Models that foresee traffic volume for explicit settings are utilized to illuminate all that, from traffic-signal examples to the application on your telephone that lets you know how to get from Point A to Point B. Scientists from North Carolina State University have now shown a strategy that decreases the computational intricacy of these models, causing them to work all the more productively.
“We use models to anticipate how much traffic there will be on some random stretch of street at a particular moment,” says Ali Hajbabaie, co-creator of a paper on the work and an associate teacher of common development and natural design at NC State. “These models function admirably, yet the particular determining questions can be so computationally complex that they are either difficult to tackle with restricted registering assets, or they take such a long time that the forecast possibly opens up when it is as of now not valuable.”
The analysts’ beginning stage for this work was a calculation intended to assist with smoothing out complex registering difficulties. However, they observed it couldn’t be applied straightforwardly to traffic issues.
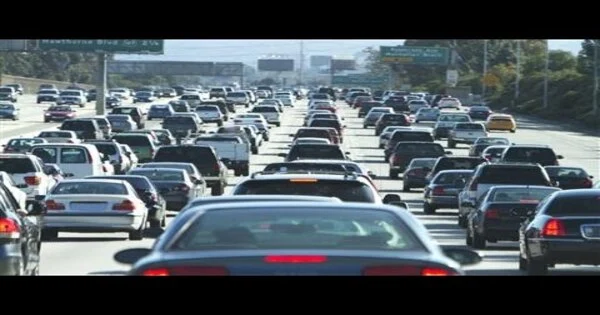
“Along these lines, we adjusted that calculation to check whether we could figure out how to involve it in models that anticipate how much traffic there will be in a particular spot and time,” Hajbabaie says. “Also, the outcomes were satisfying.”
In particular, the scientists concocted an adjusted variant of the calculation that successfully breaks the bigger traffic determining model into an assortment of more modest issues that can then be addressed in line with each other.
This cycle altogether decreases run time for the gauging model. Nonetheless, the level of superior proficiency varies greatly depending on how complex the anticipating questions are.The more complicated the inquiry is, the more prominent the better productivity.
The altered technique likewise works on run time by permitting the model to perceive when it has arrived at an adequate answer—the arrangement doesn’t need to be awesome. Customarily, models will run until they track down an ideal arrangement, or one extremely near ideal. Yet, for most purposes, an outcome that is within 5%—or even 10%—of the ideal arrangement will turn out great.
“Our methodology here basically sets blunder bars around the ideal arrangement and permits the model to quit running and report an outcome when it draws adequately near,” Hajbabaie says.
“Our modified algorithm outperformed the benchmark in two ways. First, our algorithm used far less computer memory. Second, our algorithm’s run time was orders of magnitude faster.
Hajbabaie
The scientists tried the altered calculation against a benchmark calculation utilized in buyer programming to resolve questions connected with traffic estimating.
“Our altered calculation beat the benchmark in two ways,” Hajbabaie says. In the first place, our calculation utilized undeniably less PC memory. Second, our calculation’s run time was significantly quicker.
“Now, we’re available to work with traffic organizers and architects who are keen on investigating how we can utilize this adjusted calculation to resolve certifiable issues.”
The paper shows up in IEEE Transactions on Intelligent Transportation Systems.