the particles found by AI, the group additionally distinguished normal synthetic highlights of the atoms that advanced beneficial ways of behaving. “That permits us to zero in on atoms that have these attributes, or sanely engineer new particles with these synthetic gatherings,” Ferguson said.
The group hopes to proceed with this cycle to look for additional particles and trust that others in the field will share datasets to make the hunt considerably more productive. They desire to evaluate particles for more unambiguous invulnerable movement, such as enacting specific white blood cells, or find a blend of atoms that gives them better control of the safe reaction.
“Eventually, we need to find particles that can treat infection,” Esser-Kahn said.
A group from the Pritzker School of Sub-atomic Designing (PME) at the College of Chicago handled the issue by utilizing AI to direct high-throughput trial screening of this tremendous pursuit space.
More information: Yifeng Tang et al. Data-driven discovery of innate immunomodulators via machine learning-guided high throughput screening, Chemical Science (2023). DOI: 10.1039/D3SC03613H