Edge computing is a distributed computing system that puts enterprise applications closer to data sources such as Internet of Things (IoT) devices or local edge servers. It is a distributed information technology (IT) architecture in which client data is processed at the periphery of the network, as close to the originating source as possible. This proximity to data at its source can result in significant business benefits such as faster insights, faster response times, and increased bandwidth availability.
The University of Surrey’s researchers have successfully proven proof-of-concept for employing their multimodal transistor (MMT) in artificial neural networks that imitate the human brain. This is a significant step toward employing thin-film transistors as artificial intelligence hardware, and it advances edge computing by reducing power requirements and improving efficiency rather than relying entirely on computer processors.
The MMT, which was first disclosed by Surrey researchers in 2020, overcomes long-standing transistor difficulties and can perform the same tasks as more sophisticated circuits. This new study, published in the peer-reviewed journal Scientific Reports, employs mathematical modeling to demonstrate the concept of employing MMTs in artificial intelligence systems, which is an important step toward manufacturing.
There is a huge need for technological advancements to promote the expansion of low-cost, large-area electronics, which have been proved to be used in artificial intelligence applications. Thin-film transistors play a role in achieving high processing power while using few resources.
Isin Pesch
The researchers demonstrate, using measured and simulated transistor data, that well-designed multimodal transistors can operate reliably as rectified linear unit-type (ReLU) activations in artificial neural networks, attaining almost comparable classification accuracy as pure ReLU implementations. They trained an artificial neural network to recognize handwritten numbers using both measured and simulated MMT data and compared the results to the software’s built-in ReLU. The results validated MMT devices’ promise for thin-film decision and classification circuits. The similar strategy might be applied to more complicated AI systems.
Surprisingly, the study was led by a Surrey undergraduate, Isin Pesch, who worked on the project as part of her final year research module for her BEng (Hons) in Electronic Engineering with Nanotechnology. Covid required her to study remotely from her home in Turkey, but she was able to drive the development with the help of an international research team that comprised researchers from the University of Rennes in France and UCL in London.
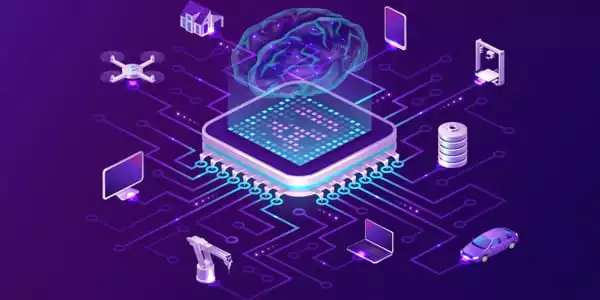
Isin Pesch, main author of the article, which was published before she graduated in July 2021, stated: “There is a huge need for technological advancements to promote the expansion of low-cost, large-area electronics, which have been proved to be used in artificial intelligence applications. Thin-film transistors play a role in achieving high processing power while using few resources. We can now see that MMTs, a unique sort of thin-film transistor created at the University of Surrey, have the dependability and uniformity required for this purpose.”
The tremendous rise and increased computational capacity of IoT devices has resulted in previously unheard-of data volumes. And data volumes will continue to rise as the number of mobile devices linked to 5G networks increases.
Previously, the promise of cloud and AI was to automate and accelerate innovation by generating actionable insights from data. However, the tremendous volume and complexity of data generated by connected devices has exceeded network and infrastructure capacities.
Dr Radu Sporea, Senior Lecturer at the Advanced Technology Institute at the University of Surrey, stated: “These findings serve as a reminder of Surrey’s position as a pioneer in AI research. Many of my colleagues are interested in people-centered AI and how to maximize human advantages, including how to apply these new notions ethically. The Advanced Technology Institute’s research advances physical implementation as a step toward powerful yet economical next-generation electronics. It’s amazing that teamwork is yielding such results, with academics at all levels collaborating, from undergraduates like Isin, who led this project, to seasoned professionals.”
Edge computing enables you to realize the full potential of the massive amounts of untapped data generated by connected devices. You can discover new business opportunities, improve operational efficiency, and provide your consumers with faster, more dependable, and consistent experiences. By evaluating data locally, the best edge computing models can help you accelerate performance. A well-thought-out approach to edge computing can keep workloads up to date in accordance with set standards, help protect privacy, and conform to data residency laws and regulations.