Energy units, essential parts of hydrogen power module electric vehicles (FCEVs), act as eco-accommodating energy transformation frameworks that create electric power and intensity through the substance responses of hydrogen and oxygen. Korean nearby legislatures are excited about putting resources into significant gear to extend the FCEV market, yet they frequently face resistance from neighborhood inhabitants. To ease residents’ interests, it is important to lay out a framework that can guarantee both the efficiency and security of hydrogen energy units.
The Korea Exploration Organization of Norms and Science has fostered an innovation for the constant discovery of miniature deformities on the power device surface during the creation cycle.
The recently evolved innovation depends on profound learning and empowers continuous 3D estimation. It can distinguish surrenders on a superficial level in a single shot, permitting constant checking of item quality without intruding on the assembling system.
“This method may be utilized to inspect various problems and defects in metal fuel cell separators in real time. It can optimize performance while also enhancing the reliability and security of the fuel cells now being manufactured.”
Dr. Young-Sik Ghim, the leader of the Optical Imaging and Metrology Team.
A solitary shot design projection technique is utilized for the continuous 3D estimation of the surface shape. The light with a thick composite framework design is projected onto the outer layer of an item, and afterward, the distorted example upon reflection is examined to get 3D data about imperfections or harms.
Nonetheless, one impediment to this philosophy is that it can’t quantify surfaces with low reflectivity or different blended designs. For example, the metal separator, which is a center part of power modules, is trying to be investigated utilizing continuous 3D estimation since it is made of treated steel (SUS) with a lopsided surface.
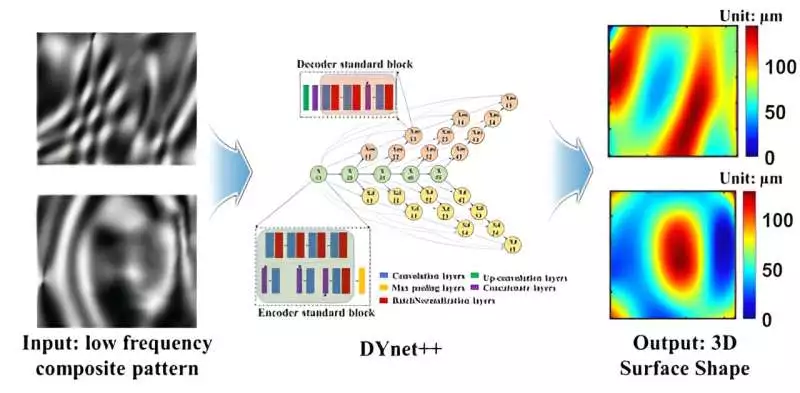
The 3D shapes anticipated by the prepared DYnet++ organization Credit: Korea Exploration Foundation of Norms and Science (KRISS)
To conquer this impediment, the Optical Imaging and Metrology Group of KRISS presented a man-made brainpower (artificial intelligence) calculation for the example projection technique. They utilized DYnet++, another profound learning network created by KRISS, which was prepared with estimation information on a huge number of surface shapes. This permits DYnet++ to perform ongoing 3D morphology estimations of surfaces with low reflectivity or complex shapes.
To apply this innovation to energy unit tests, the exploration group additionally prepared the computer-based intelligence calculation utilizing information on metal separators with surface deformities. The 3D morphology estimation results show that imprints and scratches on the example surfaces, which are challenging to set in stone through traditional 2D review, are effectively distinguished in a single shot on the grounds that the simulated intelligence calculation procured the application capacities even with a modest quantity of information.
A large amount of picture information with erratic complex examples and their comparing 3D shapes is gained and used to prepare the planned DYnet++ organization. Move learning is utilized for the examination of information obtained under the new ecological circumstances, which requires only one–10th of the amount of information needed to change the prepared model compared with what was utilized to assemble the underlying calculation. In view of the changed model, the 3D estimation results for different complex examples can be anticipated.
The innovation created through this exploration can be effectively applied to the creation line, no matter what the shape or size of the subject to be estimated. It empowers programmed examination of deformities in any event, during an assembly cycle with outside vibrations and critical temperature changes. This innovation can add to the presentation of brilliant processing plants in different regions of the assembling business, including energy components, by expanding efficiency, further developing item quality, and reducing costs.
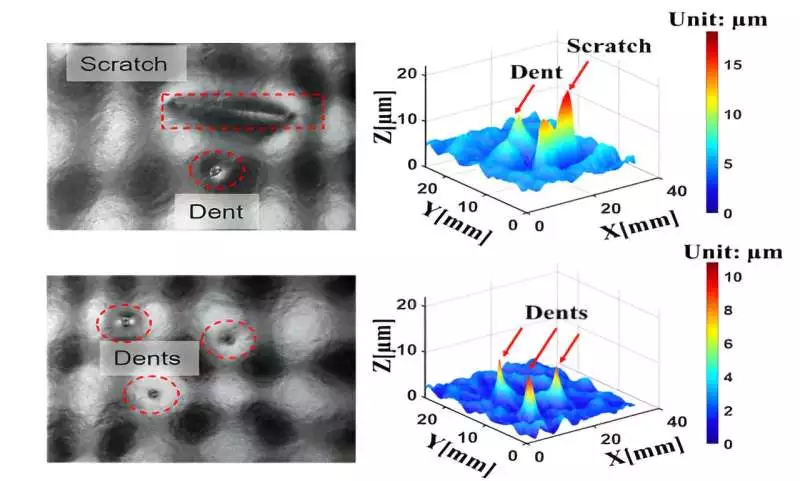
Estimation consequences of a power device test with a harsh surface and low reflectivity Credit: Korea Exploration Organization of Norms and Science (KRISS)
Dr. Youthful Sik Ghim, the head of the Optical Imaging and Metrology Group, expressed, “This innovation can be utilized for an ongoing review of different blames and deformities in energy component metal separators. It can augment execution as well as work on the toughness and security of the energy units that are at present being created.”
The paper is distributed in the journal IEEE Exchanges on Modern Hardware.
More information: Manh The Nguyen et al, DYnet++: A Deep Learning Based Single-Shot Phase-Measuring Deflectometry for the 3-D Measurement of Complex Free-Form Surfaces, IEEE Transactions on Industrial Electronics (2023). DOI: 10.1109/TIE.2023.3253940