Deep learning and artificial intelligence bring about transformative changes, but they also come with enormous costs. OpenAI’s ChatGPT algorithm, for instance, has a daily operating cost of at least $100,000. Accelerators or computer hardware designed to efficiently carry out the particular operations of deep learning could lessen this. However, it is only possible to materially integrate such a device with common silicon-based computing hardware.
Until a team of researchers at the University of Illinois at Urbana-Champaign successfully integrated arrays of electrochemical random-access memory, or ECRAM, onto silicon transistors for the first time at the material level, this was preventing the implementation of an extremely promising deep learning accelerator. An ECRAM device designed and fabricated with materials that can be deposited directly onto silicon during fabrication was recently reported in Nature Electronics by the researchers, who were led by graduate student Jinsong Cui and professor Qing Cao of the Department of Materials Science & Engineering. This resulted in the realization of the first practical ECRAM-based deep learning accelerator.
“Ours is the first to achieve all these properties and be integrated with silicon without compatibility issues,” Cao stated. “Other ECRAM devices have been made with the many difficult-to-obtain properties needed for deep learning accelerators.” The final significant obstacle to the technology’s widespread use was this.”
“Our ECRAM devices will be most beneficial for AI edge-computing applications sensitive to chip size and energy consumption, That’s where this sort of device offers the most substantial benefits compared to what is feasible with silicon-based accelerators.”
Professor Qing Cao of the Department of Materials Science & Engineering.
ECRAM is a memory cell or gadget that stores information and utilizes it for computations in a similar physical area. This non-standard engineering figure dispenses with the energy cost of carrying information between the memory and the processor, permitting information-intensive activities to be performed productively.
By shifting mobile ions between a gate and a channel, ECRAM encodes data. The resulting change in the electrical conductivity of a channel stores information when electrical pulses are applied to a gate terminal. These pulses either draw ions into or inject ions into a channel. It is then analyzed by estimating the electric flow that streams across the channel. ECRAM can store data as a non-volatile memory because an electrolyte prevents unwanted ion flow between the gate and the channel.
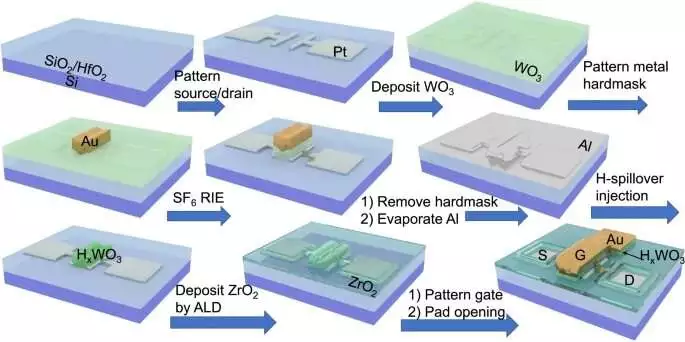
ECRAM manufacturing process Credit: Electronics in Nature (2023) DOI: The following materials were chosen by the research team to be compatible with silicon microfabrication methods: tungsten oxide for the entryway and channel, zirconium oxide for the electrolyte, and protons as the versatile particles. As a result, the devices were able to be integrated into and controlled by standard microelectronics. Other ECRAM devices utilize organic materials or lithium ions, both of which are incompatible with silicon microfabrication, and draw inspiration from neurological processes or even rechargeable battery technology.
Furthermore, the Cao bunch gadget has various different highlights that make it ideal for profoundly learning gas pedals. ” “An ideal memory cell must achieve a whole slew of properties, even though silicon integration is essential,” Cao stated. Numerous other desirable characteristics result from the materials we chose.”
Because the gate and channel terminals were made of the same material, injecting ions into the channel and drawing ions out of the channel are symmetric operations, which make the control scheme easier to understand and significantly improve reliability. The channel held ions for hours at a time, which is enough for most deep neural network training. Since the particles were protons, the gadgets exchanged quickly with the smallest particle. The researchers discovered that their devices were significantly more efficient than standard memory technology and lasted for more than 100 million read-write cycles. Finally, because the materials are compatible with techniques for microfabrication, the devices could be reduced to the nano- and micro-scales, enabling high density and computing power.
By fabricating arrays of ECRAMs on silicon microchips to perform matrix-vector multiplication, a mathematical operation essential to deep learning, the researchers demonstrated their device. The array multiplied the vector inputs, which were represented as applied voltages, by altering the resulting currents using matrix entries, which were neural network weights stored in the ECRAMs. A high degree of parallelism was used for both this operation and the weight update.
According to Cao, “AI edge-computing applications sensitive to chip size and energy consumption will be the most useful for our ECRAM devices.” When compared to accelerators based on silicon, this type of device offers the greatest advantages in this area.
The new device is protected by a patent, and partners from the semiconductor industry are helping the researchers bring this new technology to market. Cao says that autonomous vehicles, which have to learn their surroundings quickly and make decisions with limited computational resources, are a prime application of this technology. Cao is working with Illinois faculty in computer science and electrical and computer engineering to develop software and algorithms that take advantage of the ECRAM’s unique capabilities and integrate their ECRAMs with silicon chips made in a foundry.
More information: Jinsong Cui et al, CMOS-compatible electrochemical synaptic transistor arrays for deep learning accelerators, Nature Electronics (2023). DOI: 10.1038/s41928-023-00939-7
Journal information: Nature Electronics