Researchers have fostered another AI stage that makes the calculations that control molecule bars and lasers more intelligent than any time in recent memory. Their work could help lead to the advancement of better than ever molecule gas pedals that will assist researchers with unlocking the privileged insights of the subatomic world.
Daniele Filippetto and partners at the Department of Energy’s Lawrence Berkeley National Laboratory (Berkeley Lab) fostered the arrangement to naturally make up for constant changes to gas pedal pillars and different parts, like magnets. Their AI approach is also superior to current bar control frameworks in terms of understanding why things fail and utilizing material science to plan a response.A paper portraying the examination was distributed toward the end of last year in Nature Scientific Reports.
“We are attempting to teach material science to a chip, while simultaneously giving it the insight and experience of a senior researcher working on the machine,” said Filippetto, a staff researcher at the Accelerator Technology and Applied Physics Division (ATAP) at Berkeley Lab and delegate overseer of the Berkeley Accelerator Controls and Instrumentation Program (BACI) program.
“I come from an accelerator background, my colleagues really inspired me to appreciate the power of machine learning during my post-doc. I’ve learnt that machine learning is a wonderful instrument to address a variety of problems, but you always have to use your physics to guide in how you use and use it,”
Dan Wang, a research scientist in the BACI group
Their exploration can likewise affect numerous applied fields of molecular gas pedals, going from independent activities in modern and clinical settings to expanded accuracy in logical applications like direct colliders and ultrafast free electron lasers.
The original method was exhibited at the High Repetition-Rate Electron Scattering Apparatus (HiRES) gas pedal at Berkeley Lab as a team with specialists from Los Alamos National Laboratory and UCLA. The HiRES beamline is primarily used to investigate the underlying elements of novel quantum materials.The instrument has added to various logical disclosures, for example, playing out the very first ultrafast electron diffraction investigations of optical liquefying of tantalum ditelluride, a material with intriguing and possibly helpful properties. Presently, this clever machine is showing its handiness in fostering new techniques for controlling expansive classes of gas pedals.
Molecule gas pedals produce and speed up light emission particles, like electrons, protons, and particles of nuclear and subatomic size. As machines become more remarkable and complex, control and enhancement of the molecule or laser shaft become increasingly important in addressing issues of logic, clinical, and modern applications.
Filippetto and associates at the BACI program are driving the worldwide improvement of AI equipment. These instruments give a stage to foster shrewd calculations that respond rapidly and definitively to unexpected perturbances, gain from their slip-ups, and take on the best technique for coming to or keeping up with the objective pillar setpoint.
The instruments they are creating enjoy the additional benefit of giving an exact model of the general behavior of an atom smasher framework, regardless of the intricacy. Regulators can use these improved capabilities to make more compelling consistent decisions.
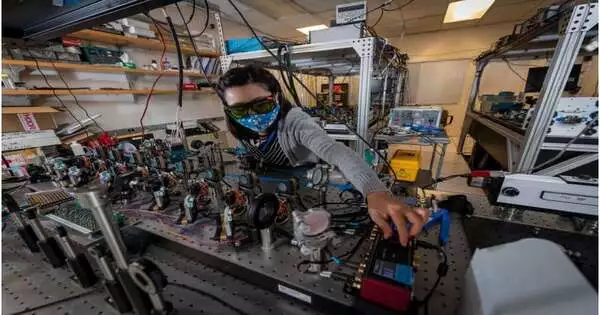
Early Career Research Scientist Dan Wang is chipping away at the piezo dormancy engine regulators to drive piezo mirrors for laser arrangement in the rational laser consolidating framework. Thor Swift / Berkeley Lab
The current focal point of Filippetto’s work is utilizing the power and forecast of AI instruments to expand the general solidity of molecule radiation.
“In the event that you can foresee the bar properties with a precision that outperforms their variances, you can then utilize the forecast to build the exhibition of the gas pedal,” he said. “Constant information on key bar boundaries would gigantically affect the precision of investigations.”
Right away, such a methodology could appear to be not going to create precise outcomes, like difficulties with securities exchange conduct forecast, but early outcomes from the gathering are promising. As a matter of fact, the calculation utilized, which depends on brain network models, shows a ten-fold expansion in the accuracy of anticipated bar boundaries compared with commonplace measurable examination. In related work, a new Halbach grant went to Simon Leemann, staff researcher in the Accelerator Physics Group in ATAP, and teammates for creating AI control techniques that work on the exhibition of the Advanced Light Source by settling the exceptionally relativistic electron bar at the trial source focus by about one significant degree, a remarkable level.
In related research published in Optics Express, Dan Wang, an exploration researcher in the BACI group who started her profession at Berkeley Lab quite a while back as a post-doctoral scientist, is utilizing AI devices to propel the innovation of control in complex laser frameworks. For Wang’s situation, a definitive objective is to have the option to precisely join many super serious laser beats into one strong and sound shaft the size of a human hair. In a rational bar, the period of each information laser should be controlled within a couple of levels of error, which is extremely difficult. The laser energy can be joined in various ways, yet in all cases, the lucidity of the shaft must be settled against ecological annoyances like warm float, air changes, or even the development of the supporting table.
To do this, Wang and her partners fostered a brain network model that is multiple times quicker at rectifying framework blunders in the joined laser cluster than other regular techniques. The model they created is likewise equipped to help the framework perceive stage mistakes and boundary changes in the lasers and to autocorrect for annoyances when they happen.
The analysts’ technique worked in the two recreations and tests in lasers, where phenomenal control execution was accomplished. The following stage in the examination is to execute AI models anxious PCs, for example, field programmable door clusters (FPGAs) for quicker reaction, and furthermore to exhibit the speculation of this AI based control technique in additional perplexing frameworks where there are undeniably more factors to represent.
“I come from a gas pedal foundation, yet during my post-doc, my partners truly assisted me in embracing the force of AI,” Wang said. “What I’ve discovered is that AI is an integral asset to take care of various issues, yet you generally need to utilize your material science to direct how you use and apply it.”
“To address the issues of new science, this work represents dynamic input and AI techniques that are urgent empowering agents for the up and coming age of gas pedal and laser execution to control new photon sources and future molecule colliders,” said Cameron Geddes, overseer of the Accelerator Technology and Applied Physics Division.
More information: Alexander Scheinker et al, An adaptive approach to machine learning for compact particle accelerators, Scientific Reports (2021). DOI: 10.1038/s41598-021-98785-0