AI propulsions have recently enabled the advancement of computational devices that can create convincing yet falsely created texts, also known as “deepfake texts.”While the programmed creation of texts could make them interesting for applications, it likewise brings serious concerns regarding security and deception to the surface.
Artificially generated texts could eventually be used to deceive web clients, for example, through a wide range of fanatics or rough texts aimed at radicalizing people, fake news for disinformation crusades, email texts for phishing assaults, or phony audits focusing on specific inns, scenes, or cafés.All in all, this could additionally lessen a few clients’ confidence in web-based content while provoking different clients to take part in an unsocial and unsafe way of behaving.
A new report driven by scientists at Virginia Tech, in collaboration with specialists at the College of Chicago, LUMS Pakistan, and the College of Virginia, has of late investigated the limits and qualities of existing methodologies for identifying deep-fake texts. Their paper, with understudies Jiameng Pu and Zain Sarwar as lead creators, is set to be introduced at IEEE S&P’23, a meeting zeroing in on PC security.
“While these systems showed high detection accuracies, it was unknown how well they would perform in practice, in adversarial environments. Existing countermeasures were tested on datasets manufactured by researchers rather than synthetic data collected in the field. In actuality, attackers would adapt to these protections in order to avoid detection, and previous efforts did not account for such adversarial scenarios.”
Bimal Viswanath, researcher from Virginia Tech who led the study.
“A large part of the security research we led before 2016 expected an algorithmically frail aggressor.” This supposition is not generally legitimate given the advances made in man-made intelligence and ML. We need to consider algorithmically keen or ML-fueled foes. This incited us to begin investigating this space. “In 2017, we published a paper investigating how language models (LMs) like RNNs can be abused to create fake surveys on stages like Cry,” Bimal Viswanath, a Virginia Tech scientist who led the review, told TechXplore.
“This was our initial introduction to this space. From that point forward, we saw rapid advances in LM advancements, particularly after the introduction of the Transformer family of models.These advances raise the danger of abusing such devices to empower huge-scope missions to spread disinformation, create assessment spam and harmful substances, and develop more viable phishing methods.
Throughout recent years, numerous PC researchers overall have been attempting to foster computational models that can precisely identify engineered text created by cutting-edge LMs. This prompted the presentation of various different guarded systems, remembering some that search out unambiguous curiosities for engineered messages and others that depend on the utilization of pre-prepared language models to assemble finders.
“While these guards detailed high location exactnesses, it was still unclear how well they would function in the long run, under antagonistic settings,” Viswanath explained.”Existing guards were tested on datasets created by analysts rather than engineered information in nature.”Assailants would eventually adjust to these guards to avoid detection, and existing works did not account for such ill-advised settings.
Guards that noxious clients can undoubtedly beat by slightly altering their language models’ plans are finally ineffective in reality.Viswanath and his partners hence set off to investigate the limits, qualities, and true worth of probably the most encouraging deepfake text location models made up to this point.
Their paper zeroed in on six existing engineered text-location plans presented throughout recent years, all of which had achieved amazing exhibitions in starting assessments, with discovery exactnesses ranging from 79.6% to 98.5%. The models they assessed are BERT-Guard, GLTR-GPT2, GLTR-BERT, GROVER, Quick, and RoBERTa-Safeguard.
“We thank the designers of these models for imparting code and information to us, as this permitted us to precisely repeat them,” Viswanath said. “Our most memorable goal was to consistently assess the performance of these guards on real-world datasets.”To do this, we arranged for four novel engineered datasets, which we have delivered to the local area.
Viswanath and his colleagues gathered a large number of engineered text articles created by various text-age as-a-administration stages, as well as deepfake Reddit posts created by bots, to organize their datasets.Text-age as-a-service stages are man-made intelligence-fueled web locales that permit clients to just make engineered text, which can be abused to make deceptive content.
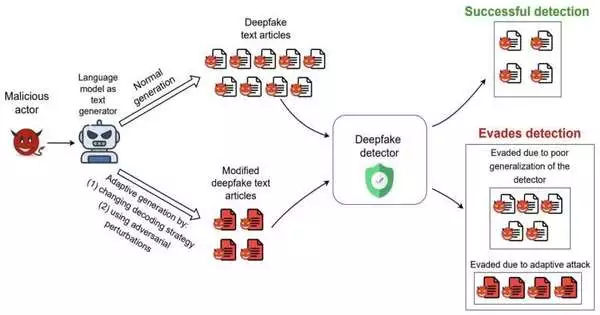
To dependably survey the exhibition of the six guard models they chose in identifying deepfake texts, the scientists proposed a progression of “minimal expense” avoidance systems that just expect changes to the LM-based text generator at deduction time. This essentially implies that the LM creating the phony message can be adjusted or improved during preliminary work without the requirement for extra preparation.
“We likewise proposed a clever avoidance system, called DFTFooler, that can naturally bother or change any engineered text article to dodge location while saving the semantics,” Viswanath said. “DFTFooler makes use of freely available LMs and employs novel approaches to the engineered text location problem.”Unlike other ill-conceived bother plans, DFTFooler does not require inquiry access to the casualty guard classifier to make sly examples, making it a more covert and useful assault device.
The group’s assessments yielded a few intriguing outcomes. The analysts, right off the bat, found that the exhibition of three out of the six guard models they surveyed altogether declined when they were tried on genuine world datasets, with 18% to almost 100% drops in their precision. This emphasizes the importance of working on these models to ensure that they sum up well across various pieces of information.
Moreover, Viswanath and his partners found that changing a LM’s text unraveling (i.e., text testing) system frequently broke large numbers of the guards. This basic system requires no extra model preparation, as it just changes a LM’s current text age boundaries and is hence simple for assailants to uphold.
“We likewise find that our new antagonistic text control system, called DFTFooler, can effectively make sly examples without requiring any questions to the safeguard’s classifier,” Viswanath said. “Among the six guards we assessed, we find that one safeguard called Quick is strongest in these ill-disposed settings, compared with other protections.” Sadly, Quick has an intricate pipeline that utilizes various high-level NLP methods, making it harder to grasp its better exhibition.
To acquire knowledge about the characteristics that make the Quick model especially strong and solid in recognizing deep-fake texts, the scientists led a top-to-bottom examination of its elements. They observed that the model’s strength is due to its utilization of semantic elements removed from the articles.
Conversely, with the other guard models assessed in this review, Quick examines a text’s semantic elements, checking out named substances and relations between these elements in the text. This novel feature appeared to be effective in the model’s display of genuine world deepfake datasets.
Roused by these discoveries, Viswanath and his partners made DistilFAST, a worked-on form of Quick that just dissects semantic elements. They found that this model beat the first Quick model under ill-disposed settings.
“Our work features the potential for semantic elements to empower adversarially-hearty engineered location plans,” Viswanath said. “While Quick shows promise, there is still a lot of room for improvement.”Creating semantically consistent, long text articles is still a challenge for LMs.Hence, contrasts in the portrayal of semantic data in engineered and genuine articles can be taken advantage of to assemble hearty guards.
While attempting to dodge deepfake text finders, assailants generally do not have the option to change the semantic substance of engineered texts, especially when these texts are intended to convey explicit thoughts. Later on, the discoveries assembled by this group of analysts and the worked on Quick model they made could hence assist with fortifying guards against engineered texts on the web, possibly restricting huge scope disinformation or radicalization crusades.
“At the moment, this course has not been investigated in the security community,” Viswanath added. “In our future work, we intend to use information charts to remove more extravagant semantic elements, ideally creating more performant and hearty guards.”
More information: Jiameng Pu et al, Deepfake Text Detection: Limitations and Opportunities, arXiv (2022). DOI: 10.48550/arxiv.2210.09421
Yuanshun Yao et al, Automated Crowdturfing Attacks and Defenses in Online Review Systems, Proceedings of the 2017 ACM SIGSAC Conference on Computer and Communications Security (2017). DOI: 10.1145/3133956.3133990
Journal information: arXiv