A new, fast method for determining the location, size, and category of multiple objects has been developed by researchers, and it does not necessitate the acquisition of images or the complex reconstruction of a scene. It’s possible that the new method could be useful for identifying dangers while driving because it significantly reduces the amount of computing power required for object detection.
“Our procedure depends on a solitary pixel identifier, which empowers proficient and strong multi-object identification straightforwardly from a few 2D estimations,” said research group pioneer Liheng Bian from the Beijing Foundation of Innovation in China. “It is anticipated that this kind of image-free sensing technology will resolve the issues of existing visual perception systems’ low perception rate, high computing overhead, and heavy communication load.
Classification, single-object recognition, and tracking are the only outcomes that can be achieved using image-free perception techniques today. The researchers devised an approach known as image-free single-pixel object detection (SPOD) in order to accomplish all three at once. They report in Optics Letters that SPOD has an object detection accuracy of just over 80%.
“The compact, optimized pattern delivers greater image-free sensing performance than the full-size pattern utilized by other single-pixel detection approaches, according to the study.”
Research team leader Liheng Bian from the Beijing Institute of Technology in China.
The SPOD method builds on the group’s previous successes in developing efficient imaging-free sensing technology for scene perception. Single-pixel detector-based image-free classification, segmentation, and character recognition are among their previous accomplishments.
Bian stated, “For autonomous driving, SPOD could be used with lidar to help improve object detection accuracy and speed of scene reconstruction.” We believe it has a detection rate and accuracy sufficient for autonomous driving and reduces the amount of transmission bandwidth and computing resources required for object detection.”
Detection without images
Automating complex visual tasks, such as navigating a vehicle or tracking a moving plane, typically necessitates taking precise pictures of the scene in order to extract the characteristics required to identify an object. Notwithstanding, this requires either complex imaging equipment or confounded remaking calculations, which prompts high computational expense, a long running time, and a weighty information transmission load. Consequently, the customary picture first, see later methodologies may not be best for object recognition.
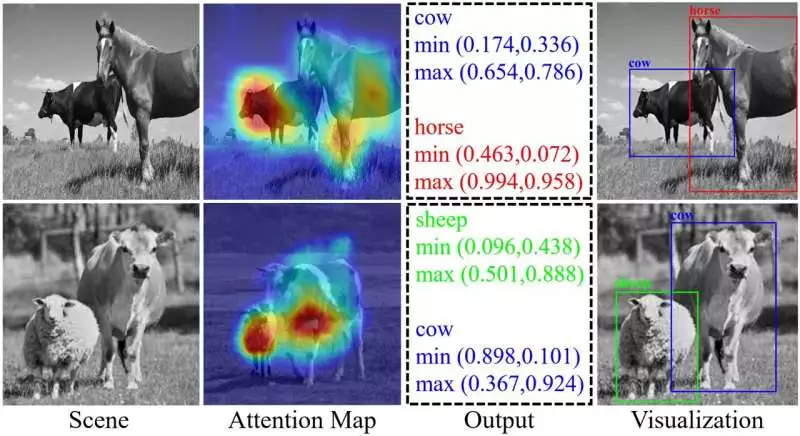
The scientists showed that SPOD accomplishes a discovery exactness of 82.41% at a testing pace of 5% with a revive pace of 63 FPS. The visualization’s outcomes are depicted. Credit: Lintao Peng, Beijing Institute of Technology
Image-free sensing techniques employing single-pixel detectors have the potential to reduce the amount of computational power required for object detection. Rather than utilizing a pixelated indicator, for example, a CMOS or CCD, single-pixel imaging enlightens the scene with a succession of organized light examples and afterward records the communicated light power to secure the spatial data of items. This data is then used to computationally remake the item or work out its properties.
For SPOD, the researchers quickly scanned the entire scene and took 2D measurements with a small but optimized structured light pattern. To extract the scene’s high-dimensional meaningful features, these measurements are fed into a transformer-based encoder deep learning model. A multi-scale attention network-based decoder based on these features then outputs the class, location, and size information for all of the scene’s targets simultaneously.
Lintao Peng, a member of the group, stated, “Compared to the full-size pattern used by other single-pixel detection methods, the small, optimized pattern produces better image-free sensing performance.” Additionally, the SPOD decoder’s multi-scale attention network keeps its focus on the scene’s target area. This enables cutting-edge object detection performance and more effective scene feature extraction.
Proof-of-concept demonstration
A proof-of-concept setup was constructed by the researchers in order to experimentally demonstrate SPOD. Film prints of randomly chosen images from the Pascal Voc 2012 test dataset were used as target scenes. Spatial light modulation and image-free object detection with SPOD took an average of just 0.016 seconds per scene at a sampling rate of 5%.
This is much faster than first detecting objects (0.018 seconds) and then reconstructing the scene (0.05 seconds). SPOD showed a typical location precision of 82.2% for all the article classes remembered for the test dataset.
Peng stated, “The existing object detection dataset used to train the model only contains 80 categories, so SPOD cannot currently detect every possible object category.” However, the pre-trained model can be fine-tuned for specific tasks to achieve image-free multi-object detection of new target classes for applications like boat, vehicle, or pedestrian detection.”
Then, the analysts intend to expand the picture of free insight innovation to different sorts of locators and computational obtaining frameworks to accomplish remaking free detection innovation.
More information: Lintao Peng et al, Image-free single-pixel object detection, Optics Letters (2023). DOI: 10.1364/OL.486078
Journal information: Optics Letters