Individual cells’ coordinated functions determine the complexity of biological systems. Traditional methods for obtaining bulk genome or transcriptome data are incapable of revealing the cellular heterogeneity that drives this complexity. Single-cell sequencing is a method of next-generation sequencing (NGS) that examines the genomes or transcriptomes of individual cells to provide a high-resolution view of cell-to-cell variation.
Children’s Hospital of Philadelphia (CHOP) and New Jersey Institute of Technology (NJIT) researchers created new software that integrates a variety of data from a single cell, allowing researchers to see how one change in a cell can lead to several others and providing important clues for pinpointing the exact causes of genetic-based diseases.
Nature Communications published the findings.
Researchers can examine specific aspects of a cell to determine how it interacts with its microenvironment using single-cell sequencing. This is especially important in cancer research because it can be used to determine the effects of a mutation that only affects a small percentage of cells. At the single-cell level, researchers can study gene expression as well as messenger RNA, proteins, and even organelles within the cells in much greater detail and resolution than before.
With this tool, we can better understand a single cell as an entity and not just as a fragmented unit. This is a significant advancement and allows us to integrate and put all of this information into biological perspective, which is particularly important when considering information on different diseases.
Hakon Hakonarson
However, because each of a single cell’s characteristics has been studied separately, their connections with one another — for example, how a genetic variant might directly impact messenger RNA, protein synthesis, or epigenetics — may not be obvious, even when comparing data generated from the same cell.
To address this statistical and computational quandary, the researchers created an automated single-cell multimodal sequencing clustering software tool that can profile what is happening within the cell across multiple biological processes at the same time, allowing them to better characterize relationships between changes in a cell.
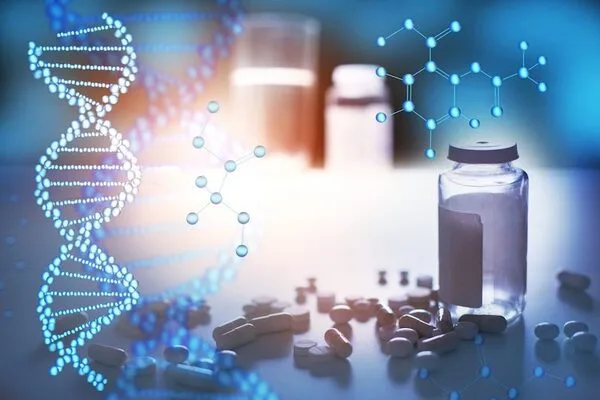
“With this tool, we can better understand a single cell as an entity and not just as a fragmented unit,” said Hakon Hakonarson, MD, Ph.D., director of the Center for Applied Genomics at CHOP and a senior author of the study. “This is a significant advancement and allows us to integrate and put all of this information into biological perspective, which is particularly important when considering information on different diseases.”
The software, referred to as single-cell multimodal deep clustering (scMDC), uses machine learning to analyze data about different characteristics of a single cell. The researchers conducted extensive simulation and real-data experiments and found that scMDC outperformed existing single-cell single-modal and multimodal clustering methods on single-cell multimodal data sets. It also utilizes linear scalability, meaning that more data sources provided to the scMDC yield better results.
Researchers can now investigate the distinct biology of individual cells in complex tissues and understand cellular subpopulation responses to environmental cues using highly sensitive ultra-low-input and single-cell RNA sequencing (RNA-Seq) methods. These assays help researchers better understand cell function and heterogeneity in time-dependent processes like differentiation, proliferation, and tumorigenesis.