Picture two groups getting down to business on a football field. The players can coordinate to accomplish a goal and go up against different players with clashing interests. That is the way the game works.
Making man-made reasoning specialists that can figure out how to contend and coordinate as well as people remains a prickly issue. A key test is empowering man-made intelligence specialists to expect future ways of behaving from different specialists when they are advancing all the while.
Due to the intricacy of this issue, current methodologies will generally be nearsighted; the specialists can figure out the following couple of moves of their partners or rivals, which prompts a horrible showing over the long haul.
Scientists from MIT, the MIT-IBM Watson man-made intelligence Lab, and somewhere else have fostered another methodology that gives man-made intelligence specialists a farsighted viewpoint. Their AI system enables helpful or serious artificial intelligence specialists to consider what other specialists will truly do as time approaches infinity, not just a couple of stages later.The specialists then adjust their ways of behaving likewise to impact other specialists’ future ways of behaving and show up at an ideal, long-haul arrangement.
“When AI agents cooperate or compete, what counts most is when their actions coincide in the future. There are many temporary behaviors along the route that don’t matter much in the long term. We genuinely care about achieving this convergent behavior, and we now have a mathematical means to do it.”
Dong-Ki Kim, a graduate student in the MIT Laboratory for Information and Decision Systems (LIDS)
This system could be utilized by a gathering of independent robots cooperating to track down a lost climber in thick woods or by self-driving vehicles that endeavor to guard travelers by anticipating the moves of other vehicles driving on a bustling roadway.
“Whenever man-made intelligence specialists are coordinating or contending, what makes the biggest difference is the point at which their ways of behaving eventually meet.” There are a ton of transient ways of behaving en route that don’t make any difference, especially over the long haul. “Attaining this met conduct is what we truly care about, and we presently have a numerical method for empowering that,” says Dong-Ki Kim, an alumni understudy in the MIT Lab for Data and Choice Frameworks (Tops) and lead creator of a paper depicting this system.
In this demo video, the red robot, which has been prepared utilizing the analysts’ AI framework, can overcome the green robot by learning more powerful ways of behaving that exploit the continually changing system of its rival.
More specialists, more issues
The scientists zeroed in on an issue known as multiagent support learning. Support learning is a type of AI where a man-made intelligence specialist advances through experimentation. Scientists give the specialist a prize for “great” ways of behaving that assist it in accomplishing an objective. The expert adjusts its behavior to increase the prize until it eventually becomes a specialist at an errand.
However, when a large number of helpful or competing experts are all learning at the same time, things become increasingly complicated.As specialists consider their colleagues’ future advancements and what their own behavior means for others, the issue quickly becomes one that necessitates a significant amount of computational ability to resolve.To this end, different methodologies just spotlight the present moment.
“The AIs truly need to ponder the finish of the game, yet they don’t have any idea when the game will end.” They must consider how to continue to adjust their behavior into vastness in order to succeed in the future.”Our paper basically proposes another objective that empowers a man-made intelligence to ponder vastness,” says Kim.
Yet, since it is difficult to plug vastness into a calculation, the scientists planned their framework so specialists center around a future place where their conduct will meet with that of other specialists, known as “balance.” A balance point determines the drawn-out exhibition of specialists, and various equilibria can exist in a multiagent situation.
As a result, a viable specialist effectively influences the future behaviors of other specialists, causing them to reach a beneficial balance from the specialist’s perspective.Assuming all specialists impact one another, they contribute to an overall idea that the scientists call a “functioning balance.”
The AI system they created, known as FURTHER (which stands for Completely Supporting Dynamic Impact with Normal Award), empowers specialists to figure out how to adjust their ways of behaving as they connect with different specialists to accomplish this dynamic harmony.
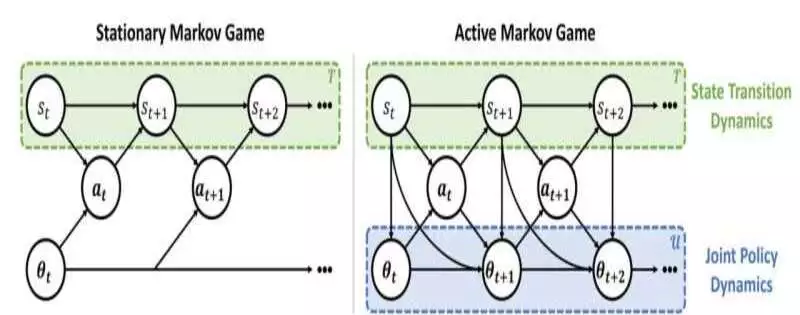
Inside the fixed Markov game setting, specialists wrongly expect that different specialists will have fixed approaches to what’s to come. Conversely, specialists in a functioning Markov game perceive that different specialists have non-fixed strategies in view of the Markovian update capabilities.
This also makes use of two AI modules. The initial module, a surmising module, empowers a specialist to figure out the future ways of behaving of different specialists and the learning calculations they use, dependent exclusively upon their earlier activities.
This data is taken care of in the support learning module, which the specialist uses to adjust its way of behaving and impact different specialists in a manner that boosts its value.
“The test was pondering vastness.” “We needed to utilize many numerical devices to empower that and make a few suspicions to inspire it to work by and by,” Kim says.
Winning over the long haul
They tried their methodology against other multiagent support learning systems in a few unique situations, including a couple of robots battling sumo-style and a fight pitting two 25-specialist groups against each other. In the two cases, the man-made intelligence specialists utilizing Additionally dominated the matches more regularly.
Kim understands that their methodology is more versatile than other techniques that require a central PC to control the specialists because it is decentralized, which means the specialists figure out how to dominate the matches freely.
The analysts utilized games to test their methodology, but FURTHER could be utilized to handle any sort of multiagent issue. For example, it very well may be applied by market analysts trying to foster sound strategy in circumstances where many connecting entities have ways of behaving and interests that change over the long haul.
Financial aspects are one application Kim is especially excited to examine. He likewise needs to dig further into the idea of a functioning harmony and keep improving the system.
The examination paper is accessible on arXiv.
More information: Dong-Ki Kim et al, Influencing Long-Term Behavior in Multiagent Reinforcement Learning, arXiv (2022). DOI: 10.48550/arxiv.2203.03535
Journal information: arXiv